Self-Supervised Graph Completion for Incomplete Multi-View Clustering
IEEE Transactions on Knowledge and Data Engineering(2023)
摘要
Incomplete multi-view clustering (IMVC) is challenging, as it requires adequately exploring complementary and consistency information under the incompleteness of data. Most existing approaches attempt to overcome the incompleteness at instance-level. In this work, we develop a new approach to facilitate IMVC from a new perspective. Specifically, we transfer the issue of missing instances to a similarity graph completion problem for incomplete views, and propose a self-supervised multi-view graph completion algorithm to infer the associated missing entries. Further, by incorporating constrained feature learning, the inferred graph can be naturally leveraged in representation learning. We theoretically show that our feature learning process performs an Auto-Regressive filter function by encoding the learned similarity graph, which could yield discriminative representation for a clustering task. Extensive experiments demonstrate the effectiveness of the proposed method in comparison with state-of-the-art methods.
更多查看译文
关键词
graph completion,clustering,self-supervised,multi-view
AI 理解论文
溯源树
样例
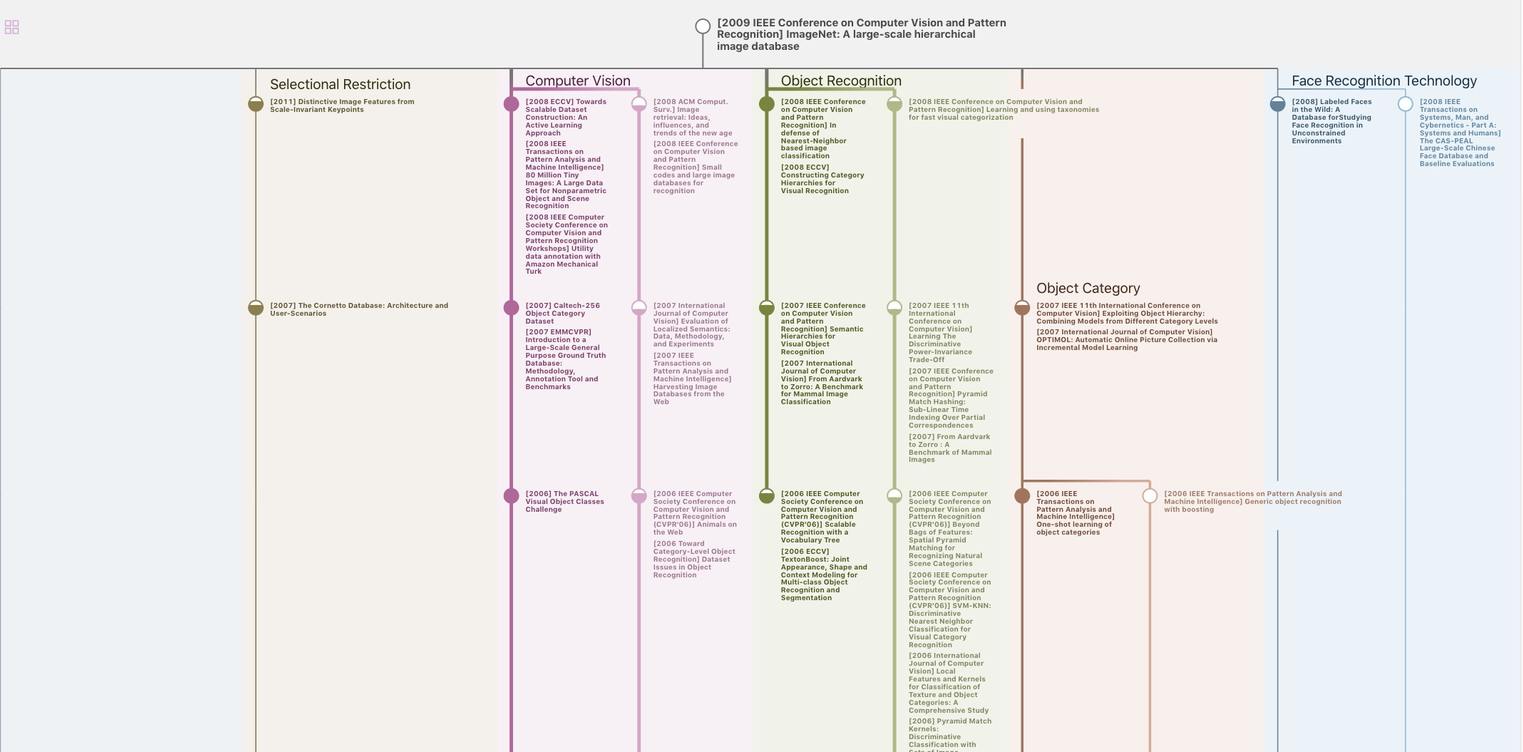
生成溯源树,研究论文发展脉络
Chat Paper
正在生成论文摘要