Self-Perceptual Generative Adversarial Network for Synthetic Aperture Sonar Image Generation
FOURTEENTH INTERNATIONAL CONFERENCE ON GRAPHICS AND IMAGE PROCESSING, ICGIP 2022(2022)
摘要
Due to the shortage of Synthetic Aperture Sonar ( SAS) image datasets, the development of many underwater tasks is hindered. To tackle this problem, coupling optical rendering and image-to-image translation is a novel and feasible way. However, because of the big gap between simulated optical images and real SAS images, the performances of existing works are not desired and have plenty of room for improvement. In this paper, we introduce a Self-Perceptual Generative Adversarial Network (SPerGAN) which can controllably generate SAS images with high fidelity. It utilizes a kind of self-perceptual loss to generate high-quality and diverse SAS images. Moreover, we introduce a novel evaluation method of SAS images that accords closely with human cognition. To evaluate the performance of our method, we first compare it against recent outstanding image-to-image translation methods on qualitative and quantitative aspects. Then we make ablation studies to explore the effects of different cycle consistency losses and hyper-parameters. The results show that our method can generate diverse and realistic SAS images.
更多查看译文
关键词
SAS image generation,generative adversarial network,image-to-image translation
AI 理解论文
溯源树
样例
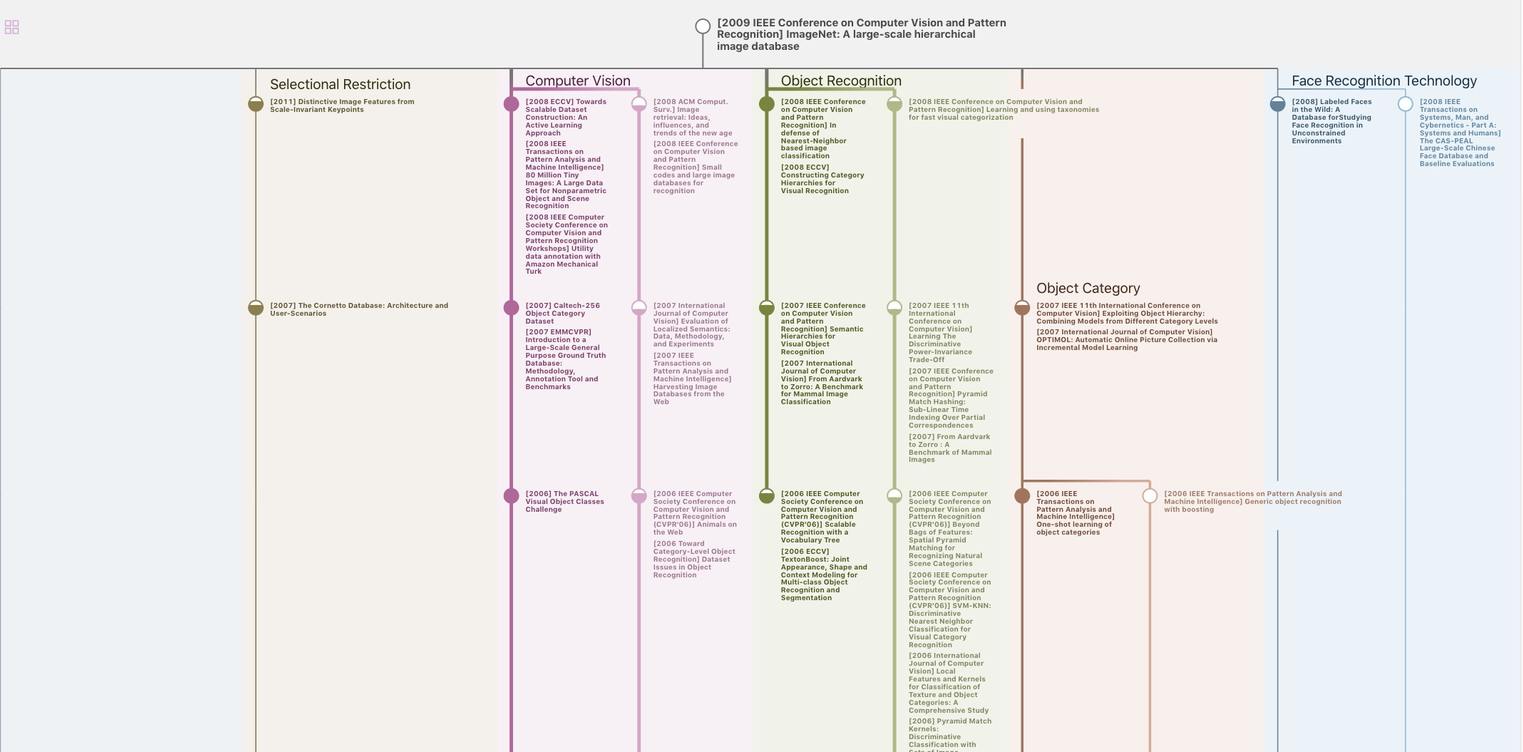
生成溯源树,研究论文发展脉络
Chat Paper
正在生成论文摘要