Simulating and Comparing CO2/CH4 Separation Performance of Membrane-Zeolite Contactors by Cascade Neural Networks
MEMBRANES(2023)
摘要
Separating carbon dioxide (CO2) from gaseous streams released into the atmosphere is becoming critical due to its greenhouse effect. Membrane technology is one of the promising technologies for CO2 capture. SAPO-34 filler was incorporated in polymeric media to synthesize mixed matrix membrane (MMM) and enhance the CO2 separation performance of this process. Despite relatively extensive experimental studies, there are limited studies that cover the modeling aspects of CO2 capture by MMMs. This research applies a special type of machine learning modeling scenario, namely, cascade neural networks (CNN), to simulate as well as compare the CO2/CH4 selectivity of a wide range of MMMs containing SAPO-34 zeolite. A combination of trial-and-error analysis and statistical accuracy monitoring has been applied to fine-tune the CNN topology. It was found that the CNN with a 4-11-1 topology has the highest accuracy for the modeling of the considered task. The designed CNN model is able to precisely predict the CO2/CH4 selectivity of seven different MMMs in a broad range of filler concentrations, pressures, and temperatures. The model predicts 118 actual measurements of CO2/CH4 selectivity with an outstanding accuracy (i.e., AARD = 2.92%, MSE = 1.55, R = 0.9964).
更多查看译文
关键词
CO2/CH4 gas mixture, membrane separation, selectivity, intelligent modeling
AI 理解论文
溯源树
样例
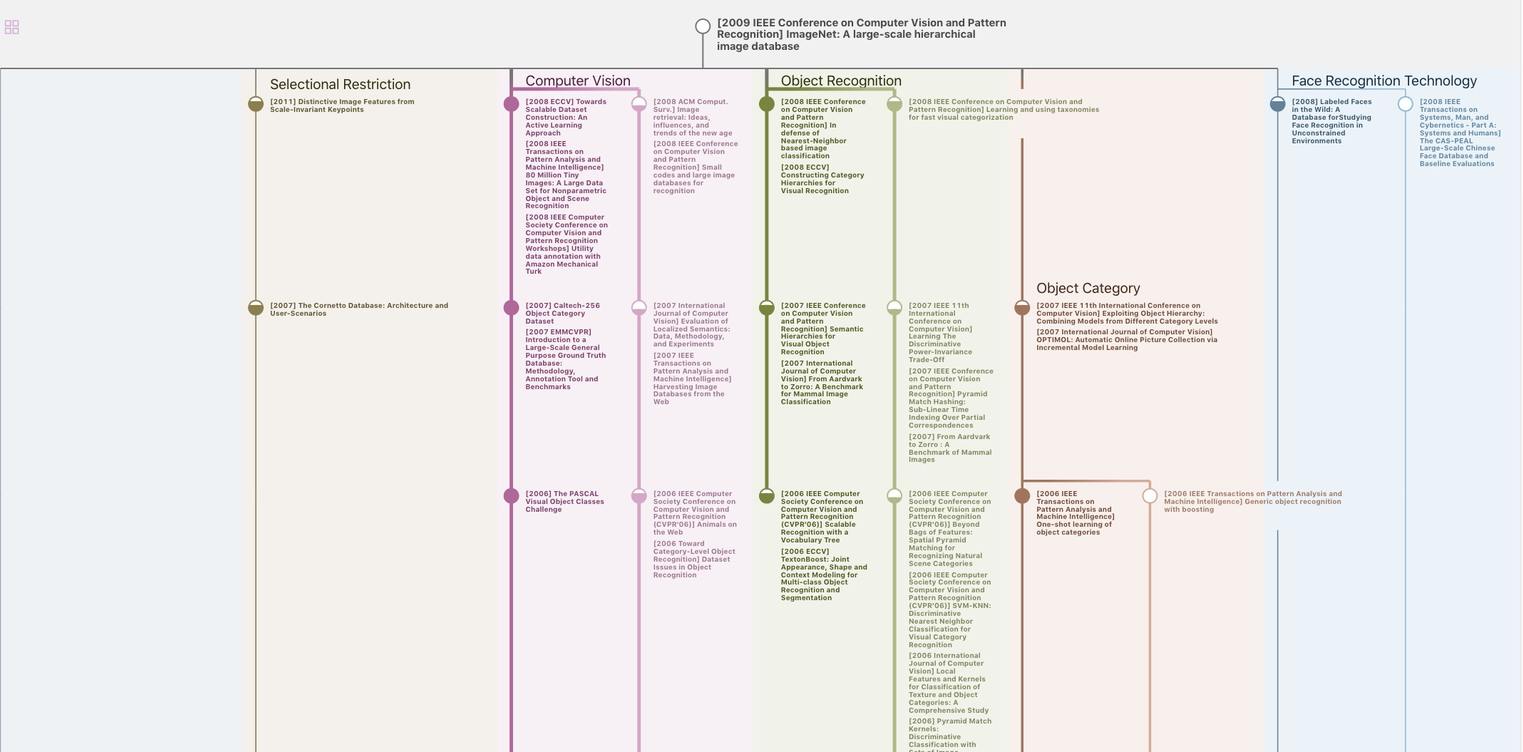
生成溯源树,研究论文发展脉络
Chat Paper
正在生成论文摘要