Performance of representation fusion model for entity and relationship extraction within unstructured text
JOURNAL OF SUPERCOMPUTING(2023)
摘要
Automated identification of entities and their relationships from unstructured text is a critical aspect of information extraction. For this, joint entity and relation extraction is a promising research area aimed at achieving this goal. As a priori knowledge, relation label plays a key role in relation extraction. However, there is a lack of emphasis on incorporating relation labels into the analysis of the unstructured text. This limits the accuracy and effectiveness of entity and relation extraction. Inspired by this motivation, we propose the representation fusion model based on the attention mechanism (RFARE) to help extract relations. We take the vector encoded by the prior relation label as the relation information, which is used to replace the relation embedding matrix with less relation information. To obtain vectors with rich semantic information for relation extraction, we use the attention mechanism to fuse important semantic features between sentences and relation labels selectively. By conducting experiments on two public datasets, NYT and WebNLG, we demonstrate that RFARE effectively leverages relation labels to enrich relation information and improve the semantic interaction between sentences and relationships. Our proposed fusion layer plays an essential role in facilitating this process and improving the overall performance of relation extraction with a simple structure. Specifically, our model achieves F1 score of 92.4% and 92.9% on NYT and WebNLG, respectively, outperforming other baseline models.
更多查看译文
关键词
Joint entity and relation extraction,Relation information,Attention mechanism
AI 理解论文
溯源树
样例
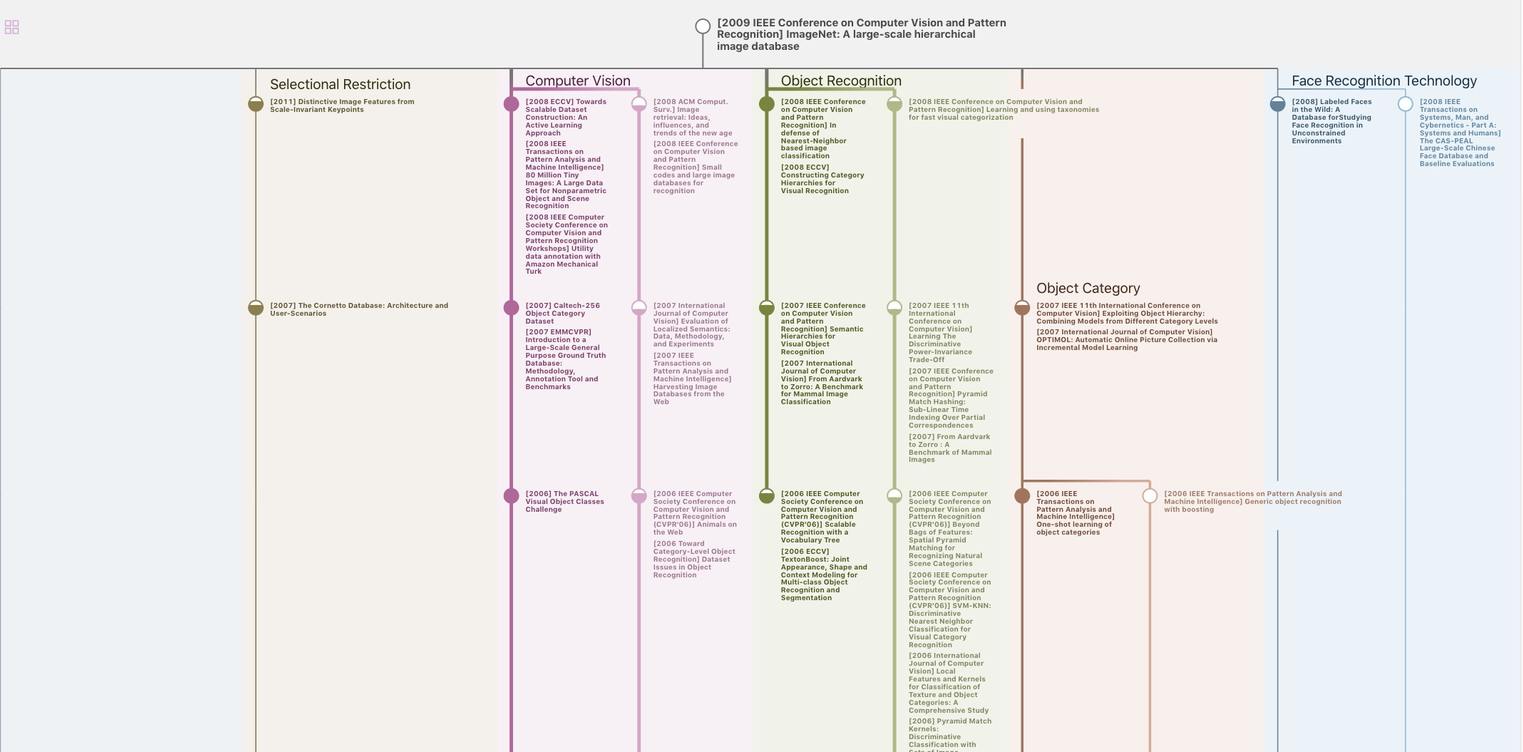
生成溯源树,研究论文发展脉络
Chat Paper
正在生成论文摘要