A hierarchical Bayesian model for estimating age-specific COVID-19 infection fatality rates in developing countries
arXiv (Cornell University)(2023)
摘要
The COVID-19 infection fatality rate (IFR) is the proportion of individuals infected with SARS-CoV-2 who subsequently die. As COVID-19 disproportionately affects older individuals, age-specific IFR estimates are imperative to facilitate comparisons of the impact of COVID-19 between locations and prioritize distribution of scare resources. However, there lacks a coherent method to synthesize available data to create estimates of IFR and seroprevalence that vary continuously with age and adequately reflect uncertainties inherent in the underlying data. In this paper we introduce a novel Bayesian hierarchical model to estimate IFR as a continuous function of age that acknowledges heterogeneity in population age structure across locations and accounts for uncertainty in the estimates due to seroprevalence sampling variability and the imperfect serology test assays. Our approach simultaneously models test assay characteristic, serology, and death data, where the serology and death data are often available only for binned age groups. Information is shared across locations through hierarchical modeling to improve estimation of the parameters with limited data. Modeling data from 26 developing country locations during the first year of the COVID-19 pandemic, we found seroprevalence did not change dramatically with age, and the IFR at age 60 was above the high-income country benchmark for most locations.
更多查看译文
关键词
infection fatality rates,hierarchical bayesian model,countries,age-specific
AI 理解论文
溯源树
样例
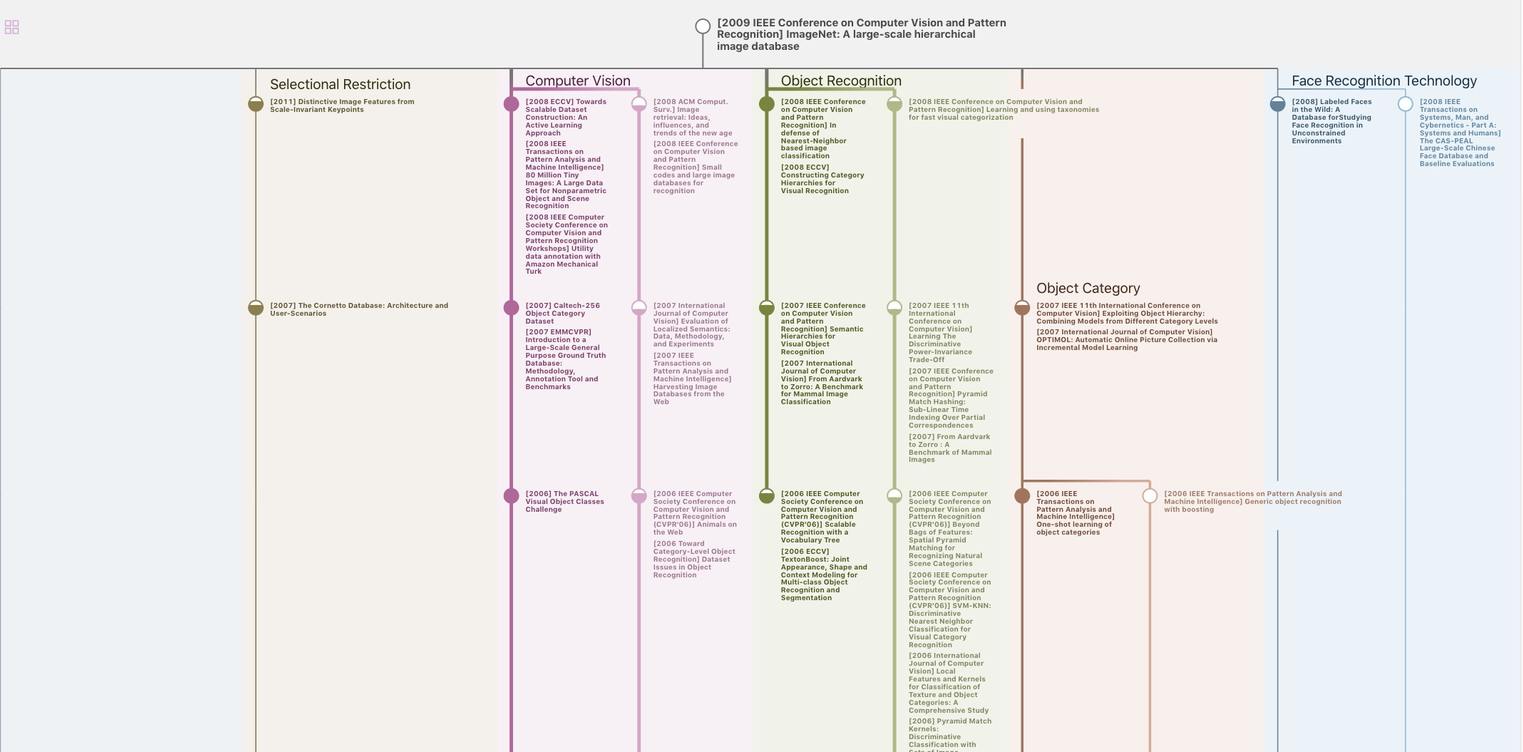
生成溯源树,研究论文发展脉络
Chat Paper
正在生成论文摘要