The Long-Term Theft Prediction in Beijing Using Machine Learning Algorithms: Comparison and Interpretation
CRIME & DELINQUENCY(2023)
摘要
To advance the interpretability of machine learning for long-term crime prediction in China, we compared the performance of multiple machine learning algorithms in predicting the spatial pattern of theft in Beijing. Gradient boosting decision tree emerged as the algorithm with best predictive accuracy. After identifying the importance of criminogenic features, we extended the interpreter SHAP to reveal nonlinear and spatially heterogeneous associations between environmental features and theft and we summarized six relation types of such associations at the global scale. At the local scale, we clustered six area types according to the contribution of environmental attributes to theft prediction in each grid. Policy makers should adopt place-based crime prevention measures based on the specific type of each grid belongs to.
更多查看译文
关键词
crime prediction,gradient boosting decision tree,interpretable machine learning,nonlinear relationship,spatial heterogeneity
AI 理解论文
溯源树
样例
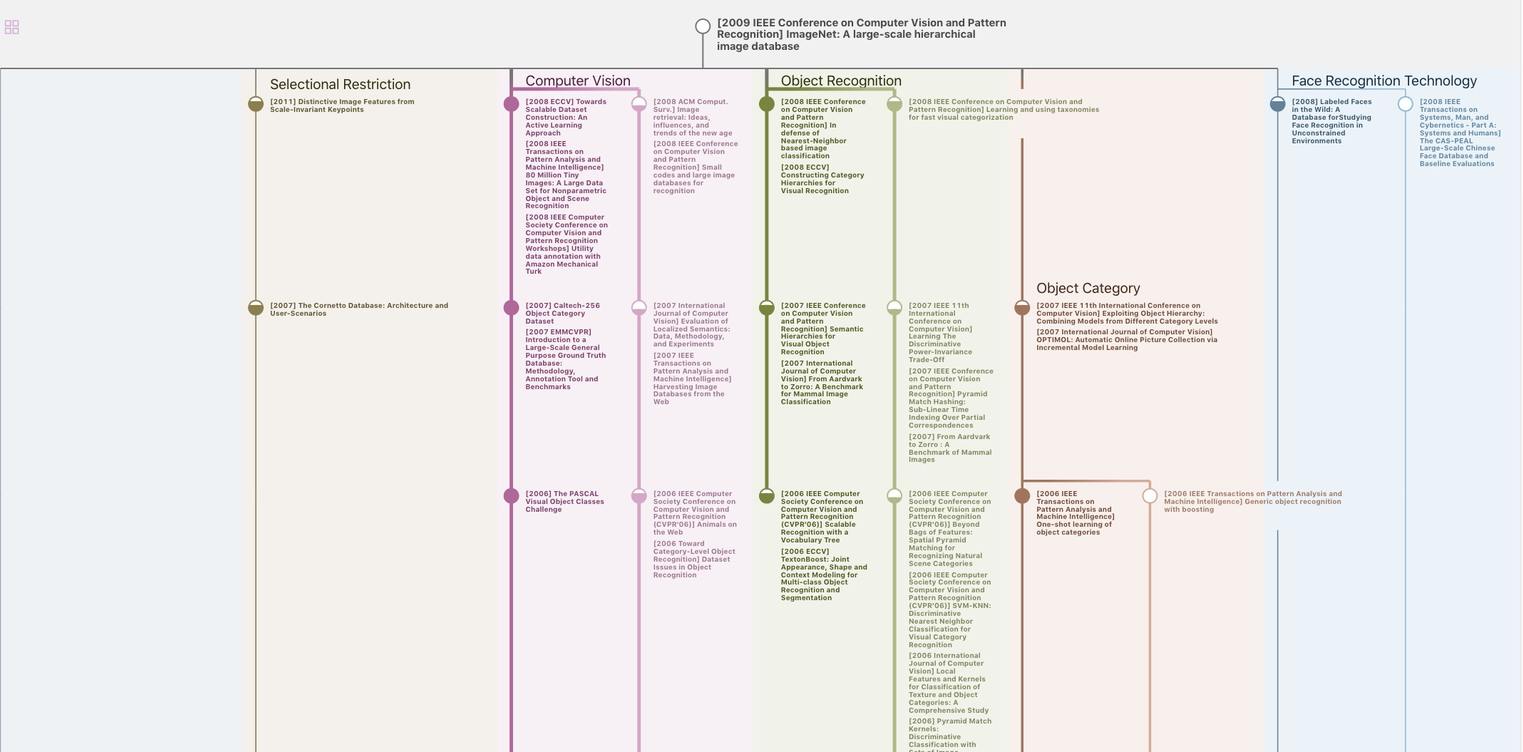
生成溯源树,研究论文发展脉络
Chat Paper
正在生成论文摘要