A projection-onto-convex-sets network for 3D seismic data interpolation
GEOPHYSICS(2023)
摘要
Seismic data interpolation is an essential procedure in seismic data processing. However, conventional interpola-tion methods may generate inaccurate results due to the sim-plicity of assumptions, such as linear events or sparsity. In contrast, deep learning trains a deep neural network with a large data set without relying on predefined assumptions. However, the lack of physical priors in the traditional pure data-driven deep learning frameworks may cause low gen-eralization for different sampling patterns. Inspired by the framework of projection onto convex sets (POCS), a new neural network is proposed for seismic interpolation, called POCS-Net. The forward Fourier transform, the inverse Fou-rier transform, and the threshold parameter in POCS are re-placed by neural networks that are independent in different iterations. The threshold is trainable in POCS-Net rather than manually set. A nonnegative constraint is imposed on the threshold to make it consistent with traditional POCS. POCS-Net is essentially an end-to-end neural network with priors of a sampling pattern and a predefined iterative frame-work. Numerical results on 3D synthetic and field seismic data sets demonstrate the superiority of the reconstruction accuracy of the proposed method compared with the tradi-tional and natural image-learned POCS methods.
更多查看译文
关键词
3d,projection-onto-convex-sets
AI 理解论文
溯源树
样例
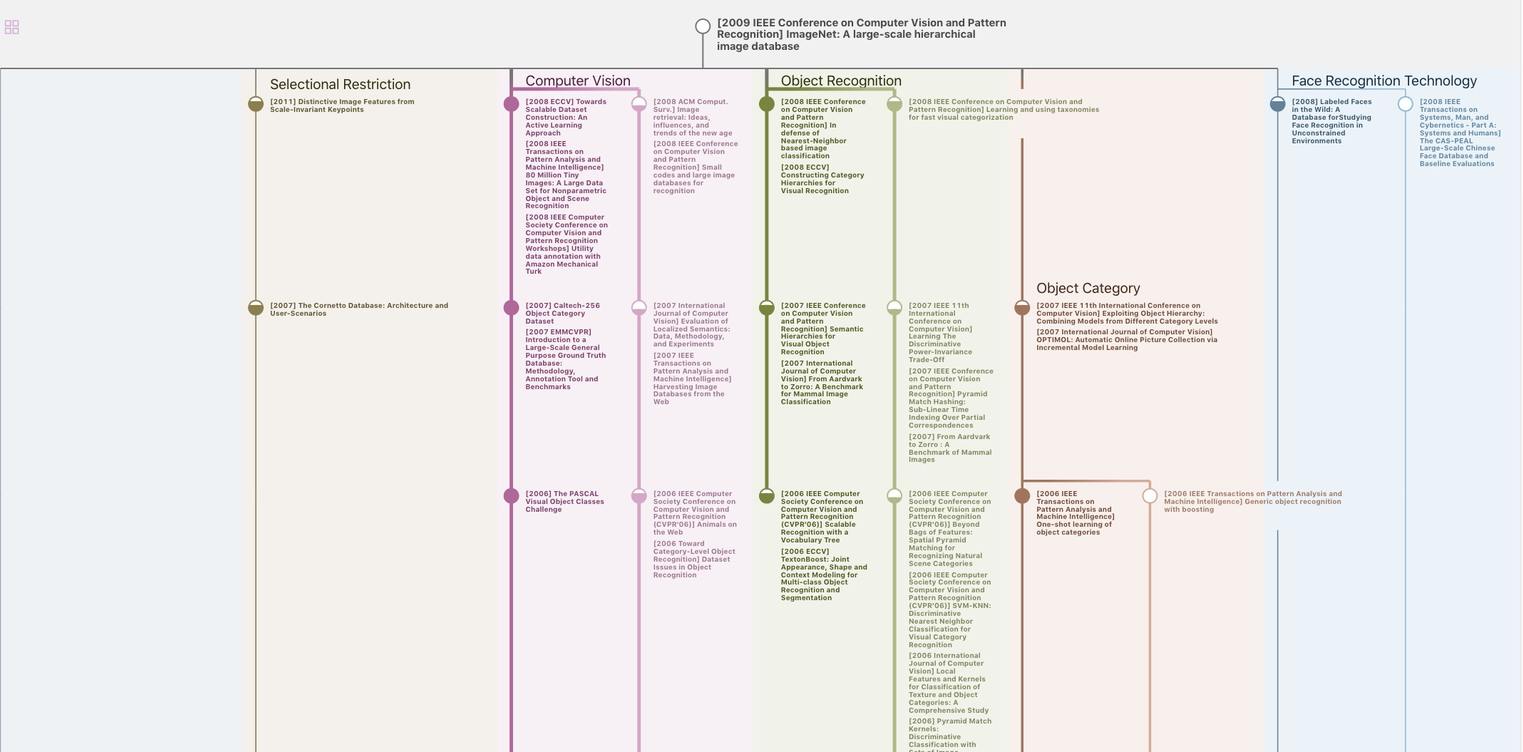
生成溯源树,研究论文发展脉络
Chat Paper
正在生成论文摘要