Evaluation of Parkinsons disease with early diagnosis using single-channel EEG features and auditory cognitive assessment
arXiv (Cornell University)(2023)
Abstract
Parkinsons disease (PD) diagnosis is challenging due to subtle early clinical signs. F-DOPA PET is commonly used for early PD diagnosis. We explore the potential of machine-learning (ML) based EEG features extracted from single-channel EEG during auditory cognitive assessment as a noninvasive, low-cost support for PD diagnosis. The study included data collected from 32 participants who underwent an F-DOPA PET scan as part of their standard treatment and 20 cognitively healthy controls. Participants performed an auditory cognitive assessment recorded with Neurosteer EEG device. Data processing involved wavelet-packet decomposition and ML. First, a prediction model was developed to predict 1/3 of the undisclosed F-DOPA results. Then, generalized linear mixed models were calculated to distinguish between PD and non-PD subjects on the frequency bands and ML-based EEG features (A0 and L1) previously associated with cognitive functions. The prediction model accurately labeled patients with unrevealed scores as positive F-DOPA. Novel EEG feature A0 and the Delta band showed significant separation between study groups, with healthy controls exhibiting higher activity than PD patients. EEG feature L1 activity was significantly lower in resting state compared to high-cognitive load. This effect was absent in the PD group, suggesting that lower activity in resting state is lacking in PD patients. This study successfully demonstrated the ability to separate patients with positive vs. negative F-DOPA PET results with an easy-to-use single-channel EEG during an auditory cognitive assessment. Future longitudinal studies should further explore the potential utility of this tool for early PD diagnosis and as a potential biomarker in PD.
MoreTranslated text
Key words
parkinsons disease,eeg,early diagnosis,assessment,single-channel
AI Read Science
Must-Reading Tree
Example
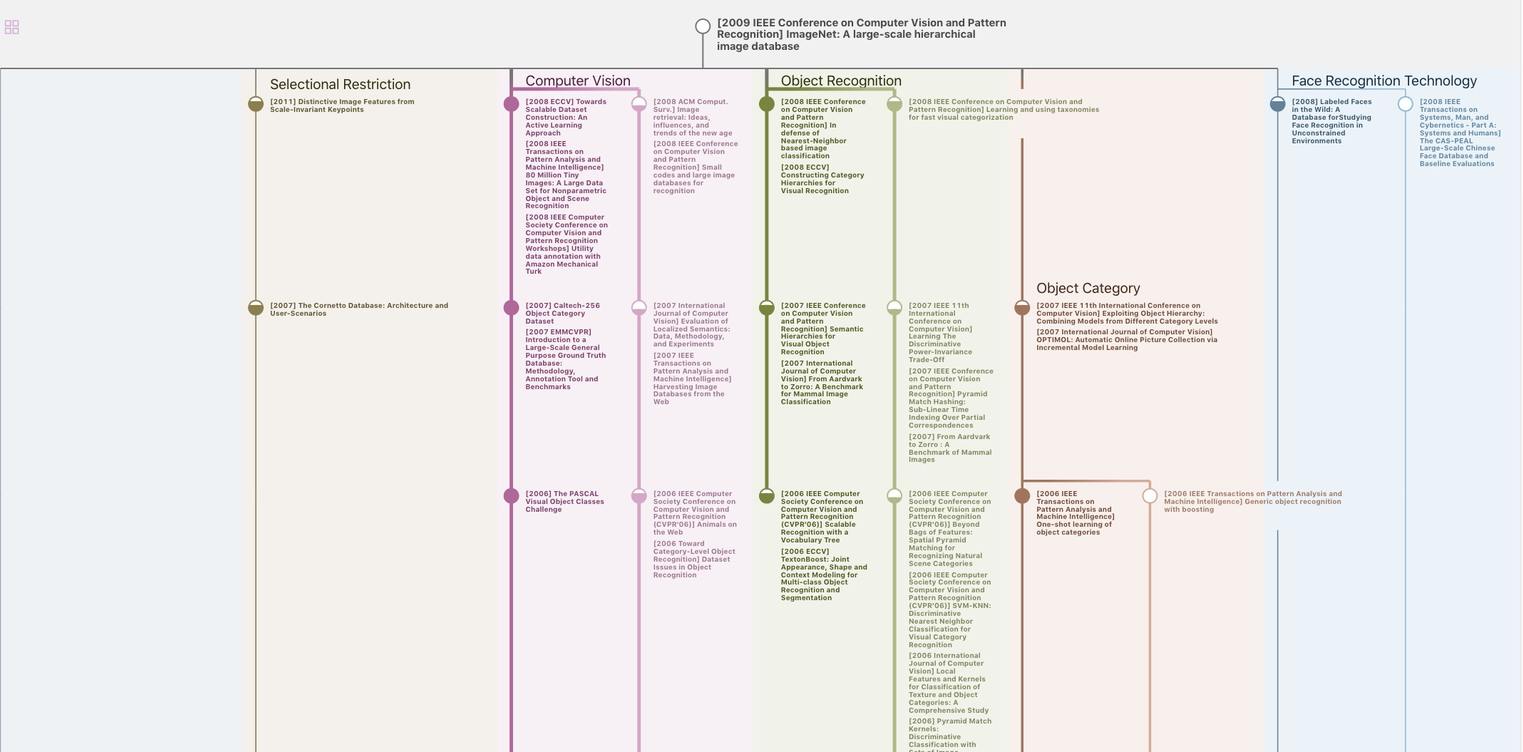
Generate MRT to find the research sequence of this paper
Chat Paper
Summary is being generated by the instructions you defined