Deep embeddings and Graph Neural Networks: using context to improve domain-independent predictions
APPLIED INTELLIGENCE(2023)
Abstract
Graph neural networks (GNNs) are deep learning architectures that apply graph convolutions through message-passing processes between nodes, represented as embeddings. GNNs have recently become popular because of their ability to obtain a contextual representation of each node taking into account information from its surroundings. However, existing work has focused on the development of GNN architectures, using basic domain-specific information about the nodes to compute embeddings. Meanwhile, in the closely-related area of knowledge graphs, much effort has been put towards developing deep learning techniques to obtain node embeddings that preserve information about relationships and structure without relying on domain-specific data. The potential application of deep embeddings of knowledge graphs in GNNs remains largely unexplored. In this paper, we carry out a number of experiments to answer open research questions about the impact on GNNs performance when combined with deep embeddings. We test 7 different deep embeddings across several attribute prediction tasks in two state-of-art attribute-rich datasets. We conclude that, while there is a significant performance improvement, its magnitude varies heavily depending on the specific task and deep embedding technique considered.
MoreTranslated text
Key words
deep embeddings,graph neural networks,domain-independent
AI Read Science
Must-Reading Tree
Example
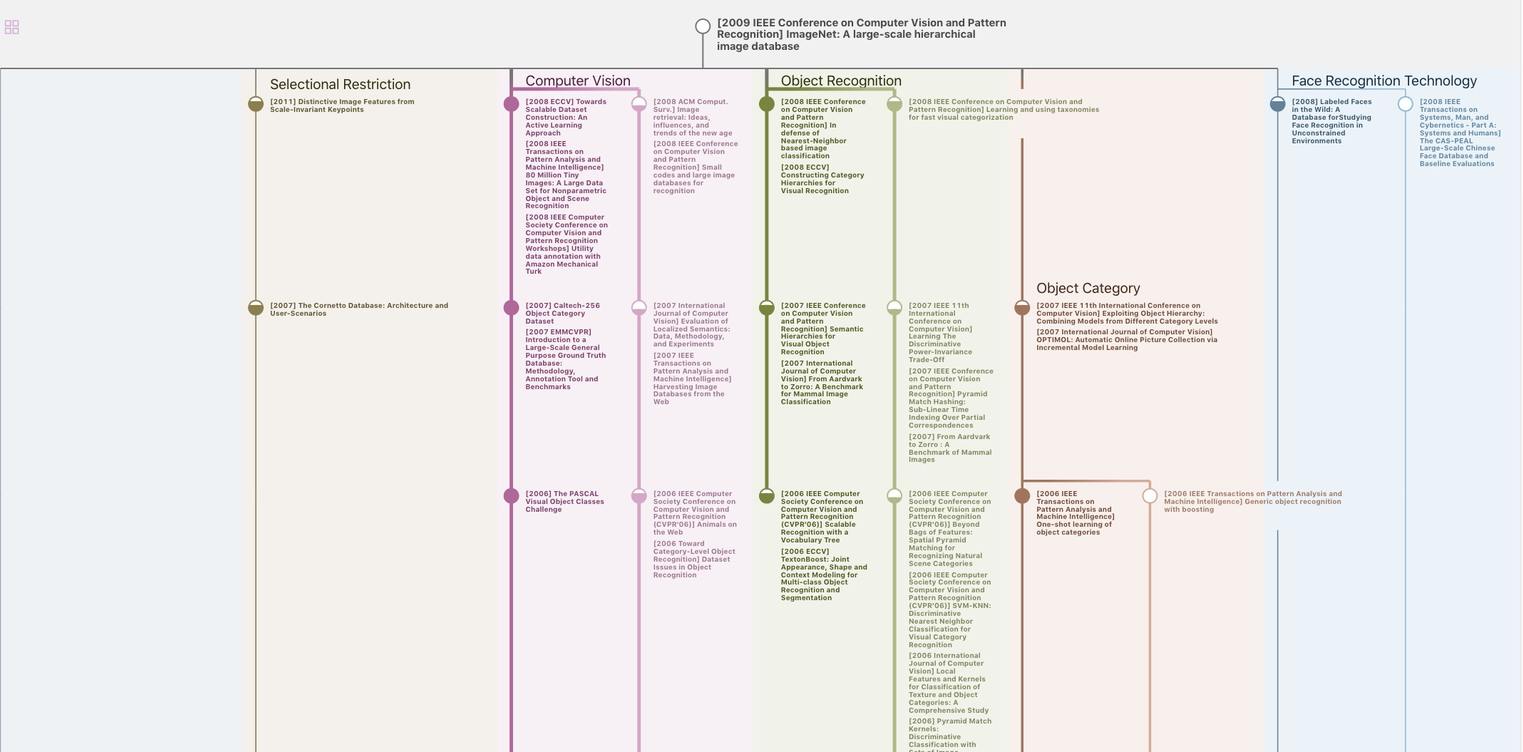
Generate MRT to find the research sequence of this paper
Chat Paper
Summary is being generated by the instructions you defined