A Primer on Variational Laplace (VL).
NeuroImage(2023)
摘要
This article details a scheme for approximate Bayesian inference, which has underpinned thousands of neuroimaging studies since its introduction 15 years ago. Variational Laplace (VL) provides a generic approach to fitting linear or non-linear models, which may be static or dynamic, returning a posterior probability density over the model parameters and an approximation of log model evidence, which enables Bayesian model comparison. VL applies variational Bayesian inference in conjunction with quadratic or Laplace approximations of the evidence lower bound (free energy). Importantly, update equations do not need to be derived for each model under consideration, providing a general method for fitting a broad class of models. This primer is intended for experimenters and modellers who may wish to fit models to data using variational Bayesian methods, without assuming previous experience of variational Bayes or machine learning. Accompanying code demonstrates how to fit different kinds of model using the reference implementation of the VL scheme in the open-source Statistical Parametric Mapping (SPM) software package. In addition, we provide a standalone software function that does not require SPM, in order to ease translation to other fields, together with detailed pseudocode. Finally, the supplementary materials provide worked derivations of the key equations.
更多查看译文
关键词
Variational Laplace,Bayes,DCM,Modelling
AI 理解论文
溯源树
样例
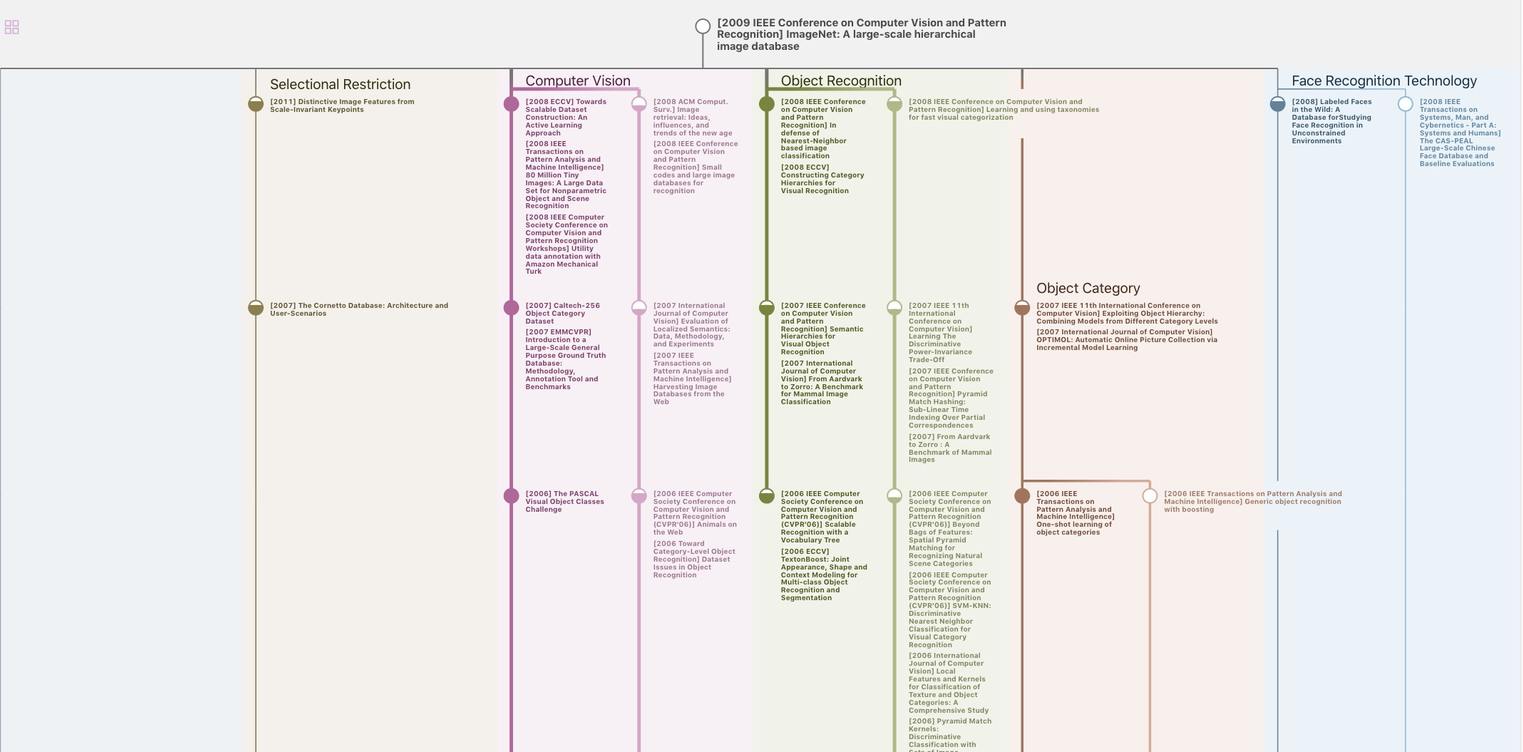
生成溯源树,研究论文发展脉络
Chat Paper
正在生成论文摘要