Machine Learning Based Prediction of Enzymatic Degradation of Plastics Using Encoded Protein Sequence and Effective Feature Representation
ENVIRONMENTAL SCIENCE & TECHNOLOGY LETTERS(2023)
摘要
Enzyme biocatalysis for plastic treatment and recyclingis an emergingfield of growing interest. However, it is challenging and time-consumingto identify plastic-degrading enzymes with desirable functionality,given the large number of putative enzyme sequences. There is a criticalneed to develop an effective approach to accurately predict the enzymeactivity in degrading different types of plastics. In this study,we developed a machine-learning-based plastic enzymatic degradation(PED) framework to predict the ability of an enzyme to degrade plasticsof interest by exploring and recognizing hidden patterns in proteinsequences. A data set integrating information from a wide range ofexperimentally verified enzymes and various common plastic substrateswas created. A new context-aware enzyme sequence representation (CESR)mechanism was developed to learn the abundant contextual informationin enzyme sequences, and feature extraction was performed for enzymesat both the amino acid level and global sequence level. Thirteen machinelearning classification algorithms were compared, and XGBoost wasidentified as the best-performing algorithm. PED achieved an overallaccuracy of 90.2% and outperformed sequence-based protein classificationmodels from the existing literature. Furthermore, important enzymefeatures in plastic degradation were identified and comprehensivelyinterpreted. This study demonstrated a new tool for the predictionand discovery of plastic-degrading enzymes.
更多查看译文
关键词
enzymatic degradation,machine learning,protein sequence,plastics
AI 理解论文
溯源树
样例
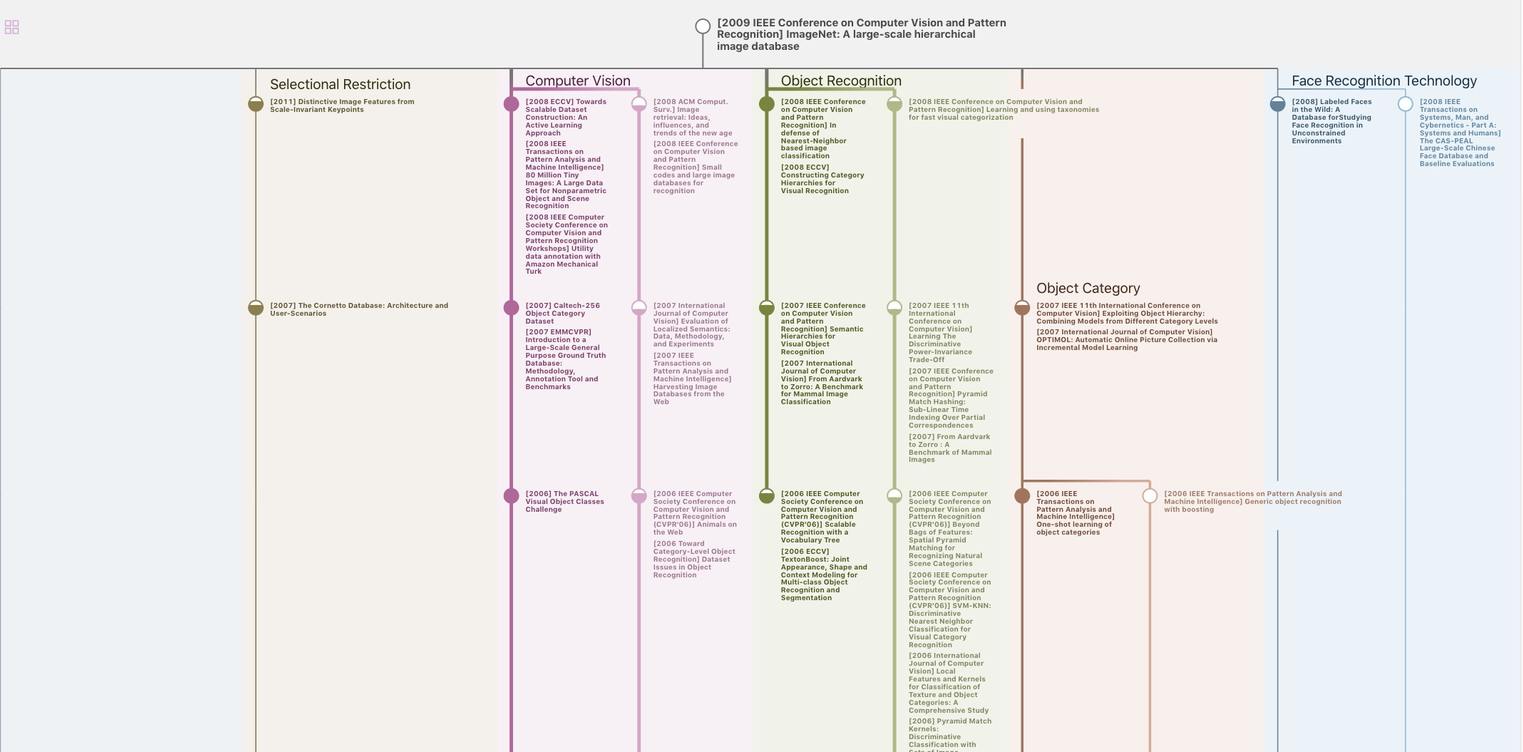
生成溯源树,研究论文发展脉络
Chat Paper
正在生成论文摘要