Multi-View Graph Convolutional Network for Multimedia Recommendation
CoRR(2023)
摘要
Multimedia recommendation has received much attention in recent years. It models user preferences based on both behavior information and item multimodal information. Though current GCN-based methods achieve notable success, they suffer from two limitations: (1) Modality noise contamination to the item representations. Existing methods often mix modality features and behavior features in a single view (e.g., user-item view) for propagation, the noise in the modality features may be amplified and coupled with behavior features. In the end, it leads to poor feature discriminability; (2) Incomplete user preference modeling caused by equal treatment of modality features. Users often exhibit distinct modality preferences when purchasing different items. Equally fusing each modality feature ignores the relative importance among different modalities, leading to the suboptimal user preference modeling. To tackle the above issues, we propose a novel Multi-View Graph Convolutional Network for the multimedia recommendation. Specifically, to avoid modality noise contamination, the modality features are first purified with the aid of item behavior information. Then, the purified modality features of items and behavior features are enriched in separate views, including the user-item view and the item-item view. In this way, the distinguishability of features is enhanced. Meanwhile, a behavior-aware fuser is designed to comprehensively model user preferences by adaptively learning the relative importance of different modality features. Furthermore, we equip the fuser with a self-supervised auxiliary task. This task is expected to maximize the mutual information between the fused multimodal features and behavior features, so as to capture complementary and supplementary preference information simultaneously. Extensive experiments on three public datasets demonstrate the effectiveness of our methods.
更多查看译文
AI 理解论文
溯源树
样例
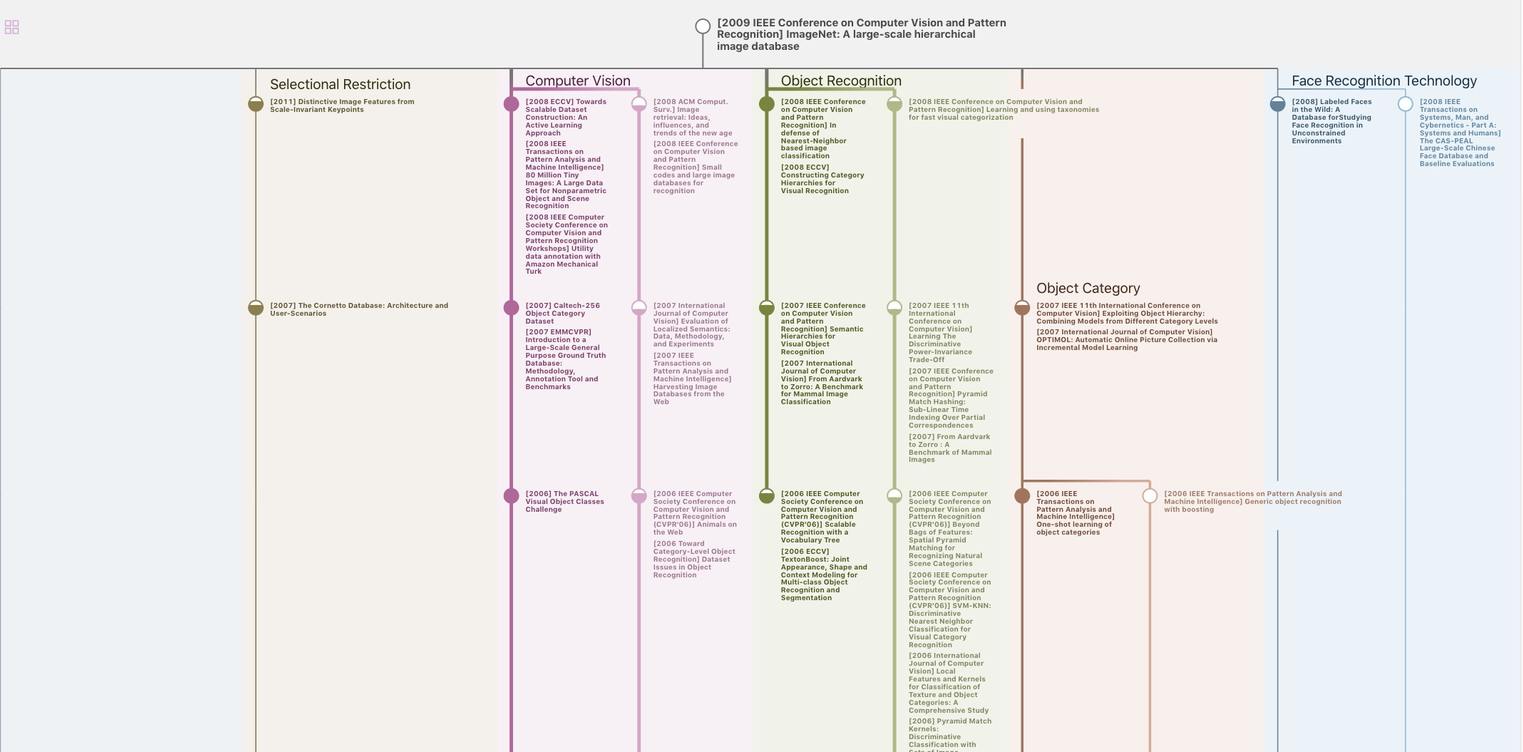
生成溯源树,研究论文发展脉络
Chat Paper
正在生成论文摘要