Learning-Based Parameter Optimization for a Class of Orbital Tracking Control Laws
The Journal of the Astronautical Sciences(2024)
摘要
This paper presents a learning algorithm for tuning the parameters of a family of stabilizing nonlinear controllers for orbital tracking, in order to minimize a cost function which combines convergence time and fuel consumption. The main feature of the proposed approach is that it achieves performance optimization while guaranteeing closed-loop stability of the resulting controller. This property is exploited also to restrict the class of admissible controllers and hence to expedite the training process. The learning algorithm is tested on three case studies: two different orbital transfers and a rendezvous mission. Numerical simulations show that the learned control parameters lead to a significant improvement of the considered performance measure.
更多查看译文
关键词
Orbital tracking,Control design,Parameter optimization,Learning
AI 理解论文
溯源树
样例
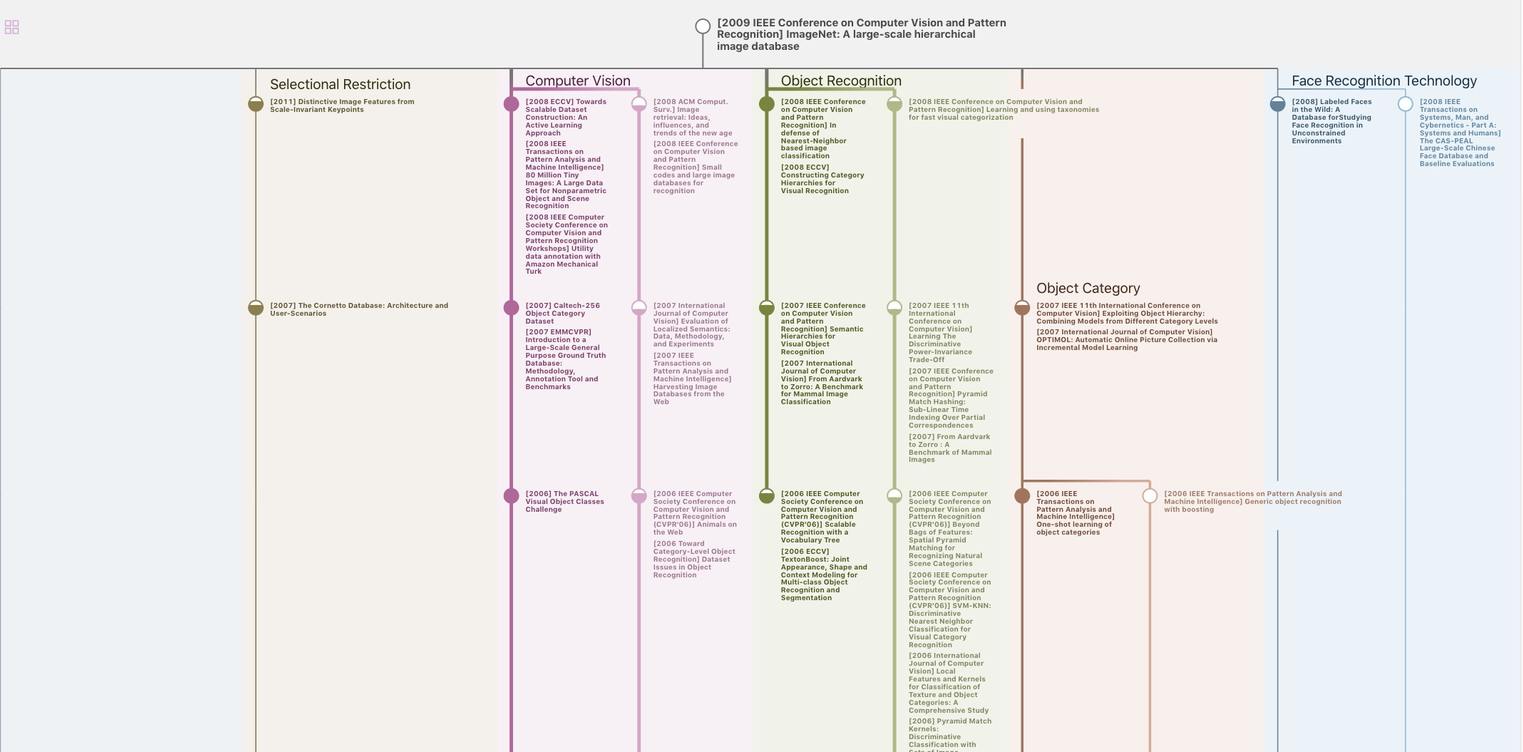
生成溯源树,研究论文发展脉络
Chat Paper
正在生成论文摘要