Data-driven Ballast Layer Degradation Identification and Maintenance Decision Based on Track Geometry Irregularities
INTERNATIONAL JOURNAL OF RAIL TRANSPORTATION(2024)
Key words
Ballast inspection,data science,maintenance,ballast degradation,track geometry,track irregularity
AI Read Science
Must-Reading Tree
Example
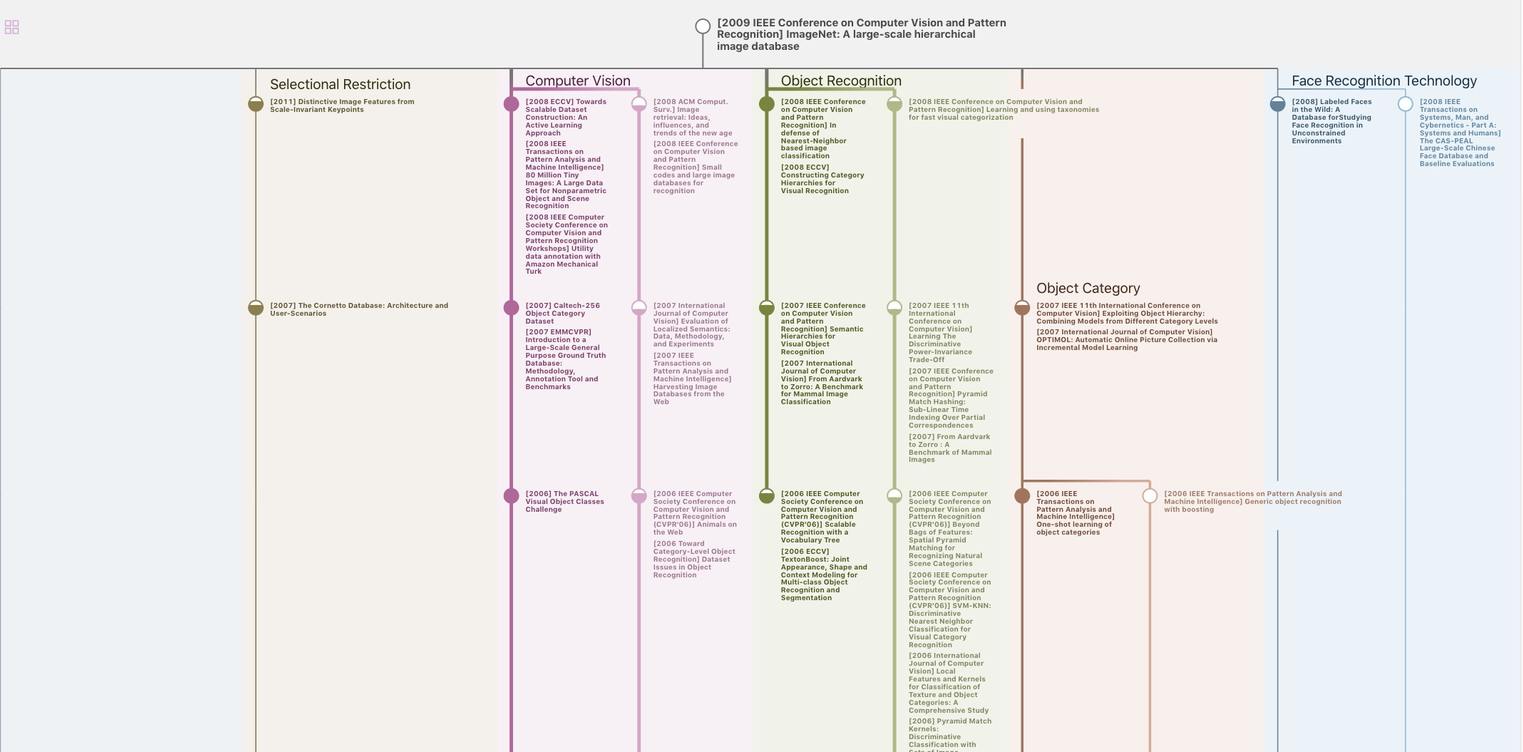
Generate MRT to find the research sequence of this paper
Chat Paper
Summary is being generated by the instructions you defined