Prior knowledge-based self-supervised learning for intelligent bearing fault diagnosis with few fault samples
MEASUREMENT SCIENCE AND TECHNOLOGY(2023)
摘要
Deep learning-based bearing fault diagnosis methods have been developed to learn fault knowledge from massive data. Owing to the deficiency of fault samples and the variability of working conditions, these deep learning-based methods are limited in industrial applications. To address this problem, this study proposes a prior knowledge-based self-supervised learning (PKSSL) method for bearing fault diagnosis. In the PKSSL method, prior diagnostic knowledge is extracted by meta-learning from a few samples. Prior diagnostic knowledge is then utilized to guide the self-supervised learning (SSL) process to reduce reliance on training data. Furthermore, a graph convolutional network is introduced to fuse the information obtained by meta-learning and SSL, which makes the model fully utilize the learned information and improves the accuracy of the fault diagnosis. The effectiveness of the proposed method was validated using two datasets. The results demonstrate that compared to other existing approaches, the proposed method exhibits a strong generalization ability to transfer diagnostic knowledge from artificial damage data to real damage data under varied operating conditions.
更多查看译文
关键词
few-shot,meta-learning,self-supervised learning,graph convolutional network,fault diagnosis
AI 理解论文
溯源树
样例
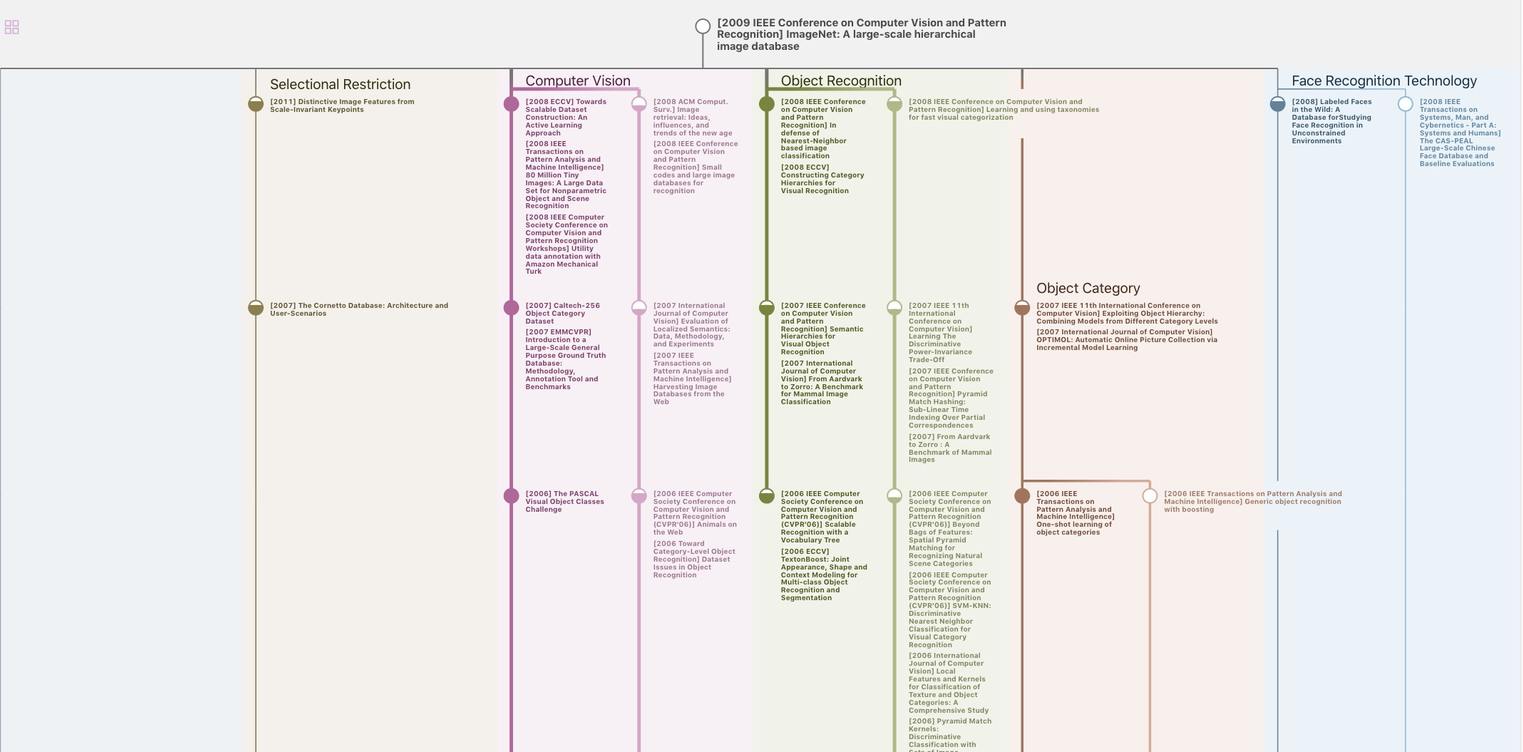
生成溯源树,研究论文发展脉络
Chat Paper
正在生成论文摘要