Lightweight Spatial-Spectral Network Based on 3D-2D Multi-Group Feature Extraction Module for Hyperspectral Image Classification
INTERNATIONAL JOURNAL OF REMOTE SENSING(2023)
摘要
Each pixel in the hyperspectral image contains tens to hundreds of narrow bands of detailed spectral information captured by a hyperspectral sensor. Hyperspectral image classification (HSIC) is widely used in remote sensing image analysis. Convolutional neural network (CNN) is one of the most commonly used deep learning methods based on visual data processing. In recent years, hyperspectral classification method based on the convolutional neural network has shown excellent performance. However, single-scale feature extraction may lose some important detail information and cannot guarantee that the best spatial features are captured. Multi-scale character extraction and increasingly deep network structures can improve classification accuracy, but also bring a large number of computational parameters, resulting in higher computational costs. In response to this series of problems, this paper proposes a lightweight spatial-spectral network based on 3D - 2D multi-group feature extraction module (MGFM) for HSCI. In the 3D-MGFM, the input feature maps are grouped and processed in parallel, and then the information of each channel is integrated by point-wise convolution to achieve spatial-spectral feature fusion. We use dilated convolutions with different dilation rate on the convolution kernel to improve the problem of feature information loss, so that we can better learn deep-level spatial-spectral features. In the 2D-MGFM, we selectively emphasize spectral feature information through SENet and extract multi-scale features based on Depthwise Separable Convolution (DSC) grouping. Experimental results on four hyperspectral datasets demonstrate that the proposed method achieves better classification performance than other state-of-the-art methods.
更多查看译文
关键词
feature extraction,classification,spatial-spectral,multi-group
AI 理解论文
溯源树
样例
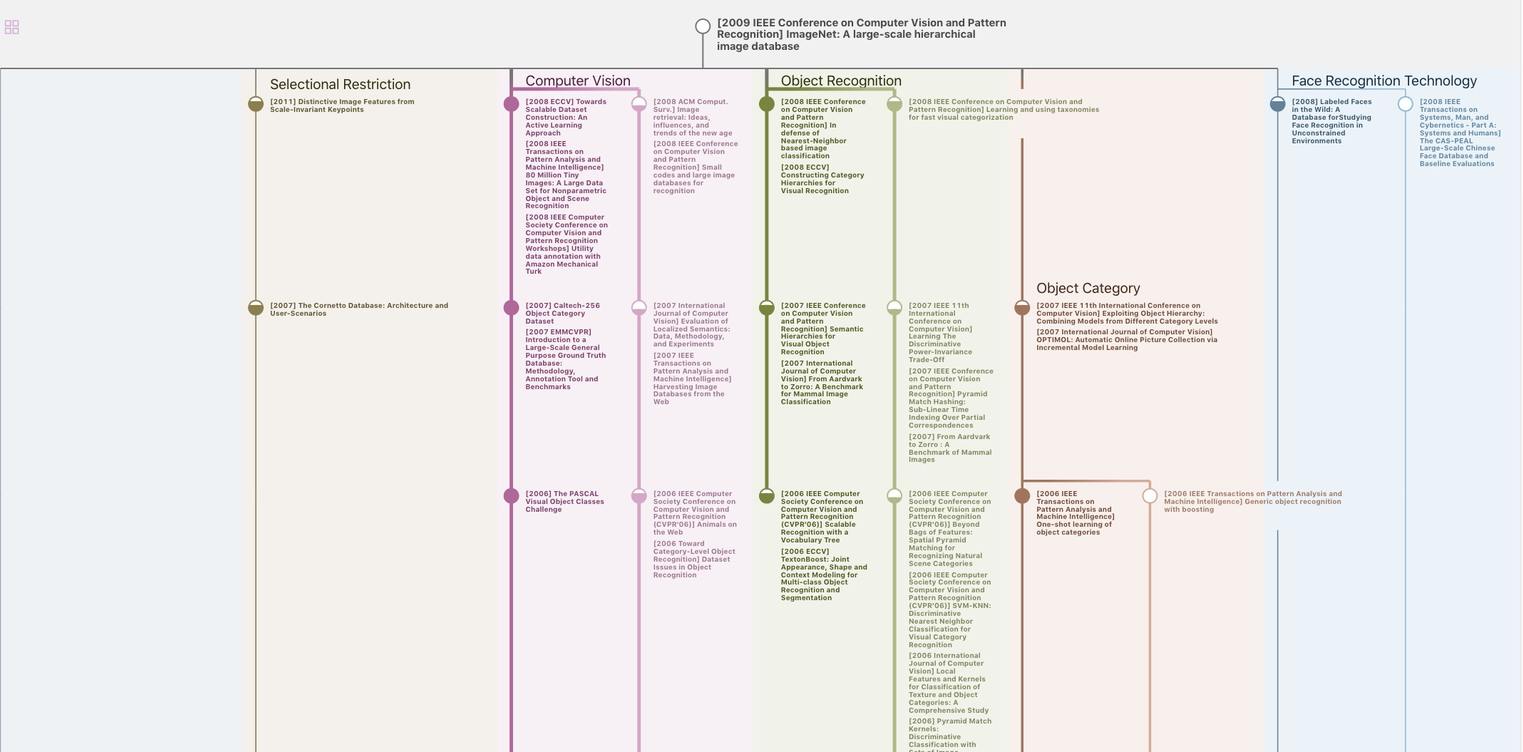
生成溯源树,研究论文发展脉络
Chat Paper
正在生成论文摘要