A novel abnormal data detection method based on dynamic adaptive local outlier factor for the vibration signals of rotating parts
MEASUREMENT SCIENCE AND TECHNOLOGY(2023)
摘要
Abnormal signals are inevitable in big data acquired from harsh industrial environments. Abnormal data detection is a crucial component of condition monitoring for rotating parts and is also the premise of data cleaning, compensation, and mining. To detect abnormal data segments of rolling bearings, this paper proposes a dynamic adaptive local outlier factor (DALOF) anomaly detection method. First, a data dynamic segmentation method based on sliding windows is designed to determine samples with variable lengths. Then, a time-domain feature extraction and fusion method based on principal component analysis is exploited to reduce the feature space discrepancy. To improve the accuracy of abnormal data detection, a data quality evaluation model is established to assess each data segment using DALOF. The validity of the proposed method is also verified by analyzing signals including missing data, random interference data, and drift data. Several other methods are respectively applied to identify these abnormal data to further demonstrate the benefits of the developed methodology.
更多查看译文
关键词
vibration signals
AI 理解论文
溯源树
样例
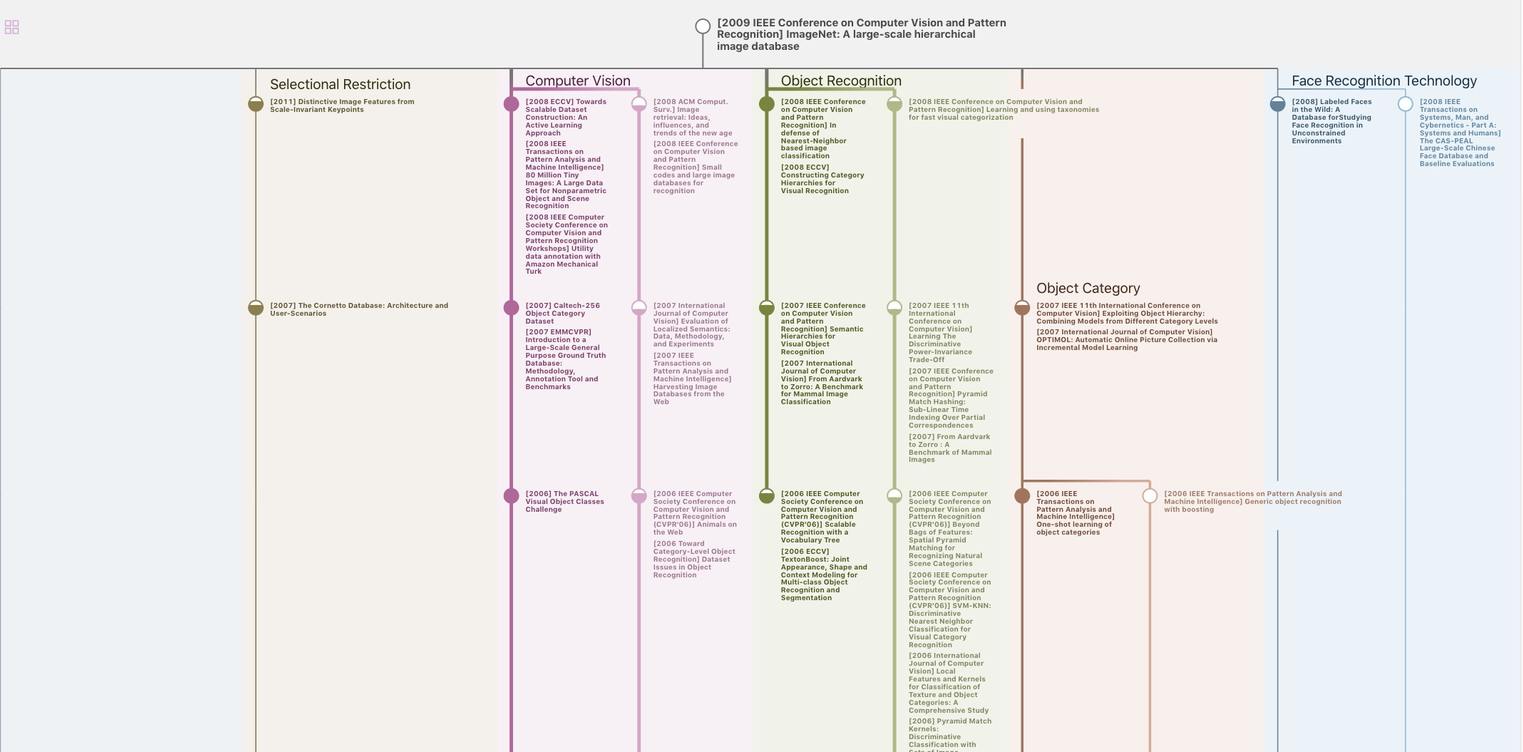
生成溯源树,研究论文发展脉络
Chat Paper
正在生成论文摘要