Bias Behind the Wheel: Fairness Analysis of Autonomous Driving Systems
arxiv(2023)
摘要
This paper analyzes fairness in automated pedestrian detection, a crucial but
under-explored issue in autonomous driving systems. We evaluate eight
state-of-the-art deep learning-based pedestrian detectors across demographic
groups on large-scale real-world datasets. To enable thorough fairness testing,
we provide extensive annotations for the datasets, resulting in 8,311 images
with 16,070 gender labels, 20,115 age labels, and 3,513 skin tone labels. Our
findings reveal significant fairness issues, particularly related to age. The
undetected proportions for children are 20.14
Furthermore, we explore how various driving scenarios affect the fairness of
pedestrian detectors. We find that pedestrian detectors demonstrate significant
gender biases during night time, potentially exacerbating the prevalent
societal issue of female safety concerns during nighttime out. Moreover, we
observe that pedestrian detectors can demonstrate both enhanced fairness and
superior performance under specific driving conditions, which challenges the
fairness-performance trade-off theory widely acknowledged in the fairness
literature. We publicly release the code, data, and results to support future
research on fairness in autonomous driving.
更多查看译文
AI 理解论文
溯源树
样例
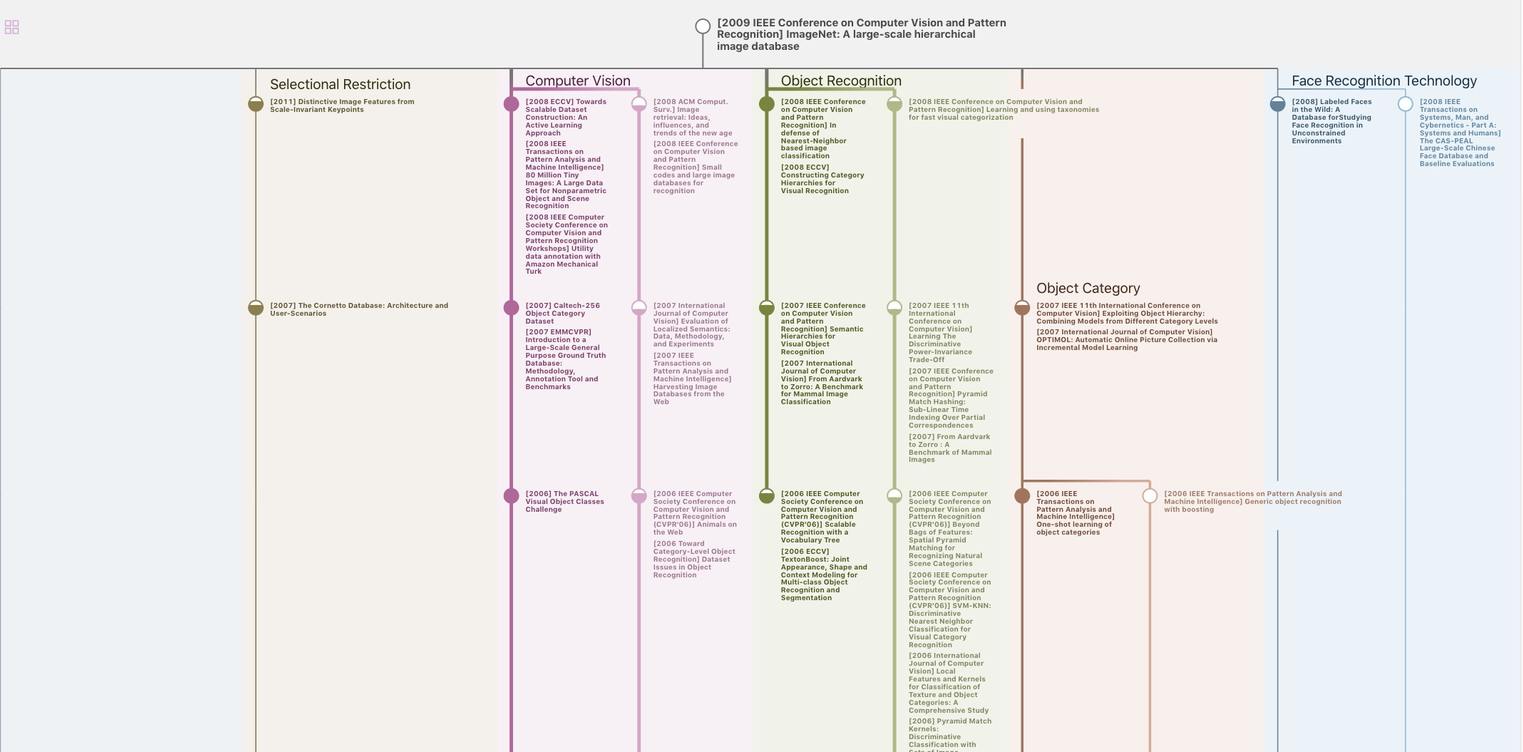
生成溯源树,研究论文发展脉络
Chat Paper
正在生成论文摘要