MomentaMorph: Unsupervised Spatial-Temporal Registration with Momenta, Shooting, and Correction
CoRR(2023)
摘要
Tagged magnetic resonance imaging (tMRI) has been employed for decades to measure the motion of tissue undergoing deformation. However, registration-based motion estimation from tMRI is difficult due to the periodic patterns in these images, particularly when the motion is large. With a larger motion the registration approach gets trapped in a local optima, leading to motion estimation errors. We introduce a novel "momenta, shooting, and correction" framework for Lagrangian motion estimation in the presence of repetitive patterns and large motion. This framework, grounded in Lie algebra and Lie group principles, accumulates momenta in the tangent vector space and employs exponential mapping in the diffeomorphic space for rapid approximation towards true optima, circumventing local optima. A subsequent correction step ensures convergence to true optima. The results on a 2D synthetic dataset and a real 3D tMRI dataset demonstrate our method's efficiency in estimating accurate, dense, and diffeomorphic 2D/3D motion fields amidst large motion and repetitive patterns.
更多查看译文
关键词
momentamorph,registration,spatial-temporal
AI 理解论文
溯源树
样例
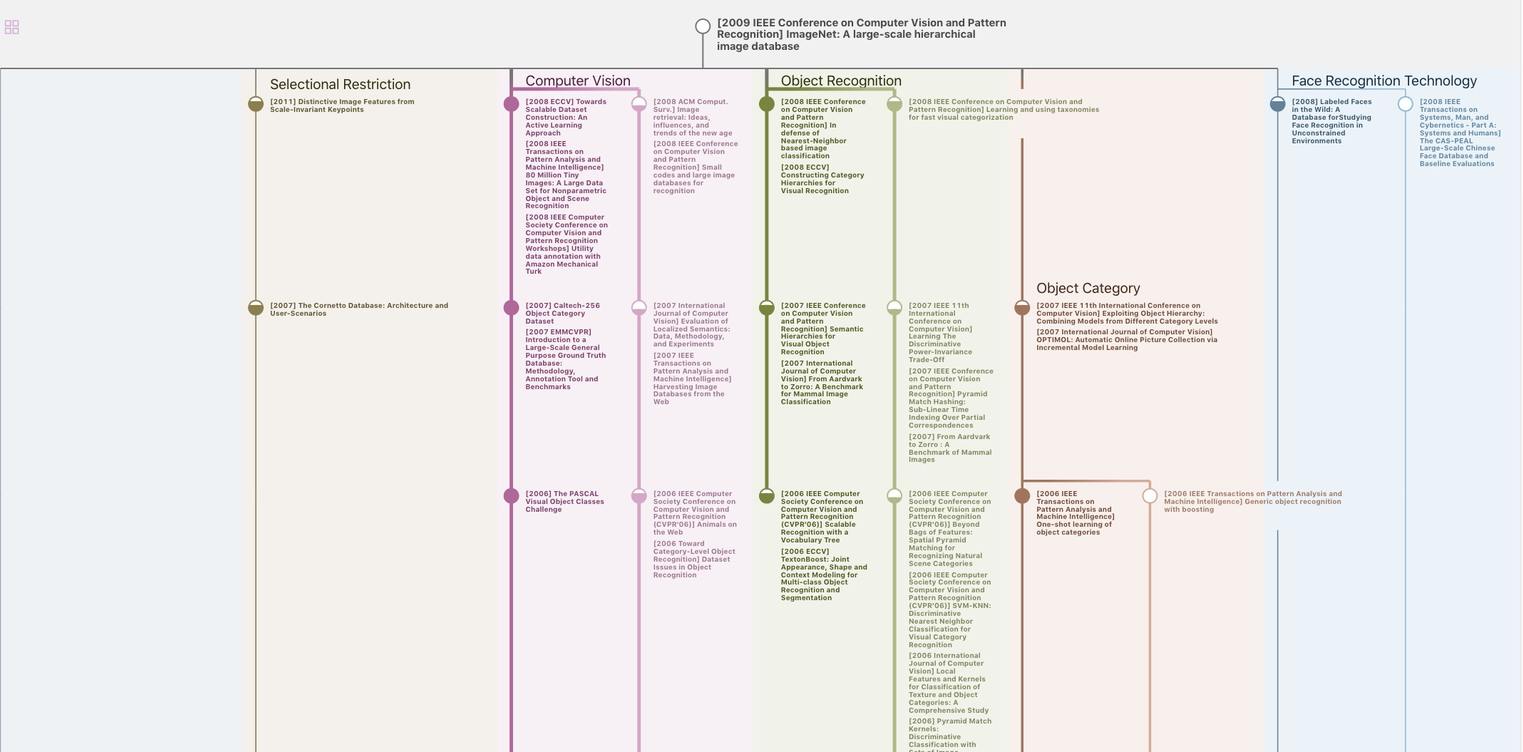
生成溯源树,研究论文发展脉络
Chat Paper
正在生成论文摘要