SLPT: Selective Labeling Meets Prompt Tuning on Label-Limited Lesion Segmentation
MEDICAL IMAGE COMPUTING AND COMPUTER ASSISTED INTERVENTION, MICCAI 2023, PT II(2023)
Abstract
Medical image analysis using deep learning is often challenged by limited labeled data and high annotation costs. Fine-tuning the entire network in label-limited scenarios can lead to overfitting and suboptimal performance. Recently, prompt tuning has emerged as a more promising technique that introduces a few additional tunable parameters as prompts to a task-agnostic pre-trained model, and updates only these parameters using supervision from limited labeled data while keeping the pre-trained model unchanged. However, previous work has overlooked the importance of selective labeling in downstream tasks, which aims to select the most valuable downstream samples for annotation to achieve the best performance with minimum annotation cost. To address this, we propose a framework that combines selective labelingwith prompt tuning (SLPT) to boost performance in limited labels. Specifically, we introduce a feature-aware prompt updater to guide prompt tuning and aTandEm Selective LAbeling (TESLA) strategy. TESLA includes unsupervised diversity selection and supervised selection using prompt-based uncertainty. In addition, we propose a diversified visual prompt tuning strategy to provide multi-promptbased discrepant predictions for TESLA. We evaluate our method on liver tumor segmentation and achieve state-of-the-art performance, outperforming traditional fine-tuning with only 6% of tunable parameters, also achieving 94% of full-data performance by labeling only 5% of the data.
MoreTranslated text
Key words
Active Learning,Prompt Tuning,Segmentation
AI Read Science
Must-Reading Tree
Example
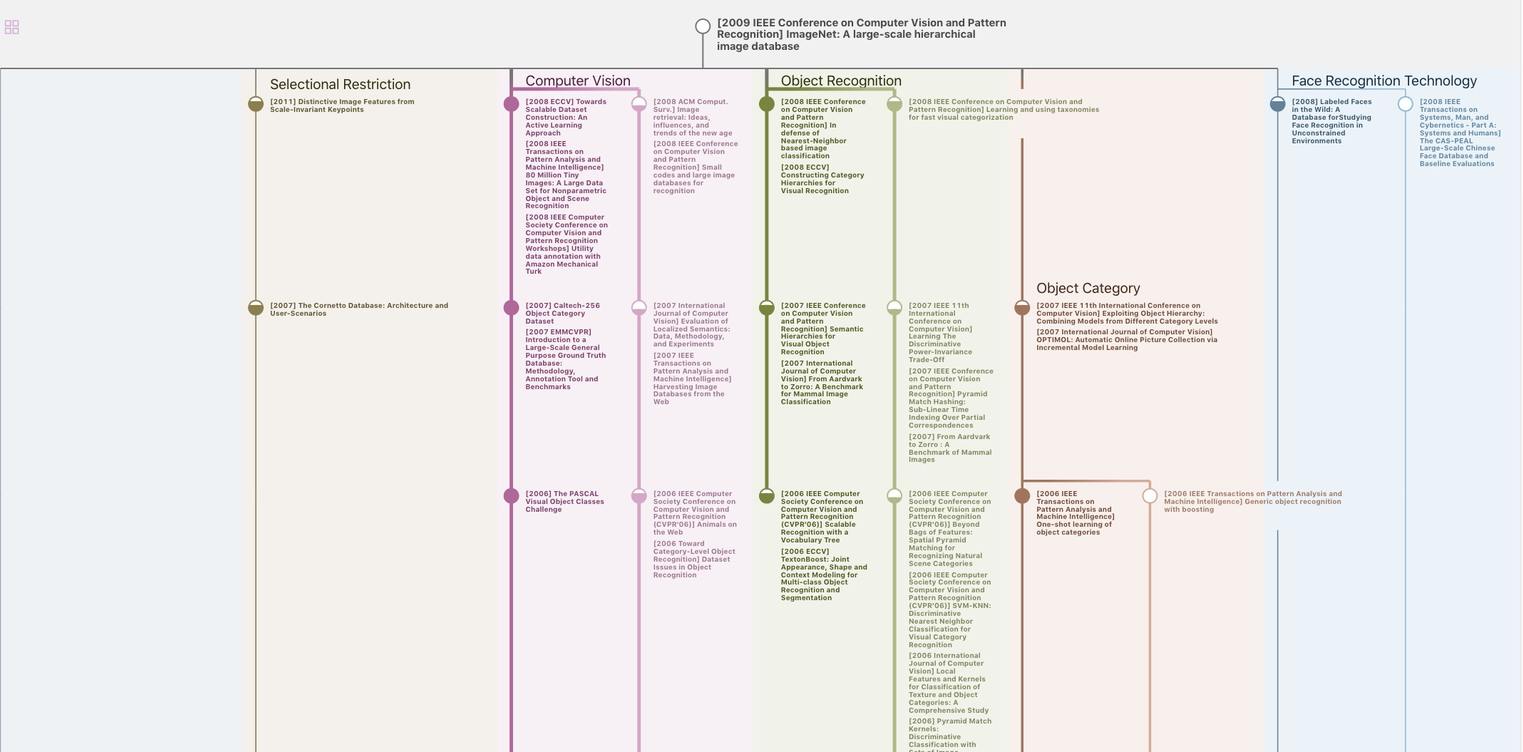
Generate MRT to find the research sequence of this paper
Chat Paper
Summary is being generated by the instructions you defined