An Area and Energy Efficient All Resistive Neuromorphic-Computing Platform Implemented by a 4-Bit-per-cell RG-FinFET Memory
2023 INTERNATIONAL VLSI SYMPOSIUM ON TECHNOLOGY, SYSTEMS AND APPLICATIONS, VLSI-TSA/VLSI-DAT(2023)
关键词
16 parallel RG-FinFETs,4-bit-per-cell RG-FinFET memory arrays,ADC,ALL resistive neuromorphic computing platform,area size,building blocks,energy-efficient AI-Inference,excellent data retention,frequency 267.0 MHz,gradual tuning capability,ReLU,Restive-gate FinFET memory,symmetrical tuning capability,voltage 0.8 V
AI 理解论文
溯源树
样例
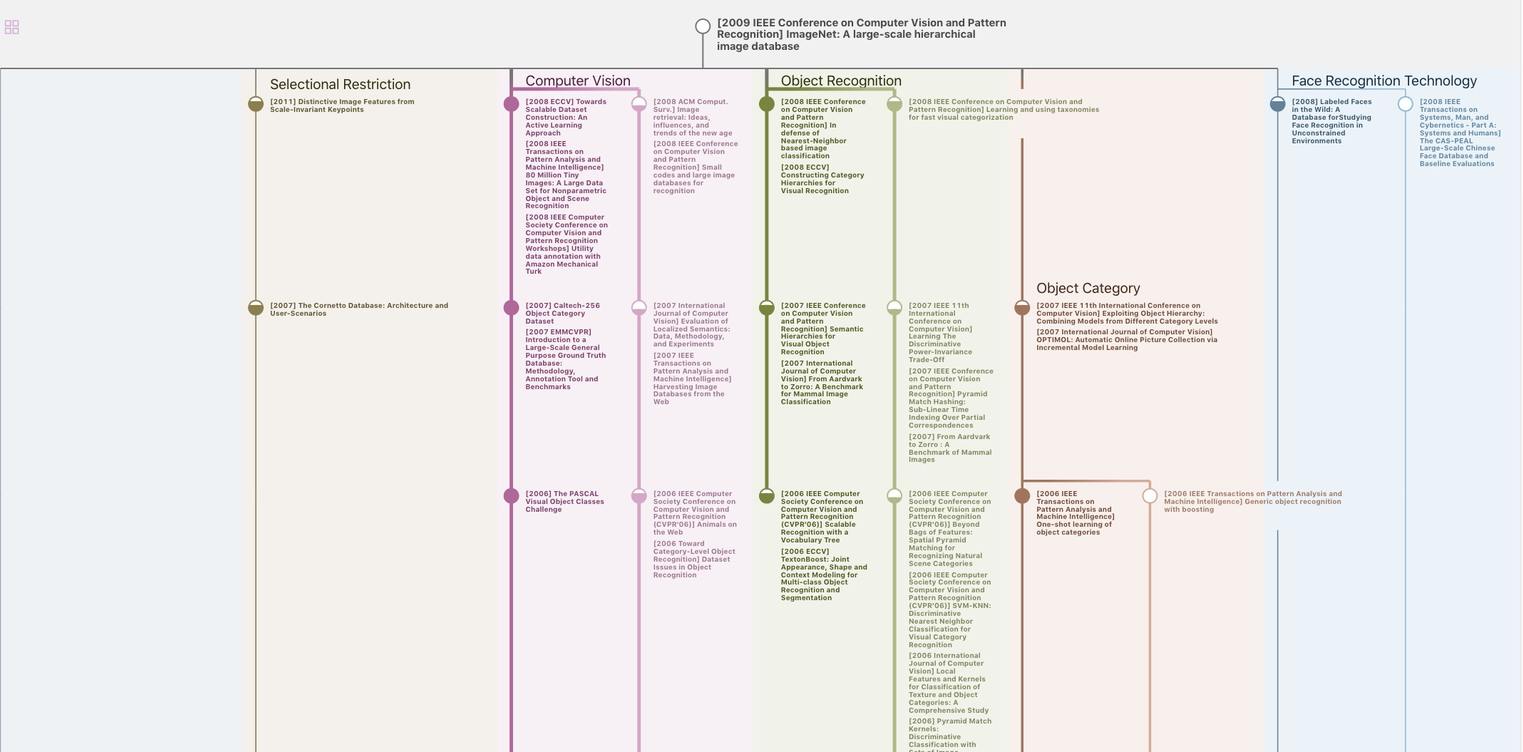
生成溯源树,研究论文发展脉络
Chat Paper
正在生成论文摘要