TOPIQ: A Top-Down Approach From Semantics to Distortions for Image Quality Assessment
IEEE TRANSACTIONS ON IMAGE PROCESSING(2024)
摘要
Image Quality Assessment (IQA) is a fundamental task in computer vision that has witnessed remarkable progress with deep neural networks. Inspired by the characteristics of the human visual system, existing methods typically use a combination of global and local representations (i.e., multi-scale features) to achieve superior performance. However, most of them adopt simple linear fusion of multi-scale features, and neglect their possibly complex relationship and interaction. In contrast, humans typically first form a global impression to locate important regions and then focus on local details in those regions. We therefore propose a top-down approach that uses high-level semantics to guide the IQA network to focus on semantically important local distortion regions, named as TOPIQ. Our approach to IQA involves the design of a heuristic coarse-to-fine network (CFANet) that leverages multi-scale features and progressively propagates multi-level semantic information to low-level representations in a top-down manner. A key component of our approach is the proposed cross-scale attention mechanism, which calculates attention maps for lower level features guided by higher level features. This mechanism emphasizes active semantic regions for low-level distortions, thereby improving performance. TOPIQ can be used for both Full-Reference (FR) and No-Reference (NR) IQA. We use ResNet50 as its backbone and demonstrate that TOPIQ achieves better or competitive performance on most public FR and NR benchmarks compared with state-of-the-art methods based on vision transformers, while being much more efficient (with only similar to 13% FLOPS of the current best FR method). Codes are released at https://github.com/chaofengc/IQA-PyTorch.
更多查看译文
关键词
Image quality assessment,top-down approach,multi-scale features,cross-scale attention
AI 理解论文
溯源树
样例
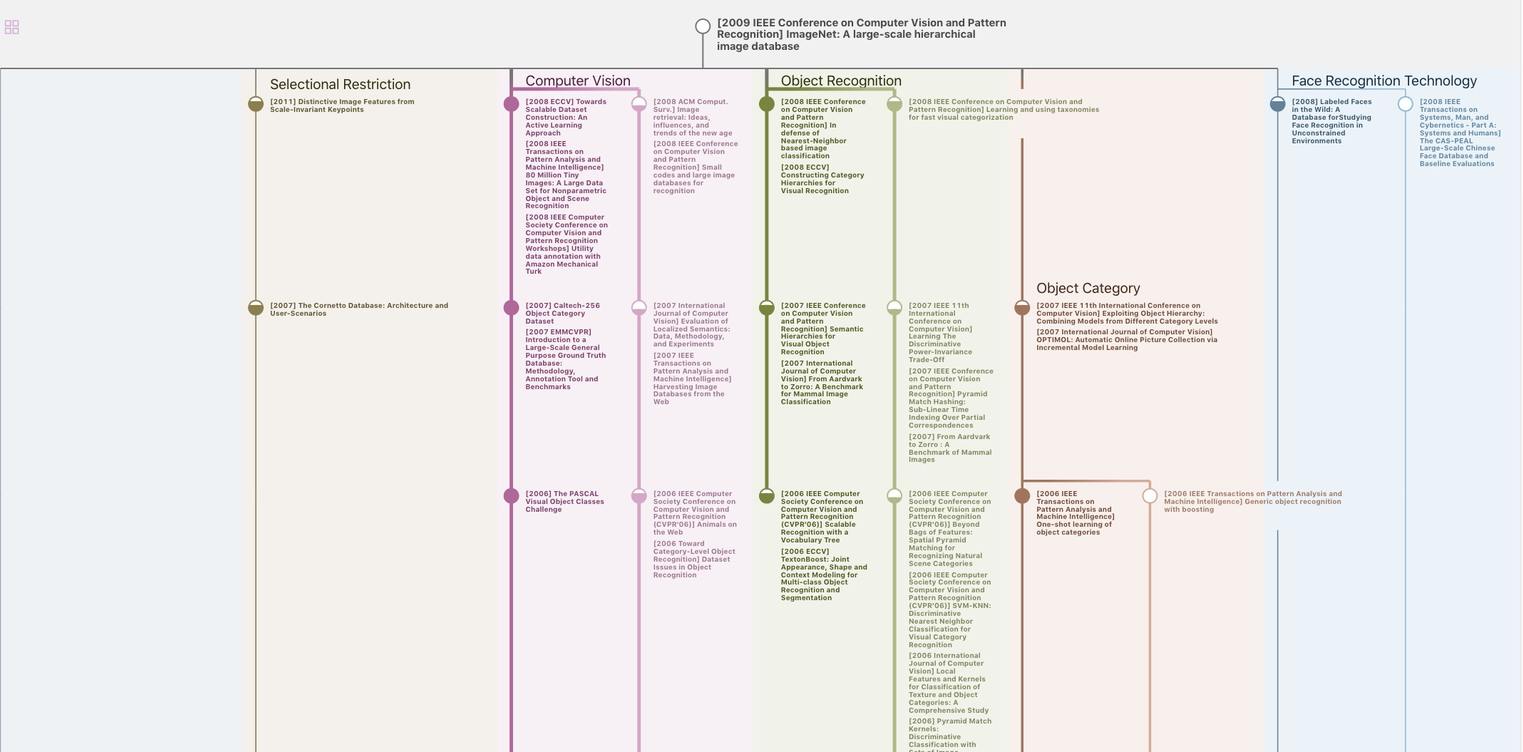
生成溯源树,研究论文发展脉络
Chat Paper
正在生成论文摘要