Wind speed forecasting using deep learning and preprocessing techniques
INTERNATIONAL JOURNAL OF GREEN ENERGY(2024)
摘要
Most forecasting algorithms are tuned to a specific location or dataset and will not perform well in other situations. Some wind speed data might contain outliers, missed values, or noise which affects the forecasting performance tremendously. This paper proposes a hybrid-forecasting model that includes pre-processing and deep learning techniques to bridge the gap in developing a generic forecasting algorithm that is unspecific of location or dataset. The proposed model includes a preprocessing part that consists of missed value and outlier handling, decomposition, Kalman filtering, and smoothing. This is an important step because no matter how accurate the forecasting model is, results will vary tremendously if the dataset is corrupted. In addition to that, three different deep-base learning algorithms RNN, GRU, and LSTM will be used based on the characteristics of each subseries to reduce the complexity of the overall forecasting model. The proposed model performed the best across the seven tested sites from different locations with different climates and geography. Compared to other forecasting models such as LSTM standalone and EWT-LSTM, a performance improvement in accuracy by 50% as well as a 25% reduction in processing time was achieved with the proposed forecasting model
更多查看译文
关键词
Artificial neural intelligence,wind speed forecasting,hybrid forecasting,data preprocessing,outlier detection,Kalman smoothing,deep neural network
AI 理解论文
溯源树
样例
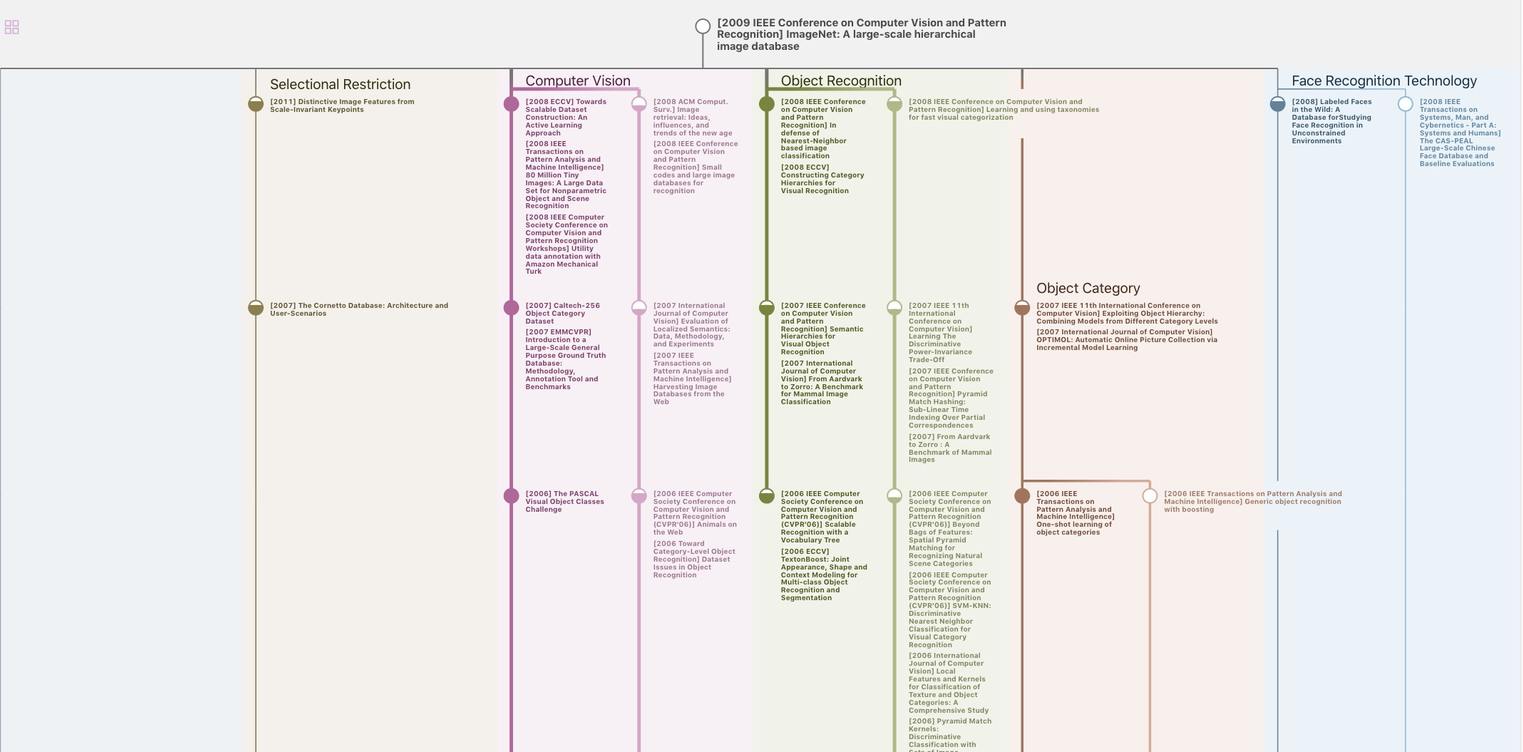
生成溯源树,研究论文发展脉络
Chat Paper
正在生成论文摘要