Clusternets: a deep learning approach to probe clustering dark energy
MONTHLY NOTICES OF THE ROYAL ASTRONOMICAL SOCIETY(2024)
摘要
Machine learning (ML) algorithms are becoming popular in cosmology for extracting valuable information from cosmological data. In this paper, we evaluate the performance of a convolutional neural network (CNN) trained on matter density snapshots to distinguish clustering dark energy (DE) from the cosmological constant scenario and to detect the speed of sound (c(s)) associated with clustering DE. We compare the CNN results with those from a Random Forest (RF) algorithm trained on power spectra. Varying the DE equation of state parameter w(DE) within the range of -0.7 to -0.99 while keeping c(s)(2)= 1, we find that the CNN approach results in a significant improvement in accuracy over the RF algorithm. The improvement in classification accuracy can be as high as 40 per cent depending on the physical scales involved. We also investigate the ML algorithms' ability to detect the impact of the speed of sound by choosing c(s)(2) from the set {1, 10(-2), 10(-4), 10(-7)} while maintaining a constant w(DE) for three different cases: w(DE) is an element of {-0.7, -0.8, -0.9}. Our results suggest that distinguishing between various values of c(s)(2) and the case where c(s)(2)=1 is challenging, particularly at small scales and when w(DE) approximate to -1. However, as we consider larger scales, the accuracy of c(s)(2) detection improves. Notably, the CNN algorithm consistently outperforms the RF algorithm, leading to an approximate 20 per cent enhancement in c(s)(2) detection accuracy in some cases.
更多查看译文
关键词
methods: numerical,methods: statistical,software: data analysis,software: simulations,dark energy,large-scale structure of Universe
AI 理解论文
溯源树
样例
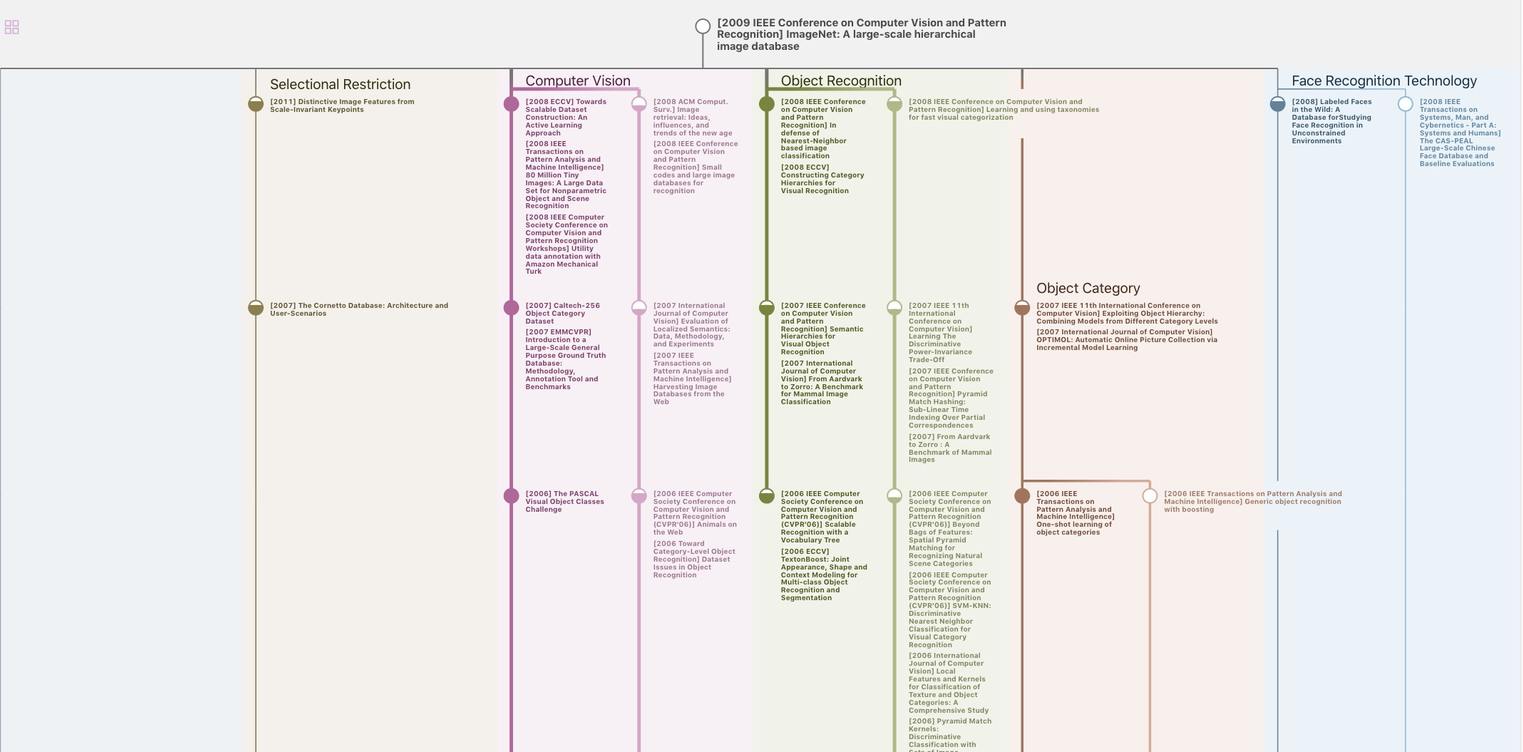
生成溯源树,研究论文发展脉络
Chat Paper
正在生成论文摘要