Optimizing Icon Sizes on In-Vehicle Panels to Minimize Perception Time and Prevent Driving Crashes
INTERNATIONAL JOURNAL OF HUMAN-COMPUTER INTERACTION(2023)
摘要
Distracted driving increases the risk of driving errors and accidents, and it has become the main factor leading to accidents. Currently, a large amount of information is displayed on in-vehicle panels, which possibly distract drivers' attention, diverting their gaze from the road. To minimize the negative impact of distracted driving, this study sought to optimize the design of icons presented on vehicle screens. We investigated the effect of icon size, icon position, driving state, and age on drivers' perception time, driving performance, and subjective evaluations. A total of 42 drivers (26 men and 20 women) between the ages of 22 and 70 years participated in the study, completing a simulated driving task and a secondary visual task. The results demonstrated significant main effects of icon size, driving state, icon position, and interaction effects. Participants spent a longer time perceiving smaller icons on the center control panel in the driving state. As the icon size increases, perception time decreases at a reduced speed. During the static state, perception time leveled out from 44 & PRIME; while during the driving state, perception time leveled out from 80 & PRIME;. On the instrument panel, perception time leveled out from 60 & PRIME; while on the center control panel, perception time leveled out from 80 & PRIME;. Subjective evaluations supported behavioral data on the effect of icon size. Our results indicated the importance of strategic design for icons on vehicle screens, which could reduce the visual workload, then reduce the risk of traffic accidents. According to the results, enterprises or designers could target the design of icon size according to different types of in-vehicle panels or screens.
更多查看译文
关键词
Distracted driving, secondary task, icons, in-vehicle panels, perception time
AI 理解论文
溯源树
样例
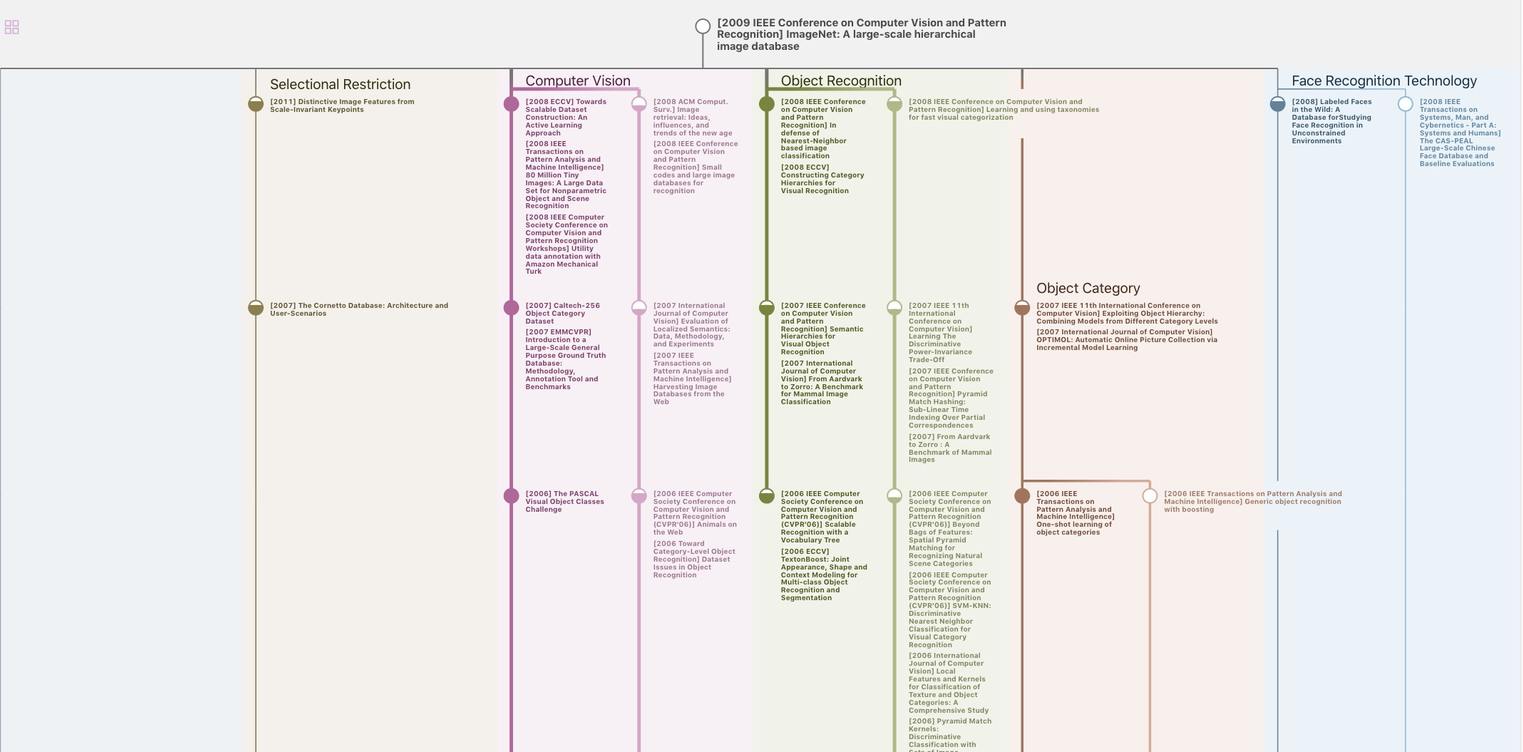
生成溯源树,研究论文发展脉络
Chat Paper
正在生成论文摘要