Federated Inference With Reliable Uncertainty Quantification Over Wireless Channels via Conformal Prediction
IEEE TRANSACTIONS ON SIGNAL PROCESSING(2024)
摘要
In this paper, we consider a wireless federated inference scenario in which devices and a server share a pre-trained machine learning model. The devices communicate statistical information about their local data to the server over a common wireless channel, aiming to enhance the quality of the inference decision at the server. Recent work has introduced federated conformal prediction (CP), which leverages devices-to-server communication to improve the reliability of the server's decision. With federated CP, devices communicate to the server information about the loss accrued by the shared pre-trained model on the local data, and the server leverages this information to calibrate a decision interval, or set, so that it is guaranteed to contain the correct answer with a pre-defined target reliability level. Previous work assumed noise-free communication, whereby devices can communicate a single real number to the server. In this paper, we study for the first time federated CP in a wireless setting. We introduce a novel protocol, termed wireless federated conformal prediction (WFCP), which builds on type-based multiple access (TBMA) and on a novel quantile correction strategy. WFCP is proved to provide formal reliability guarantees in terms of coverage of the predicted set produced by the server. Using numerical results, we demonstrate the significant advantages of WFCP against digital implementations of existing federated CP schemes, especially in regimes with limited communication resources and/or large number of devices.
更多查看译文
关键词
Servers,Reliability,Wireless communication,Histograms,Protocols,Data models,Predictive models,Conformal prediction,federated inference,wireless communications,type-based multiple access
AI 理解论文
溯源树
样例
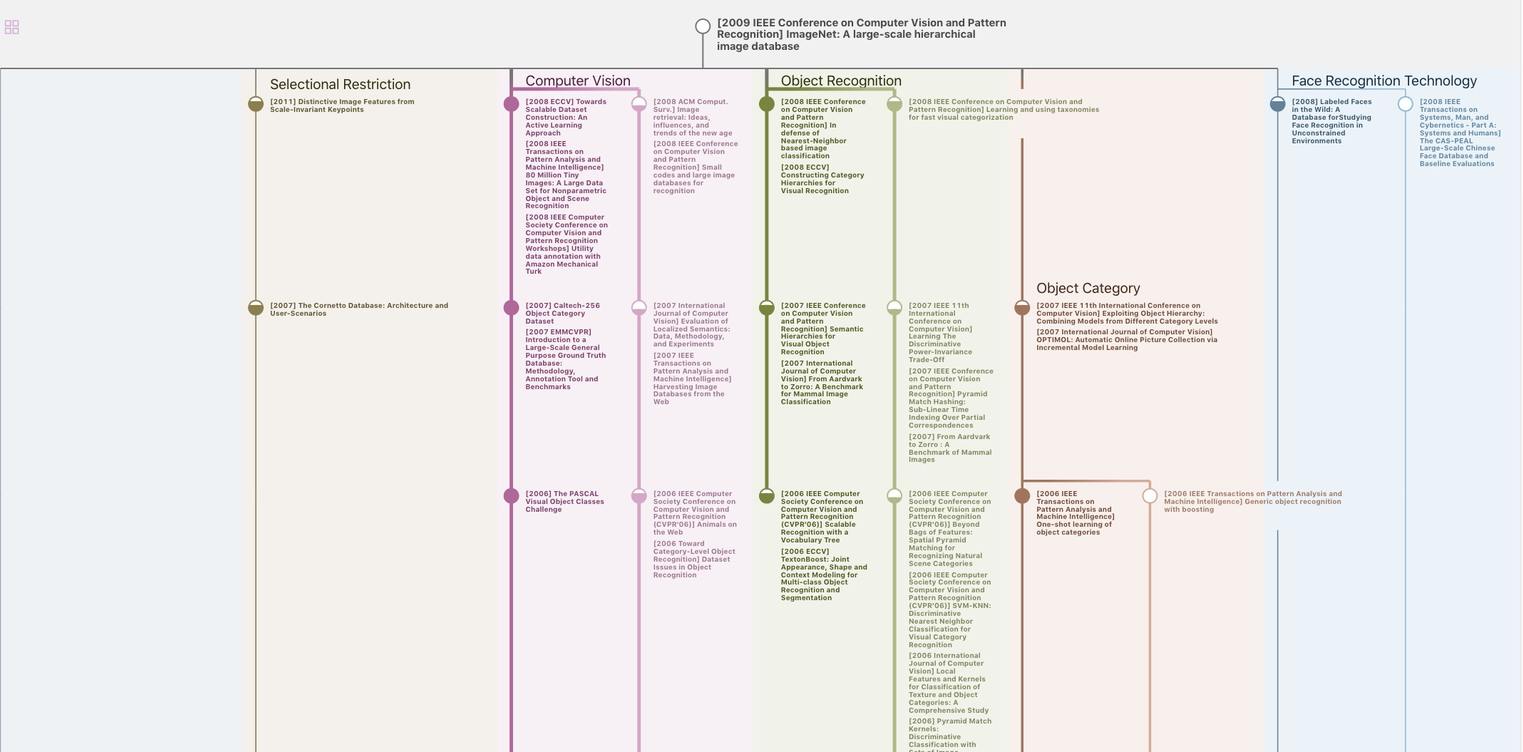
生成溯源树,研究论文发展脉络
Chat Paper
正在生成论文摘要