Gabor-Based Learnable Sparse Representation for Self-Supervised Denoising
IEEE TRANSACTIONS ON GEOSCIENCE AND REMOTE SENSING(2024)
摘要
Traditional supervised denoising networks learn network weights through "black box" (pixel-oriented) training, which requires clean training labels. The inability of such denoising networks to interpret their behavior and the requirement for clean data as labels limit their applicability in real-case scenarios. Deep unfolding methods unroll an optimization process into deep neural networks (DNNs), improving the interpretability of networks. Also, modifiable filters in DNNs allow us to embed the prior information of the desired signals to be extracted, in order to remove noise in a self-supervised manner. Thus, we propose a Gabor-based learnable sparse representation network to suppress different noise types in a self-supervised fashion through constraints/bounds applied to the parameters of the Gabor filters of the network during the training stage. The effectiveness of the proposed method is demonstrated on two noise type examples, pseudo-random noise and ground roll, on synthetic and real data.
更多查看译文
关键词
Deep learning,seismic denoising,self-supervised
AI 理解论文
溯源树
样例
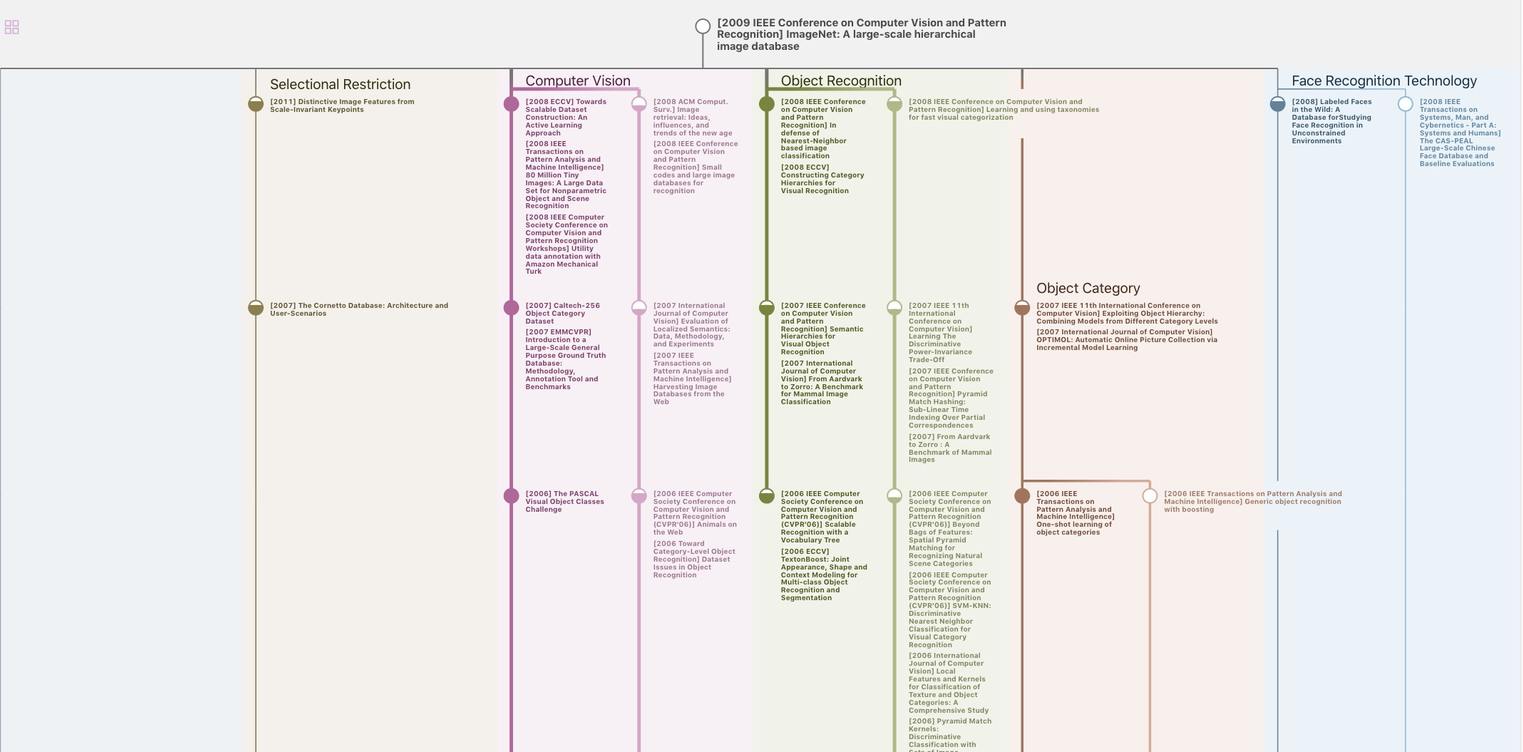
生成溯源树,研究论文发展脉络
Chat Paper
正在生成论文摘要