Seismic image super-resolution reconstruction through deep feature mining network
APPLIED INTELLIGENCE(2023)
摘要
Due to complex geological conditions and instrument inaccuracy, raw seismic data are often characterized by low resolution. Deep learning is an emerging technique for seismic resolution improvement; however, its performance is often limited by the small amount of labeled data available. In this paper, we design a deep feature mining network (DFMN) to deal with this issue. DFMN has three components: shallow feature extraction block (SFB), deep feature mining block (DFB), and enhanced reconstruction block (ERB). First, the SFB component uses multi-scale kernels to learn rich information from low-resolution data. The convolutions incorporate the benefits of different kernel sizes, which are effective for shallow feature extraction. Second, the DFB component employs a dual-branch network architecture for deep feature mining. The dual-branch network learns more complementary features than a single-branch network, thus alleviating the requirement for large amounts of training data. Third, the ERB component combines the shuffled image and the interpolated image during reconstruction. Interpolated images, incorporating prior knowledge, can provide more contextual information in our model. The results show that DFMN is superior to a traditional upscaling algorithm and other deep learning methods in terms of (1) perceptual effects: more complete structural information, such as texture details; (2) quantitative evaluation indices: higher PSNR and SSIM; and (3) generalization ability: better performance on other data.
更多查看译文
关键词
Feature extraction,Interpolation,Seismic image,Super-resolution
AI 理解论文
溯源树
样例
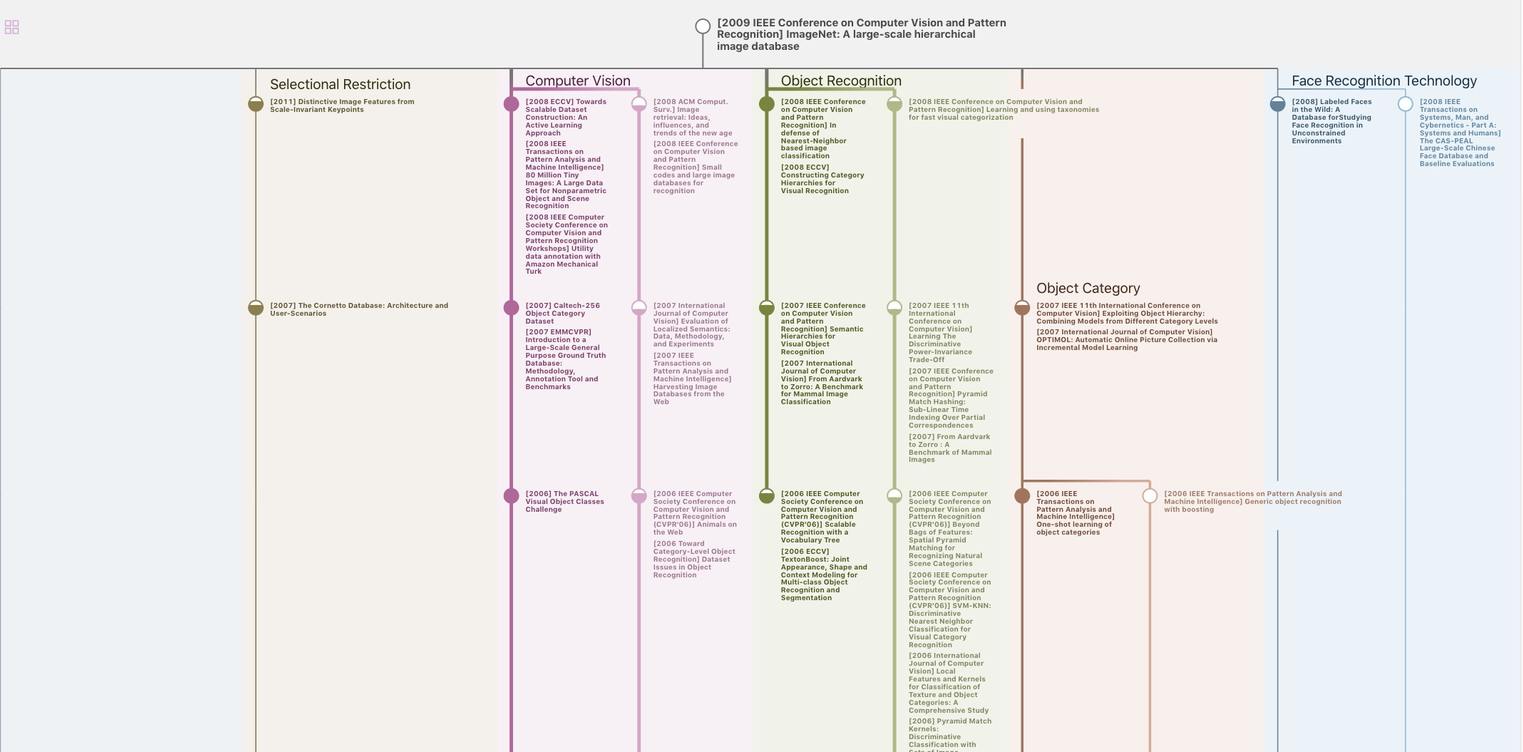
生成溯源树,研究论文发展脉络
Chat Paper
正在生成论文摘要