ResViT-Rice: A Deep Learning Model Combining Residual Module and Transformer Encoder for Accurate Detection of Rice Diseases
AGRICULTURE-BASEL(2023)
摘要
Rice is a staple food for over half of the global population, but it faces significant yield losses: up to 52% due to leaf blast disease and brown spot diseases, respectively. This study aimed at proposing a hybrid architecture, namely ResViT-Rice, by taking advantage of both CNN and transformer for accurate detection of leaf blast and brown spot diseases. We employed ResNet as the backbone network to establish a detection model and introduced the encoder component from the transformer architecture. The convolutional block attention module was also integrated to ResViT-Rice to further enhance the feature-extraction ability. We processed 1648 training and 104 testing images for two diseases and the healthy class. To verify the effectiveness of the proposed ResViT-Rice, we conducted comparative evaluation with popular deep learning models. The experimental result suggested that ResViT-Rice achieved promising results in the rice disease-detection task, with the highest accuracy reaching 0.9904. The corresponding precision, recall, and F1-score were all over 0.96, with an AUC of up to 0.9987, and the corresponding loss rate was 0.0042. In conclusion, the proposed ResViT-Rice can better extract features of different rice diseases, thereby providing a more accurate and robust classification output.
更多查看译文
关键词
transformer encoder,deep learning,resvit-rice
AI 理解论文
溯源树
样例
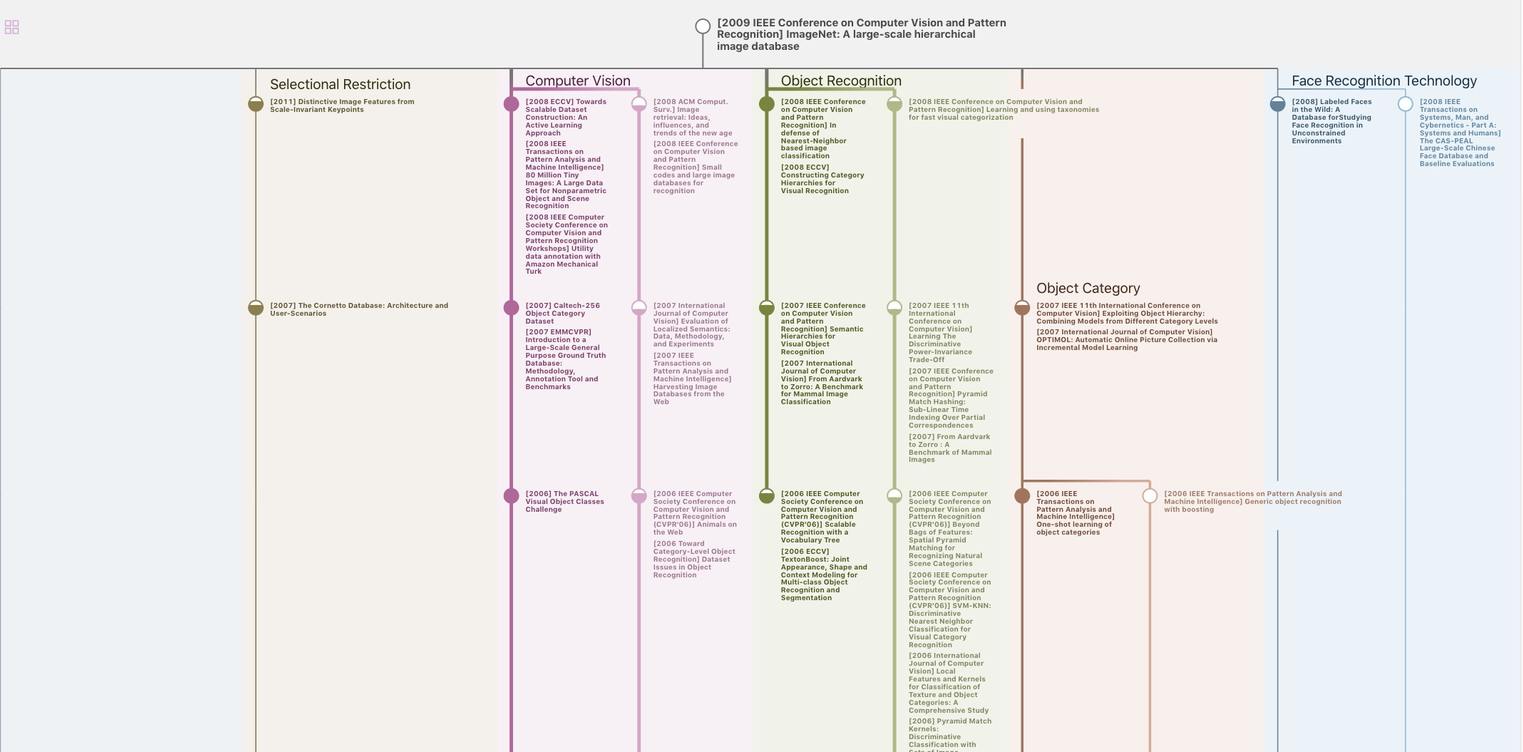
生成溯源树,研究论文发展脉络
Chat Paper
正在生成论文摘要