Maximizing supercontinuum bandwidths in gas-filled hollow-core fibers using artificial neural networks
JOURNAL OF APPLIED PHYSICS(2023)
摘要
Machine learning has been used to accelerate studies in the dynamics of optical pulses. In this study, we use machine learning to investigate the optimal design of supercontinuum-generating hollow-core antiresonant fibers (HC-ARFs) pressurized and filled with methane. Artificial neural networks (ANNs) are trained to replace the numerical solvers, accelerate the simulation of fibers, and provide a more rapid fiber design procedure. We first use an analytical model to approximate the dispersion and loss of the methane-filled silica HC-ARF. This approximation is used by the generalized unidirectional pulse propagation equation solver to simulate the pulse propagation and generate training data for our ANNs by varying design parameters including the pump center wavelength, fiber hollow-core radius, fiber length, cladding strut thickness, and gas pressure. We evaluate the performance of different spectral-predicting ANN architectures and use the trained ANN along with a custom fiber performance function to search the full parameter space. Subsequently, the regions with design parameters with predicted high performance are identified, and these high-performance HC-ARF designs are further optimized for supercontinuum generation at target supercontinuum bandwidths. We also extend our effort to maximizing the total spectral energy outside of the input pump wavelength by integrating the ANN with a global optimization technique for the search of an optimal HC-ARF design.
更多查看译文
关键词
supercontinuum bandwidths,neural networks,gas-filled,hollow-core
AI 理解论文
溯源树
样例
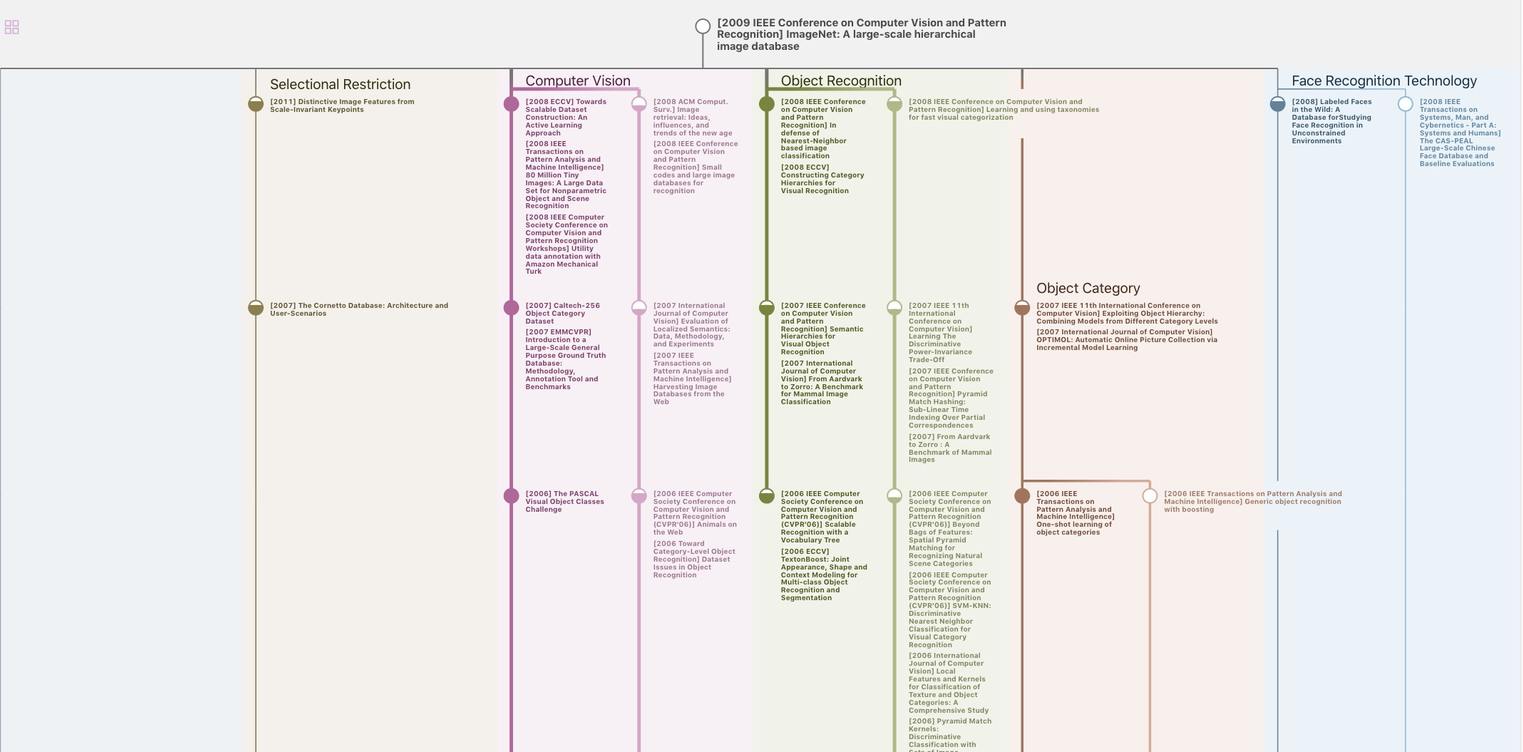
生成溯源树,研究论文发展脉络
Chat Paper
正在生成论文摘要