Determination of material parameters in constitutive models using adaptive neural network machine learning
JOURNAL OF THE MECHANICS AND PHYSICS OF SOLIDS(2023)
摘要
A challenge in the constitutive modeling of time dependent, non-linear solids is the identifica-tion of potentially large numbers of material parameters. Here we present an efficient method to determine these parameters using a machine learning algorithm based on Singular Value Decomposition (SVD) and an adaptive neural network (NN). SVD compresses training data and provides outputs for the NN. The trained network rapidly computes the material responses for a large set of material parameters. These responses are then compared with experimental data to determine the optimal parameters. We test our algorithm by performing uniaxial cyclic and relaxation tests on three hydrogels with very different time dependent behaviors: a chemically and physically crosslinked polyampholyte (c-PA) gel; a pure physically crosslinked PA (p-PA) gel, and a Poly(vinylalcohol) (PVA) hydrogel with both chemical and physical crosslinks.
更多查看译文
关键词
material parameters,constitutive models,neural network,machine learning
AI 理解论文
溯源树
样例
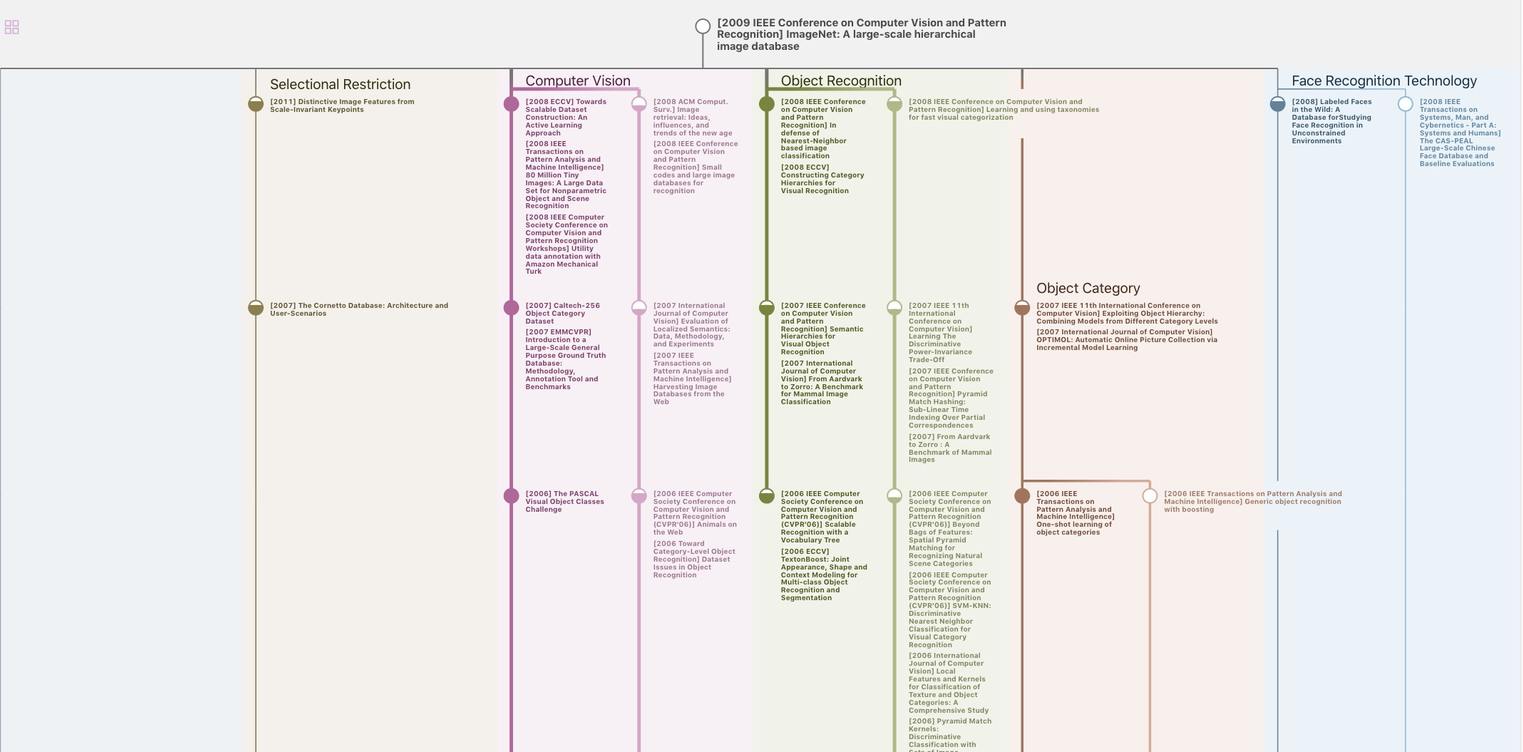
生成溯源树,研究论文发展脉络
Chat Paper
正在生成论文摘要