Deeply Activated Salient Region for Instance Search
ACM TRANSACTIONS ON MULTIMEDIA COMPUTING COMMUNICATIONS AND APPLICATIONS(2022)
摘要
The performance of instance search relies heavily on the ability to locate and describe a wide variety of object instances in a video/image collection. Due to the lack of a proper mechanism for locating instances and deriving feature representation, instance search is generally only effective when the instances are from known object categories. In this article, a simple but effective instance-level feature representation approach is presented. Different from the existing approaches, the issues of class-agnostic instance localization and distinctive feature representation are considered. The former is achieved by detecting salient instance regions from an image by a layer-wise back-propagation process. The back-propagation starts from the last convolution layer of a pre-trained CNNs that is originally used for classification. The back-propagation proceeds layer by layer until it reaches the input layer. This allows the salient instance regions in the input image from both known and unknown categories to be activated. Each activated salient region covers the full or, more usually, a major range of an instance. The distinctive feature representation is produced by average-pooling on the feature map of a certain layer with the detected instance region. Experiments show that this kind of feature representation demonstrates considerably better performance than most of the existing approaches.
更多查看译文
关键词
activated salient region,search
AI 理解论文
溯源树
样例
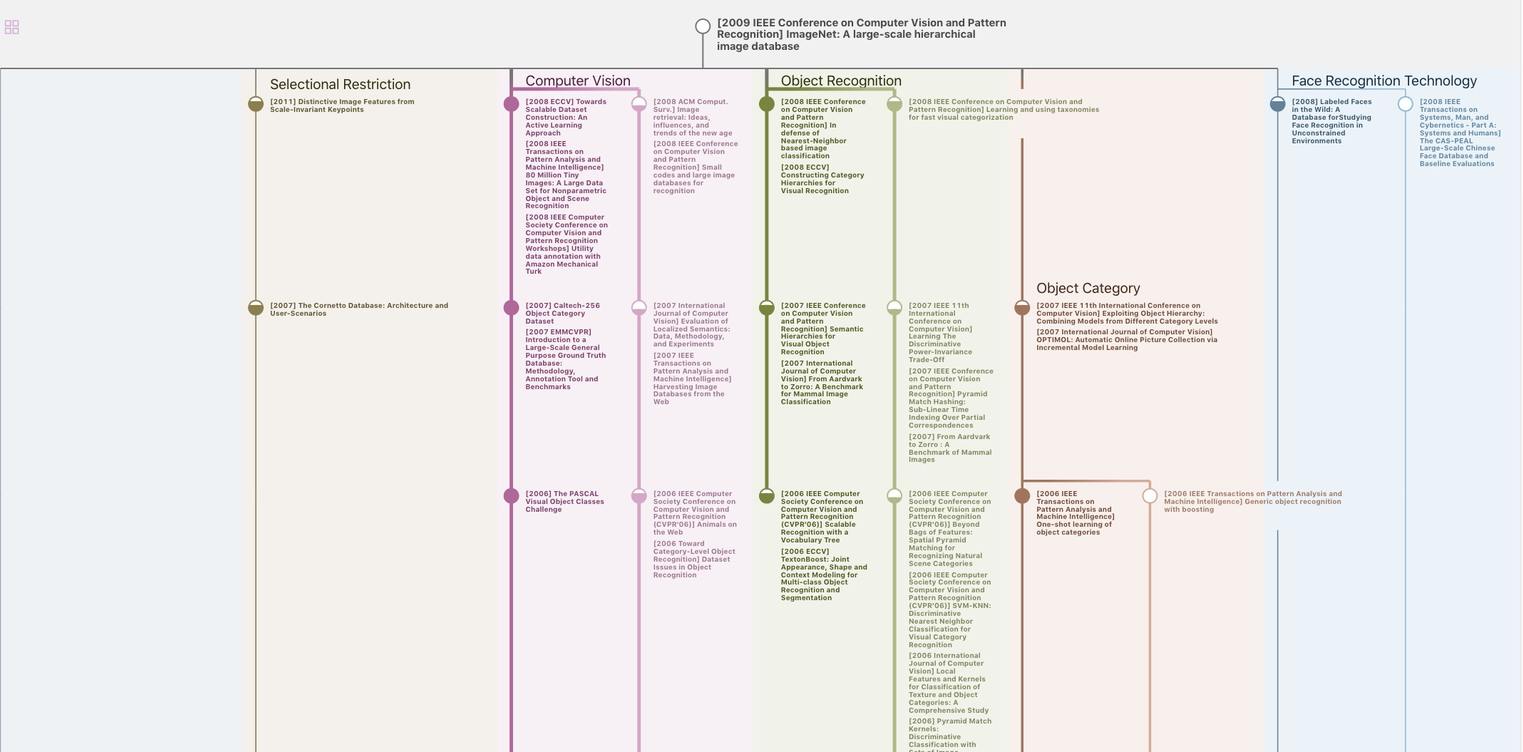
生成溯源树,研究论文发展脉络
Chat Paper
正在生成论文摘要