POSIT: Promotion of Semantic Item Tail via Adversarial Learning
CoRR(2023)
摘要
In many recommender problems, a handful of popular items (e.g. movies/TV shows, news etc.) can be dominant in recommendations for many users. However, we know that in a large catalog of items, users are likely interested in more than what is popular. The dominance of popular items may mean that users will not see items they would likely enjoy. In this paper, we propose a technique to overcome this problem using adversarial machine learning. We define a metric to translate user-level utility metric in terms of an advantage/disadvantage over items. We subsequently use that metric in an adversarial learning framework to systematically promote disadvantaged items. The resulting algorithm identifies semantically meaningful items that get promoted in the learning algorithm. In the empirical study, we evaluate the proposed technique on three publicly available datasets and four competitive baselines. The result shows that our proposed method not only improves the coverage, but also, surprisingly, improves the overall performance.
更多查看译文
关键词
semantic item tail,promotion,learning
AI 理解论文
溯源树
样例
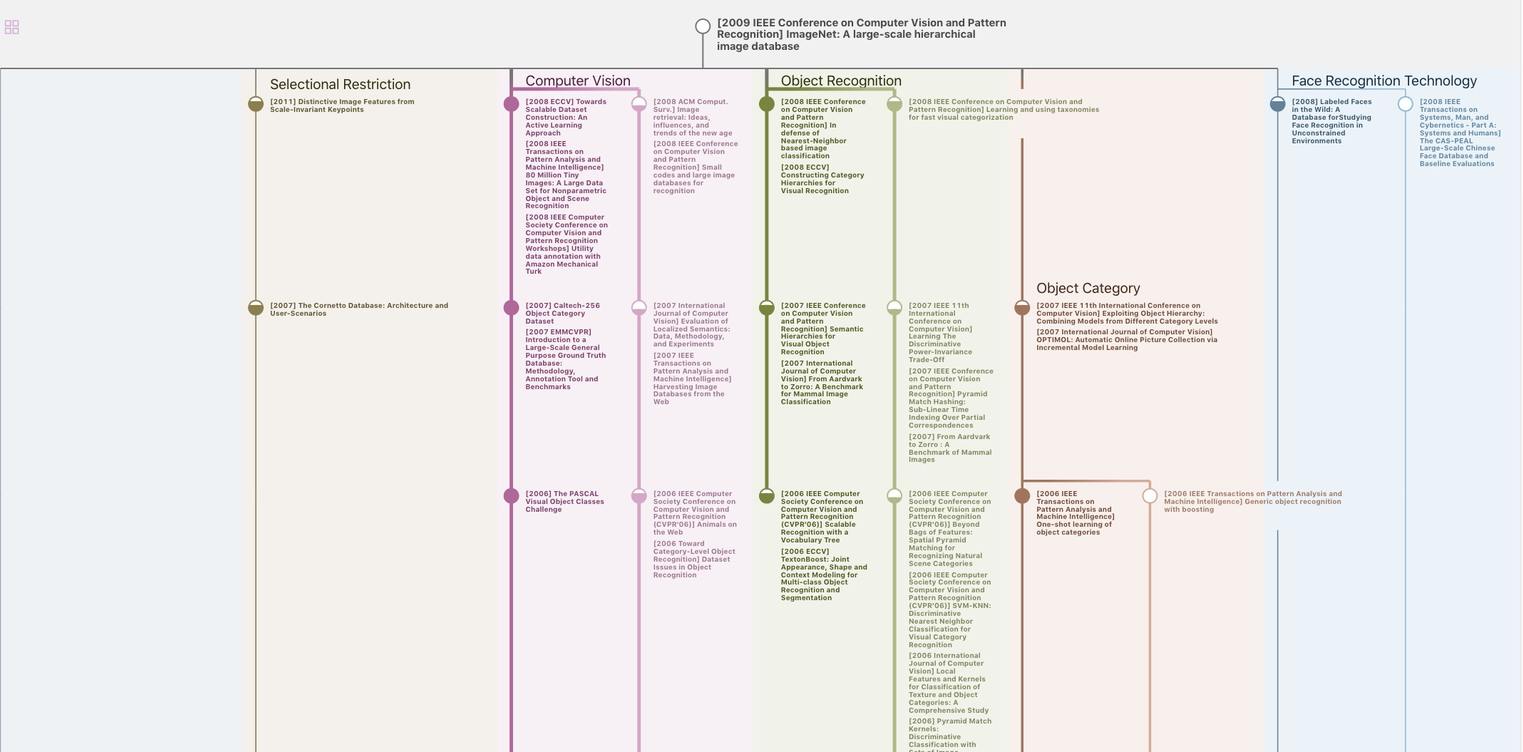
生成溯源树,研究论文发展脉络
Chat Paper
正在生成论文摘要