The Impact of Adversarial Attacks on Interpretable Semantic Segmentation in Cyber–Physical Systems
IEEE SYSTEMS JOURNAL(2023)
摘要
The widespread adoption of deep learning (DL) models raises concerns about their trustworthiness and reliability. Adversarial attacks are cyber-related attacks that target the DL network's prediction by adding imperceptible perturbations to its input. Their deployment against critical artificial-intelligence-based systems, such as industrial cyber–physical systems (ICPSs), can result in substantial damage. Research on their scope and limitations can provide information that would help with their detection and prevention. In this article, the interconnection of adversarial attacks and interpretable semantic segmentation is investigated for potential applications in the ICPS in order to contribute to the safe use of future intelligent systems. We first explore gradient-based interpretability extensions to semantic segmentation on two industry-related cyber–physical system datasets. Then, two types of attacks on semantic segmentation networks are discussed. First, we apply the dense adversary generation attack on different segmentation outputs and evaluate its influence on the corresponding saliency maps. We then introduce a way to visualize the similarity of attacked saliency maps to the original with respect to the targeted attack's direction. Finally, we extend the application of adversarial attacks on saliency maps to semantic segmentation.
更多查看译文
关键词
interpretable semantic segmentation,adversarial attacks,cyber–physical
AI 理解论文
溯源树
样例
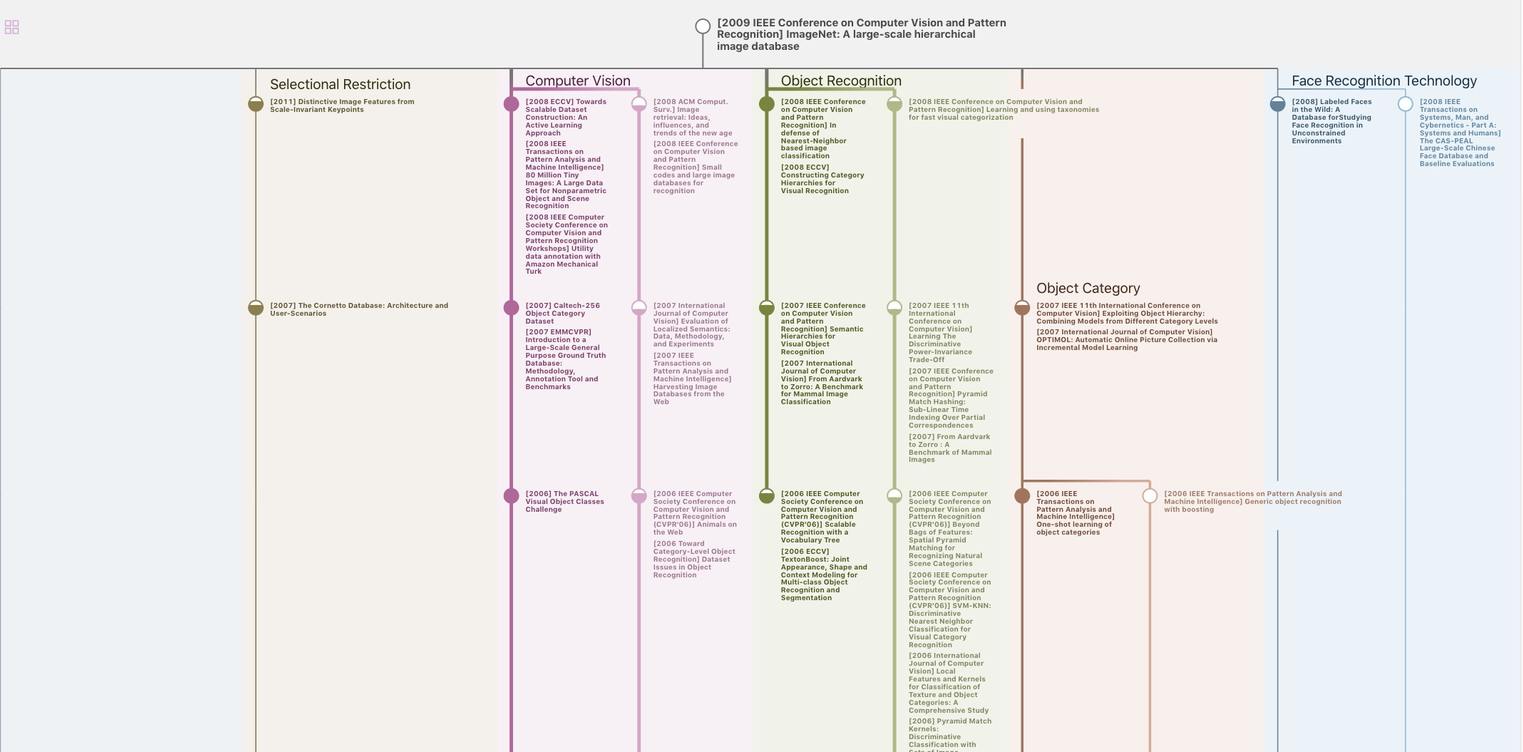
生成溯源树,研究论文发展脉络
Chat Paper
正在生成论文摘要