PSRFlow: Probabilistic Super Resolution with Flow-Based Models for Scientific Data
CoRR(2024)
摘要
Although many deep-learning-based super-resolution approaches have been proposed in recent years, because no ground truth is available in the inference stage, few can quantify the errors and uncertainties of the super-resolved results. For scientific visualization applications, however, conveying uncertainties of the results to scientists is crucial to avoid generating misleading or incorrect information. In this paper, we propose PSRFlow, a novel normalizing flow-based generative model for scientific data super-resolution that incorporates uncertainty quantification into the super-resolution process. PSRFlow learns the conditional distribution of the high-resolution data based on the low-resolution counterpart. By sampling from a Gaussian latent space that captures the missing information in the high-resolution data, one can generate different plausible super-resolution outputs. The efficient sampling in the Gaussian latent space allows our model to perform uncertainty quantification for the super-resolved results. During model training, we augment the training data with samples across various scales to make the model adaptable to data of different scales, achieving flexible super-resolution for a given input. Our results demonstrate superior performance and robust uncertainty quantification compared with existing methods such as interpolation and GAN-based super-resolution networks.
更多查看译文
关键词
probabilistic super resolution,scientific data,models,flow-based
AI 理解论文
溯源树
样例
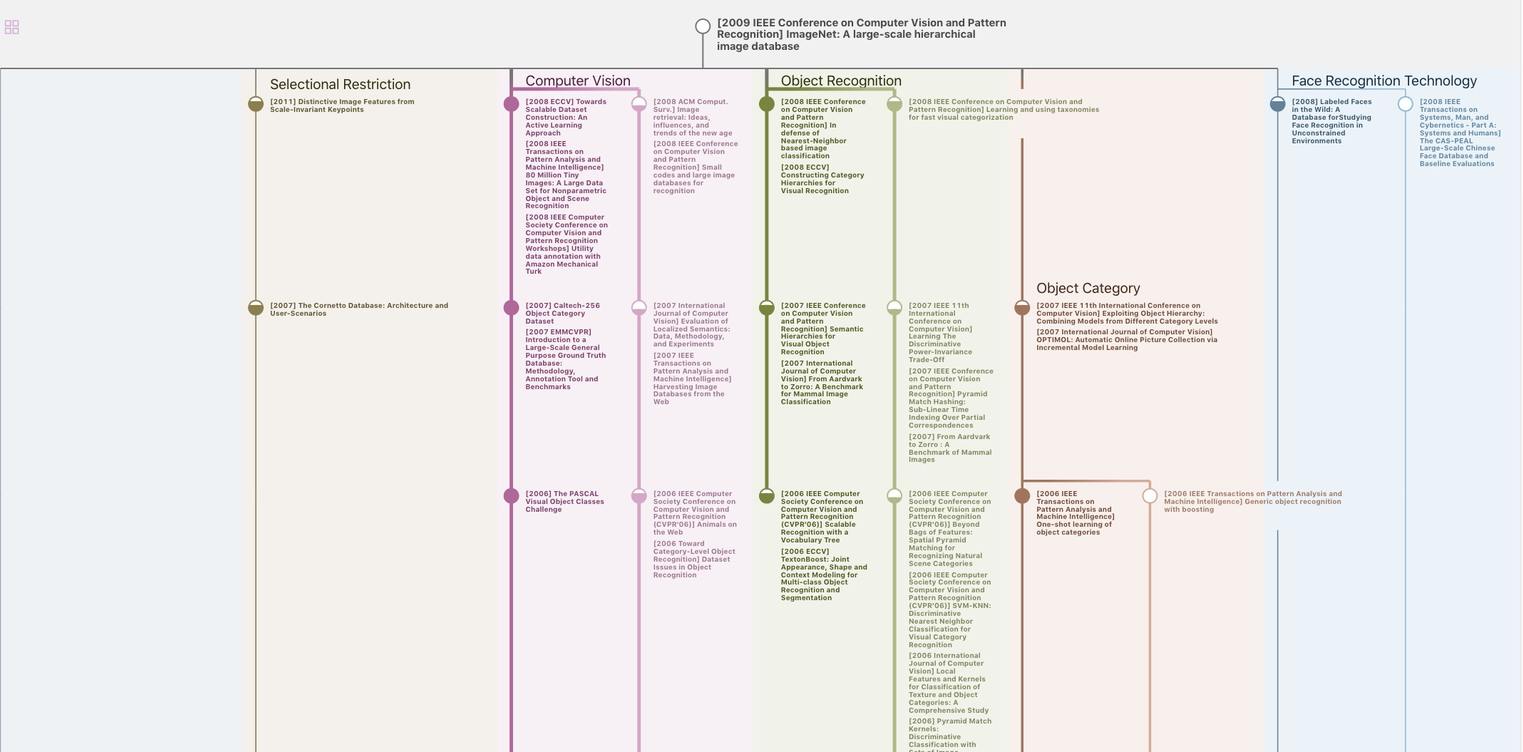
生成溯源树,研究论文发展脉络
Chat Paper
正在生成论文摘要