Experimental Evaluation of Insider Threat Detection Methods Based on Temporal Representation
2022 IEEE 10TH INTERNATIONAL CONFERENCE ON INFORMATION, COMMUNICATION AND NETWORKS (ICICN 2022)(2022)
摘要
At present, insider threat detection faces many challenges, such as imbalanced data sets, the complexity of malicious behavior, high data dimension, and difficulty for manual analysis to keep up with the data growth rate. An experimental evaluation of insider threat detection methods is carried in this paper. Firstly, the open data set is divided by day and week. Then, various temporal representations including percentile, mean difference, median difference, and concatenation of instances representations, are used to extract potential information. Four unsupervised machine learning algorithms and a supervised algorithm are comprehensively compared to explore the best detection schemes under different temporal representations. To comprehensively evaluate the detection performance of the algorithms, four commonly used performance metrics (i.e. Detection rate (DR), Precision (PR), F1-score (F1), Area Under the Curve (AUC)) are introduced. The experimental results show that the random forest algorithm can achieve the best detection results using day granularity data combined with median differential representation-meddiff30, and the DR, PR, F1, and AUC are 75.43%, 99.59%, 85.84%, and 87.71% respectively. For the four unsupervised detection algorithms, under the 20% investigation budget, the autoencoder combined with percentile representation-percentile30 in day granularity achieved DR of 95.83%, and AUC of 94.93%, with higher PR and F1 values under the same conditions. The local outlier factor algorithm combined with concatenation representation-c3 under week granularity achieved the highest DR, but the scheme of the autoencoder combined with percentile30 still achieved the highest PR, F1, and AUC.
更多查看译文
关键词
insider threats,unsupervised algorithm,supervised algorithm,temporal representation,performance metrics
AI 理解论文
溯源树
样例
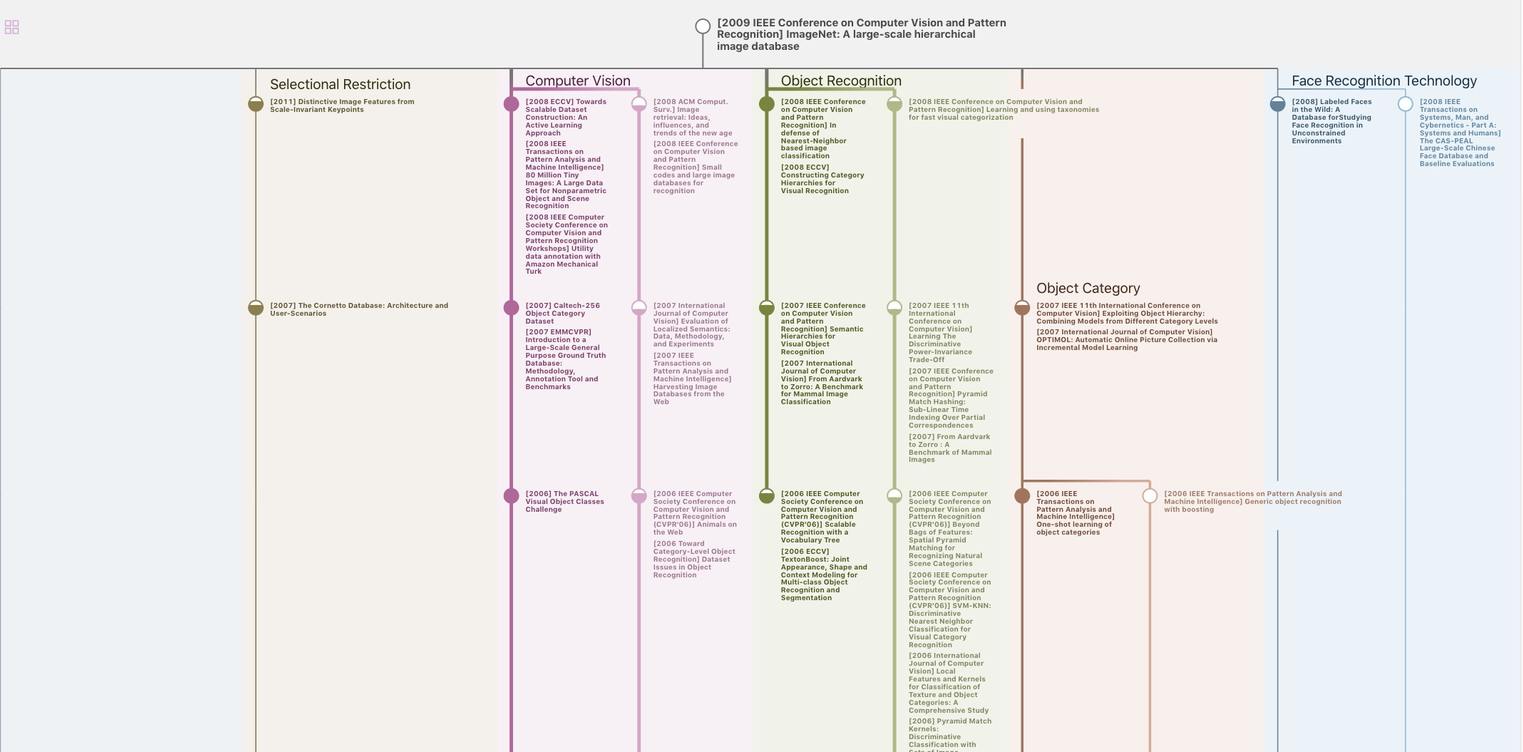
生成溯源树,研究论文发展脉络
Chat Paper
正在生成论文摘要