Characterization of Memory Access in Deep Learning and Its Implications in Memory Management
CMC-COMPUTERS MATERIALS & CONTINUA(2023)
摘要
Due to the recent trend of software intelligence in the Fourth Industrial Revolution, deep learning has become a mainstream workload for modern computer systems. Since the data size of deep learning increasingly grows, managing the limited memory capacity efficiently for deep learning workloads becomes important. In this paper, we analyze memory accesses in deep learning workloads and find out some unique characteristics differentiated from traditional workloads. First, when comparing instruction and data accesses, data access accounts for 96%-99% of total memory accesses in deep learning workloads, which is quite different from traditional workloads. Second, when comparing read and write accesses, write access dominates, accounting for 64%-80% of total memory accesses. Third, although write access makes up the majority of memory accesses, it shows a low access bias of 0.3 in the Zipf parameter. Fourth, in predicting re-access, recency is important in read access, but frequency provides more accurate information in write access. Based on these observations, we introduce a Non-Volatile Random Access Memory (NVRAM)-accelerated memory architecture for deep learning workloads, and present a new memory management policy for this architecture. By considering the memory access characteristics of deep learning workloads, the proposed policy improves memory performance by 64.3% on average compared to the CLOCK policy.
更多查看译文
关键词
Memory access,deep learning,machine learning,memory management,CLOCK
AI 理解论文
溯源树
样例
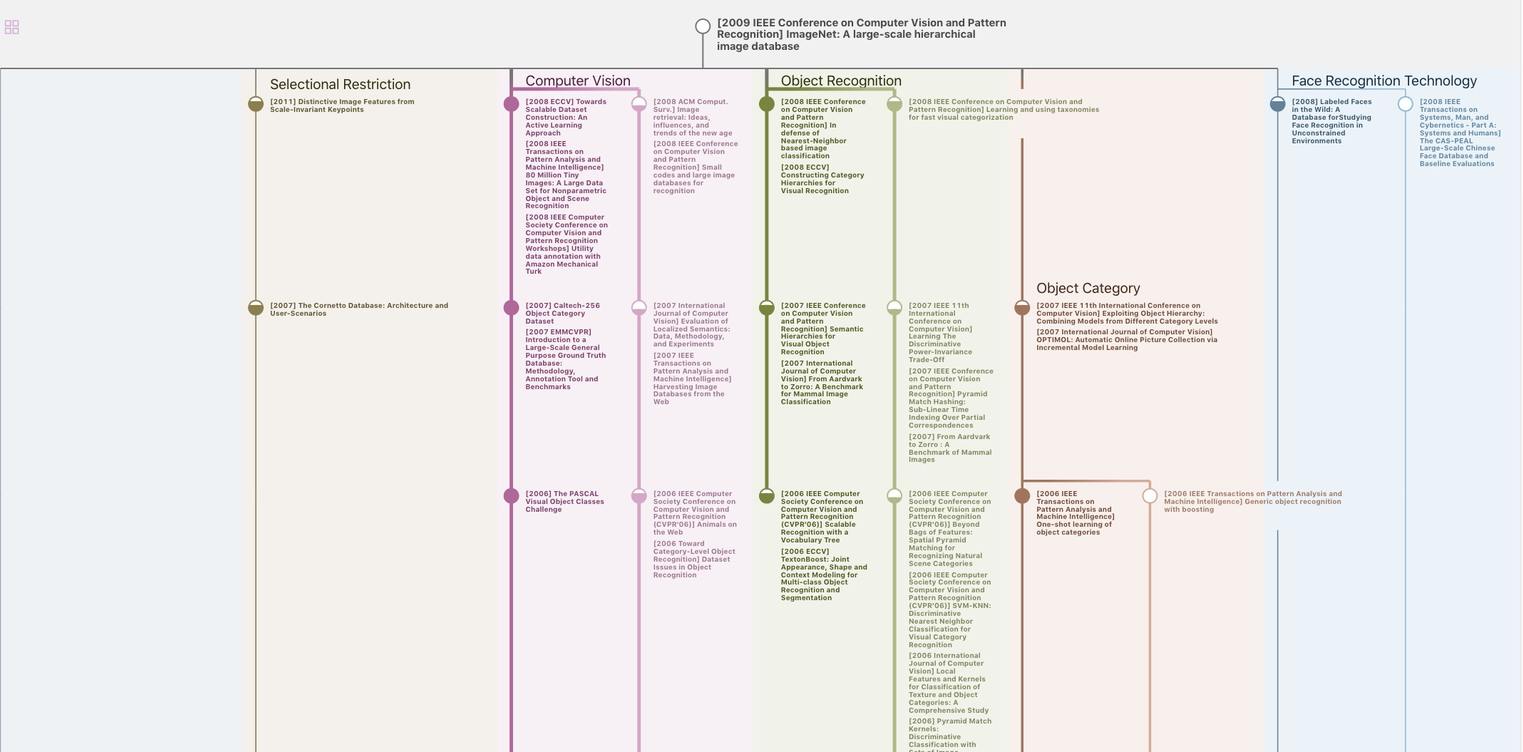
生成溯源树,研究论文发展脉络
Chat Paper
正在生成论文摘要