Real-time hand gesture recognition using multiple deep learning architectures
Signal Image Video Process.(2023)
Abstract
Human gesture recognition is one of the most challenging problems in computer vision, striving to analyze human gestures by machine. However, most of the literature on gesture recognition utilizes isolated data with only one gesture in one image or a video for classifying gestures. This work targets the identification of human gestures from the continuous stream of data input taken from a live camera feed, with no pre-defined boundaries. This task becomes even more complex given the diverse lighting conditions, varying backgrounds and different gesture positions in the same input stream of data. This work presents an effective deep learning architecture to classify gestures taken from multiple viewpoints and varying object sizes. To perform the classification, in this work, we have synthesized a real-world dataset consisting of 4500 images collected from different persons of varying age groups ranging from 10 to 50. The dataset is accumulated considering a wide variety of characteristics to address the complexities in the gesture recognition process. A real-time system is developed that captures, analyzes and classifies live gesture videos frame by frame. To prove the validity of our approach, we have compared our results with multiple deep learning architectures and other benchmark datasets. The results depict that our approach outperforms the existing works and is able to detect gestures with deteriorating lighting conditions and murky gesture positions, achieving an accuracy of 99.63%.
MoreTranslated text
Key words
Convolution neural network,Artificial neural network,Gesture recognition,Transfer learning
AI Read Science
Must-Reading Tree
Example
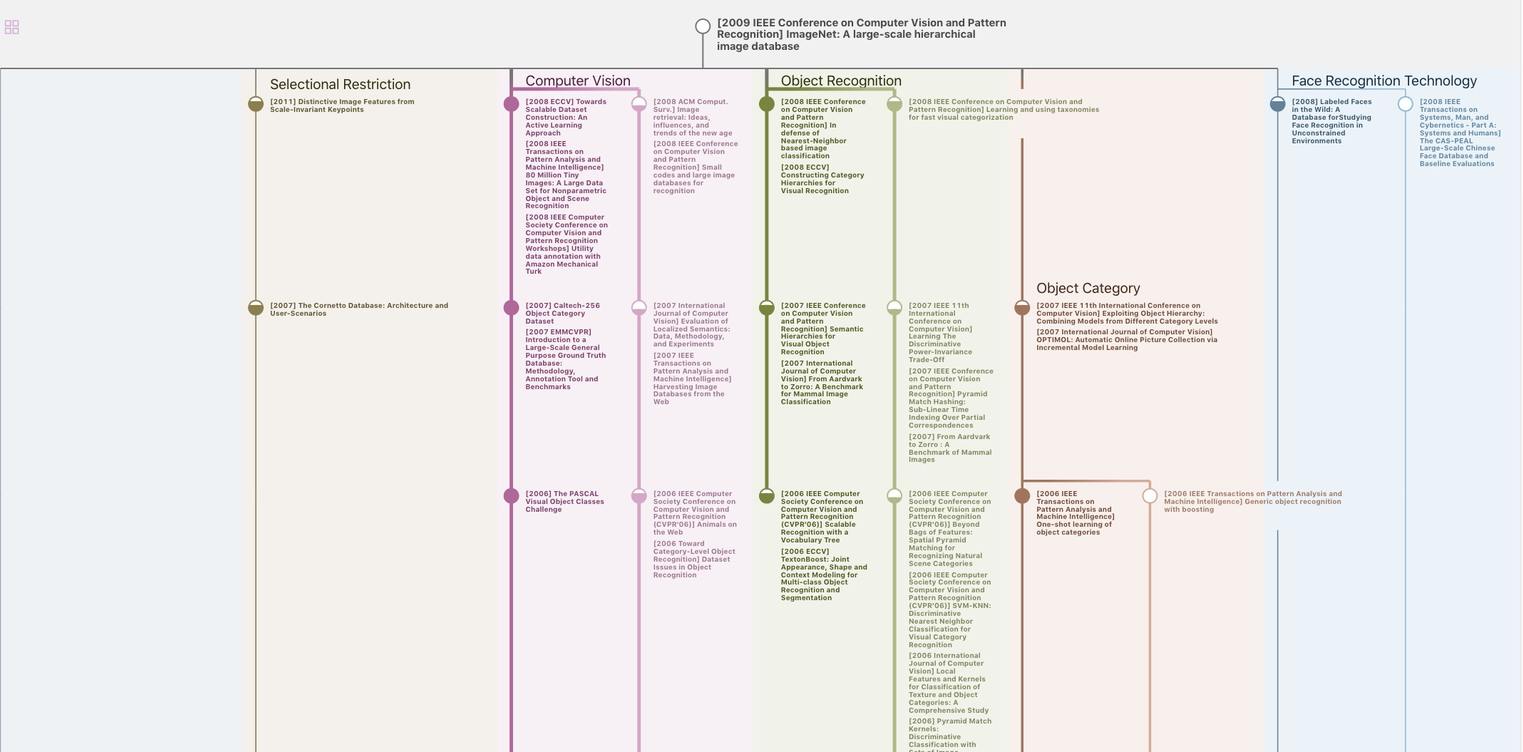
Generate MRT to find the research sequence of this paper
Chat Paper
Summary is being generated by the instructions you defined