Non-parametric maximum likelihood component separation for CMB polarization data
arXiv (Cornell University)(2023)
摘要
Mitigation of the impact of foreground contributions to measurements of
Cosmic Microwave Background (CMB) polarization is a crucial step in modern CMB
data analysis and is of particular importance for a detection of large-scale
CMB B modes. A large variety of techniques, based on different assumptions
and aiming at either a full component separation or merely cleaning the
foreground signals from the CMB maps, have been described in the literature. In
this work, we consider this problem within a unified framework based on the
maximum likelihood principle, under the assumption that the signal at each
frequency can be represented as a linear mixture of sky templates. We discuss
the impact of various additional assumptions on the final outcome of the
procedure. We find that the component separation problem can be fully solved in
two specific situations: when we either know the frequency scaling of all the
components or can correctly model them with a limited number of unknown
parameters, as is the case in the parametric component separation techniques;
or when we either know the statistical properties of all the components, the
foregrounds and CMB, or can correctly model them with a limited number of
parameters, as for instance in SMICA-like approaches. However, we also show
that much less stringent assumptions are sufficient if we only aim at
recovering the cleaned CMB signal. In particular, we discuss a “minimally
informed” non-parametric method based on maximum likelihood. The method only
assumes that the component properties are independent on the sky direction, at
least over some region of the sky, and that the CMB covariance is known up to
some limited number of parameters. We apply this method to recover the CMB B
modes polarization signal in the context of forthcoming CMB experiments and
compare its performance with that of the standard parametric... (abridged)
更多查看译文
关键词
separation,non-parametric
AI 理解论文
溯源树
样例
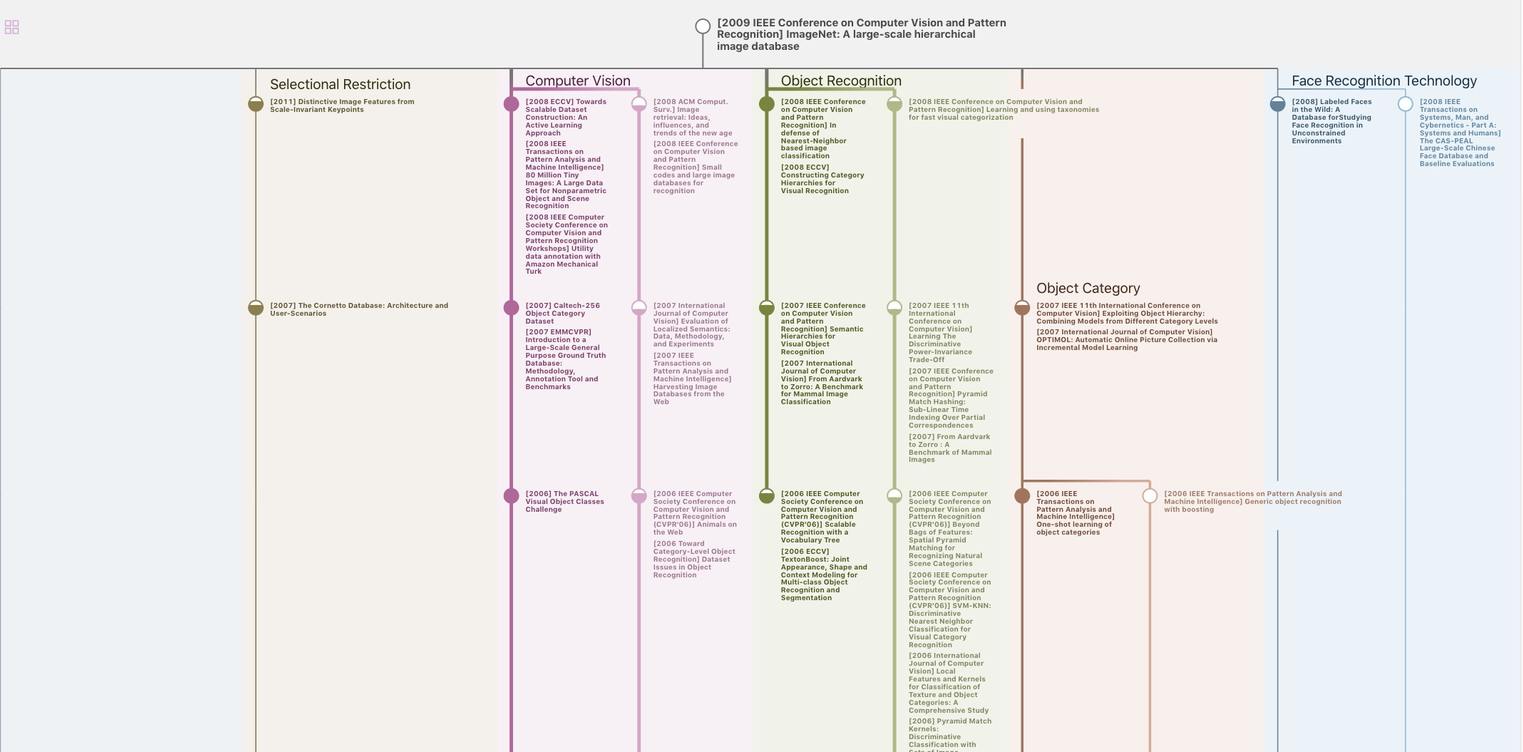
生成溯源树,研究论文发展脉络
Chat Paper
正在生成论文摘要