Bayesian diagnostic learning for a costly composite manufacturing: critical role of dataset size and auxiliary in situ measurements
INTERNATIONAL JOURNAL OF ADVANCED MANUFACTURING TECHNOLOGY(2023)
摘要
In the context of smart manufacturing with costly process trials (e.g., those in the aerospace sector), access to data-efficient, transparent, and diagnostic -capable inverse AI models is a key. This is because in such settings, the concurrent presence of numerous tool/material/process decision factors along with their potential uncertainties can rapidly yield the final product failure; hence, designers need to proactively predict and mitigate the underlying root causes. Towards this technological motivation, in this work, different Bayesian learning frameworks with inverse modelling capabilities have been developed and tested for a composites autoclave curing process, while assuming different training dataset size scenarios. We specifically present a new fully connected Bayesian Belief Network (BBN) architecture integrated with an expert-located proxy thermocouple within the manufacturing setup, to learn the thermal behavior of the final composite laminate rapidly. The results revealed that the integration of such expert knowledge under the BBN model can offer favorable predictive performance. It is shown that the model even with limited (e.g., 0.03% of the full set) but highly customized auxiliary data still yields useful and interpretable inverse decisions (with a maximum state likelihood of 0.9) for the process failure mitigation, thanks to the model’s apparent transparency based on the BBNs’ directed graph nature.
更多查看译文
关键词
Bayesian Belief Networks,Composites autoclave processing,Simulation data,Inverse modelling,Process failure diagnosis
AI 理解论文
溯源树
样例
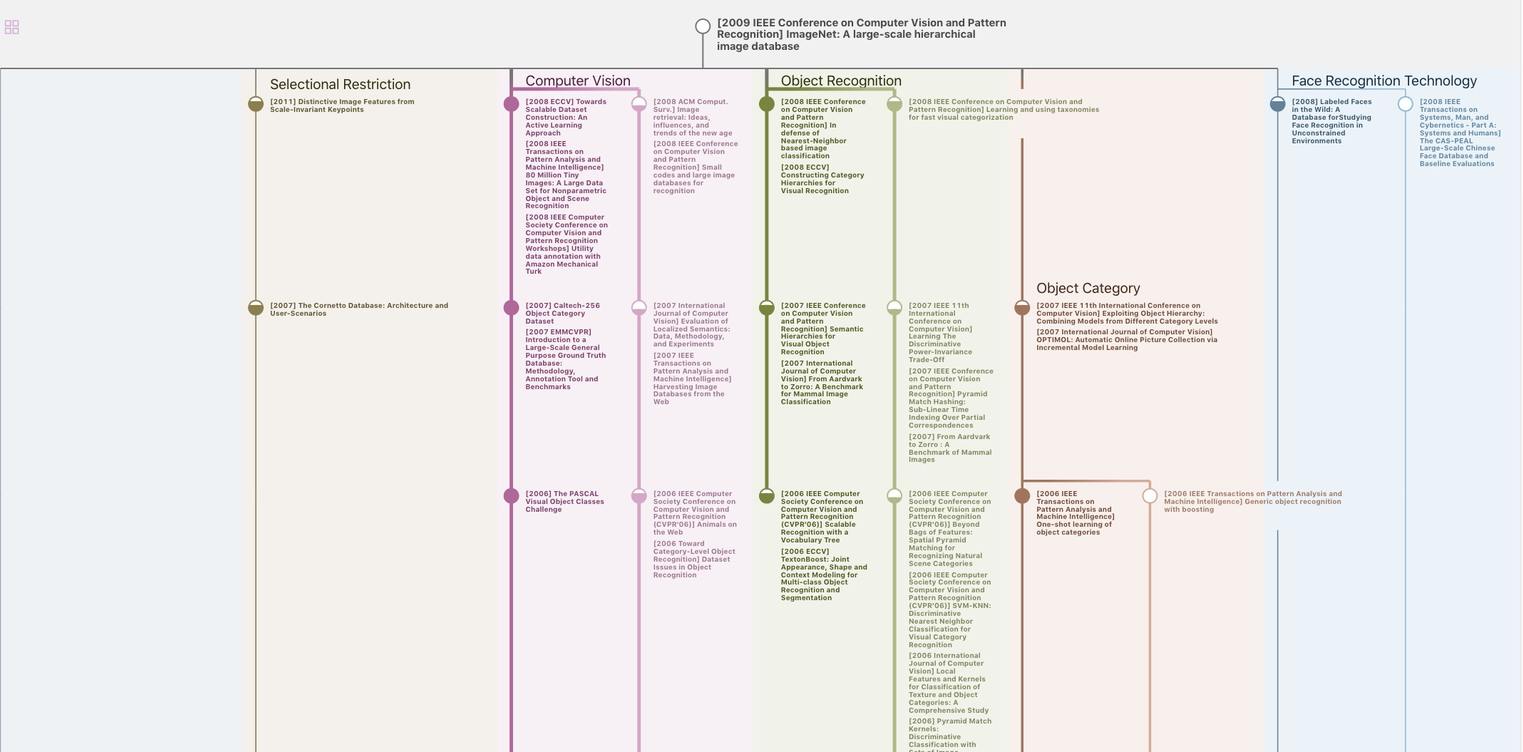
生成溯源树,研究论文发展脉络
Chat Paper
正在生成论文摘要