Data-Driven-Based Terahertz Image Restoration
IEEE SENSORS JOURNAL(2023)
摘要
Terahertz (THz) has been widely used in radar, remote sensing, atmospheric and environmental monitoring, real-time biological information extraction, and medical diagnosis. However, the long-wavelength property of THz leads to low imaging resolution. Due to the expensive nature of experimental equipment and the difficulty of obtaining THz sources, most scientific institutions have difficulty in obtaining large amounts of the THz dataset. In addition, it is difficult for convolution neural networks (CNNs) to recover low-resolution complex images because of the phase information contained in the images. In this article, we build a THz imaging system and propose a progressive multiscale augmented neural network (PMANN) to address this problem. PMANN integrates self-attention and channel attention for image contextual feature extraction by the proposed progressive network. Compared with the complex CNN (CCNN) in private cartoon datasets, PMANN improves the quantitative metrics on peak signal-to-noise ratio (PSNR) by about 18.6%. It also obtains better visual quality than CCNN. Moreover, we publicly synthesize the first grayscale image MinistTHz dataset. Compared with SwinTransformer, PMANN improves the PSNR by 28.2%. The experimental results on the THz dataset demonstrate that the proposed model achieves better performances than the state-of-the-art (SOTA) models. Our synthetic THz dataset is open at https://github.com/szpxmu/PMANN.
更多查看译文
关键词
data-driven-based
AI 理解论文
溯源树
样例
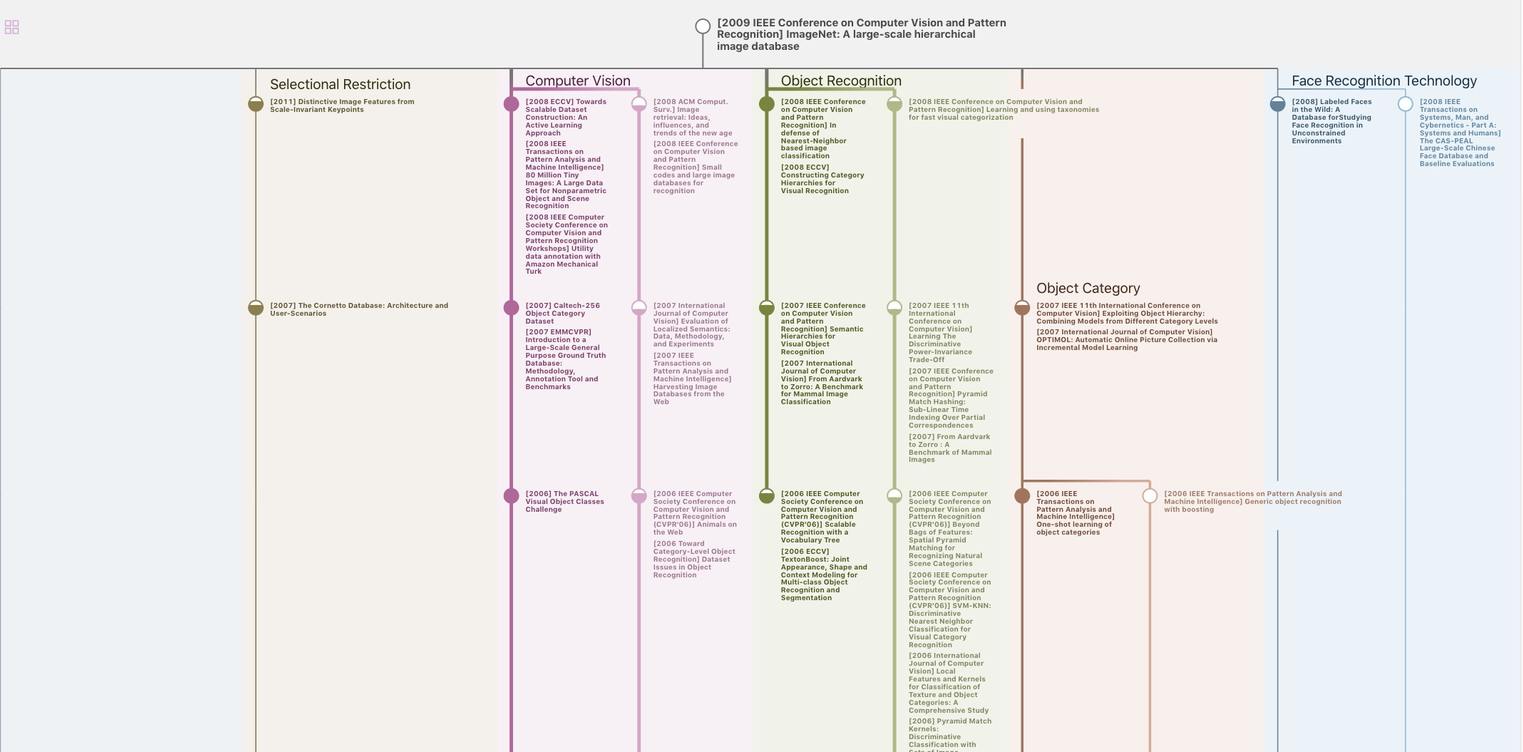
生成溯源树,研究论文发展脉络
Chat Paper
正在生成论文摘要