Quantitative Analysis of Steel Alloy Elements Based on LIBS and Deep Learning of Multi-Perspective Features
ELECTRONICS(2023)
摘要
The Si and Mn contents in steel alloys are important characteristic indexes that influence the plasticity and welding properties of these alloys. In this work, the quantitative analysis methods for trace elements under complex alloy matrices by laser-induced breakdown spectroscopy (LIBS) are studied, which provide a foundation for utilizing LIBS technology in the rapid online detection of steel alloy properties. To improve the quantitative analysis accuracy of LIBS, deep learning algorithm methods are introduced. Given the characteristics of LIBS spectra, we explore multi-perspective feature extraction and backward differential methods to extract the spatio-temporal characteristics of LIBS spectra. The Text Convolutional Neural Network (TextCNN) model, combined with multi-perspective feature extraction, displays good stability and lower average relative errors (6.988% for Si, 6.280% for Mn) in the test set compared to the traditional quantitative analysis method and deep neural network (DNN) model. Finally, the backward differential method is employed to optimize the two-dimensional LIBS spectral input matrix, and the results indicate that the average relative errors of Si and Mn elements in the test set decrease to 5.139% and 3.939%, respectively. The method proposed in this work establishes a theoretical basis and technical support for precise prediction and online quality monitoring.
更多查看译文
关键词
steel alloy elements,deep learning,features,multi-perspective
AI 理解论文
溯源树
样例
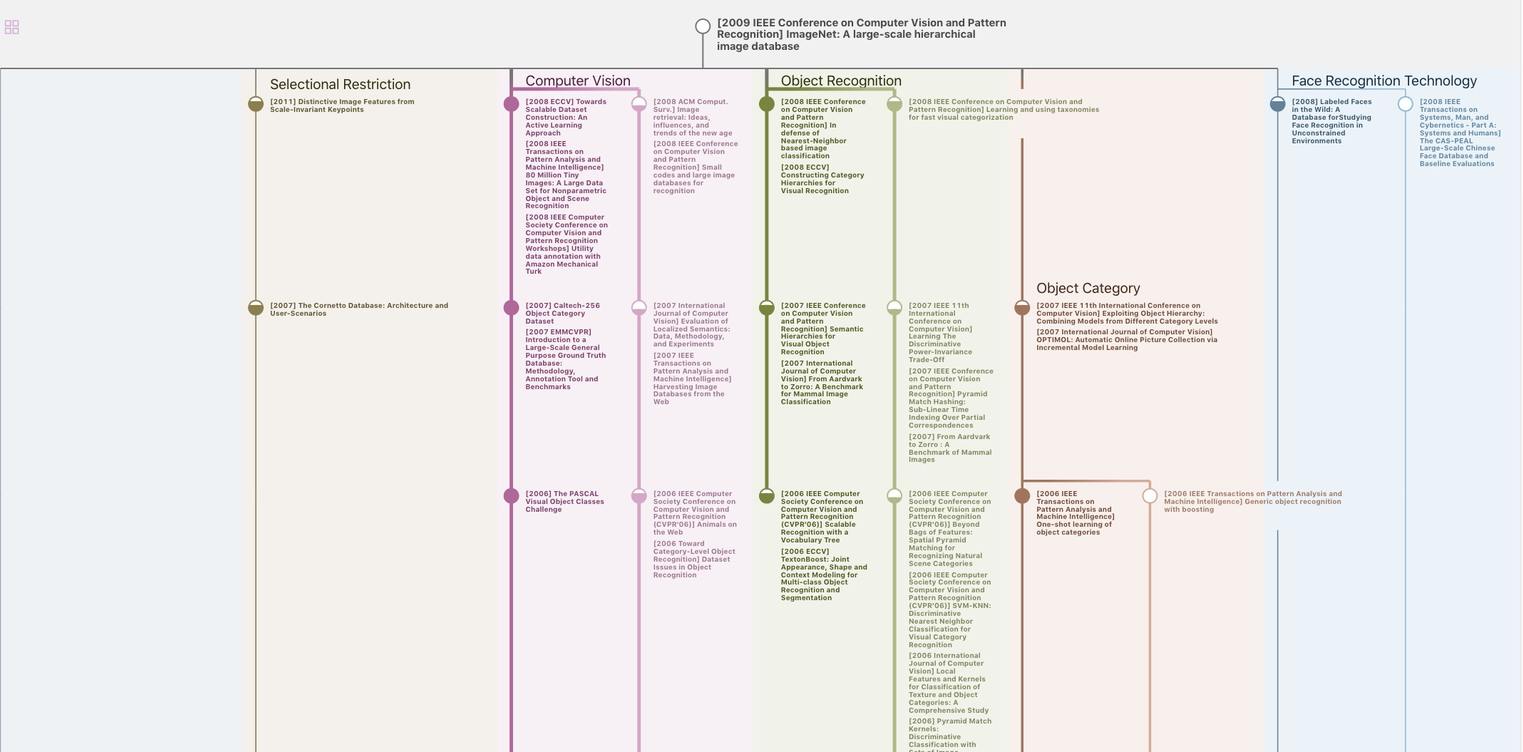
生成溯源树,研究论文发展脉络
Chat Paper
正在生成论文摘要