Continual Road-Scene Semantic Segmentation via Feature-Aligned Symmetric Multi-Modal Network
CoRR(2023)
摘要
State-of-the-art multimodal semantic segmentation approaches combining LiDAR and color data are usually designed on top of asymmetric information-sharing schemes and assume that both modalities are always available. Regrettably, this strong assumption may not hold in real-world scenarios, where sensors are prone to failure or can face adverse conditions (night-time, rain, fog, etc.) that make the acquired information unreliable. Moreover, these architectures tend to fail in continual learning scenarios. In this work, we re-frame the task of multimodal semantic segmentation by enforcing a tightly-coupled feature representation and a symmetric information-sharing scheme, which allows our approach to work even when one of the input modalities is missing. This makes our model reliable even in safety-critical settings, as is the case of autonomous driving. We evaluate our approach on the SemanticKITTI dataset, comparing it with our closest competitor. We also introduce an ad-hoc continual learning scheme and show results in a class-incremental continual learning scenario that prove the effectiveness of the approach also in this setting.
更多查看译文
关键词
road-scene,feature-aligned,multi-modal
AI 理解论文
溯源树
样例
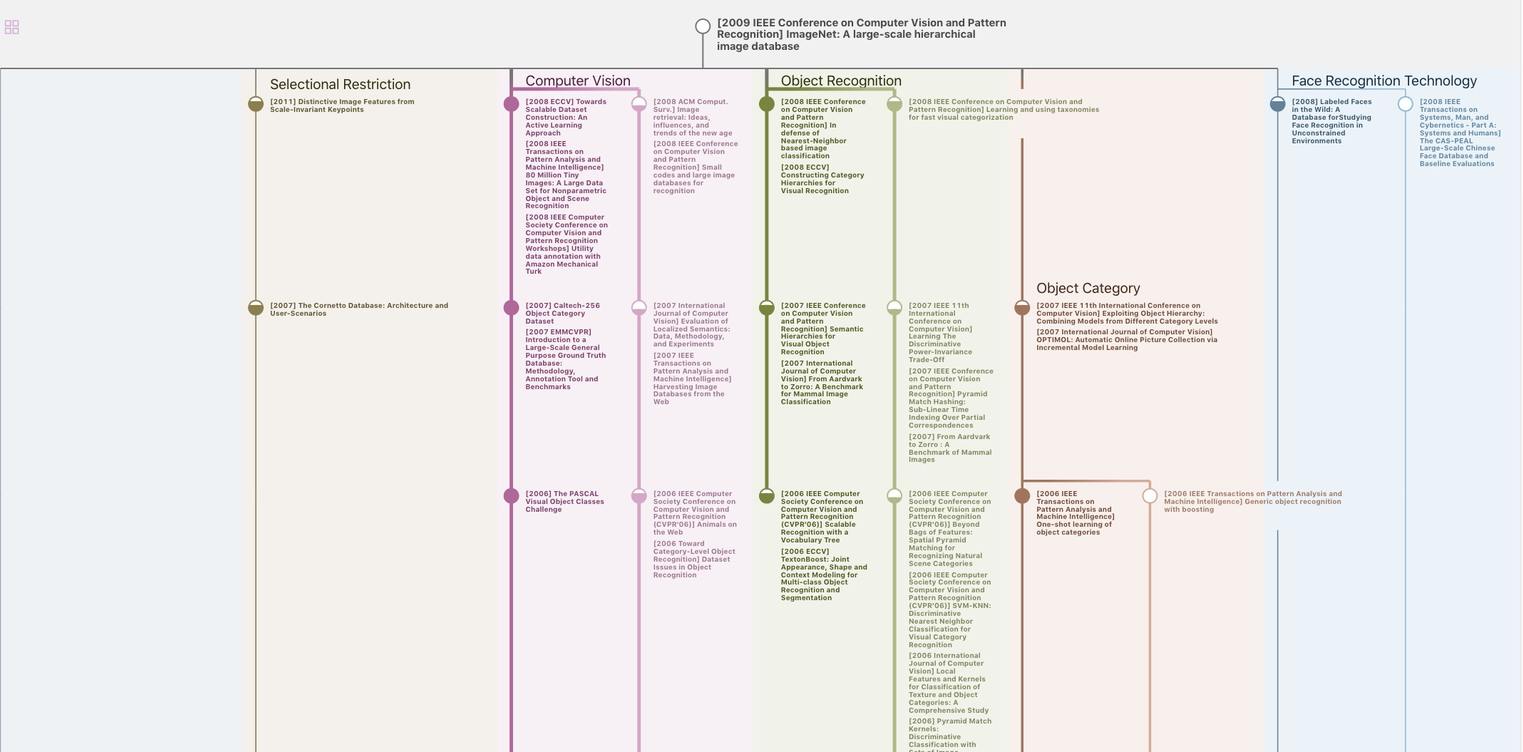
生成溯源树,研究论文发展脉络
Chat Paper
正在生成论文摘要