Fast Bayesian High-Dimensional Gaussian Graphical Model Estimation
arxiv(2023)
Abstract
Graphical models describe associations between variables through the notion of conditional independence. Gaussian graphical models are a widely used class of such models where the relationships are formalized by non-null entries of the precision matrix. However, in high dimensional cases, standard covariance estimates are typically unstable. Moreover, it is natural to expect only a few significant associations to be present in many realistic applications. This necessitates the injection of sparsity techniques into the estimation. Classical frequentist methods use penalization for this purpose; in contrast, fully Bayesian methods are computationally slow, typically requiring iterative sampling over a quadratic number of parameters in a space constrained by positive definiteness. We propose a Bayesian graph estimation method based on an ensemble of Bayesian neighborhood regressions. An attractive feature of our methods is the ability for easy parallelization across separate graphical neighborhoods, invoking computational efficiency greater than most existing methods. Our strategy induces sparsity with a Horseshoe shrinkage prior and includes a novel variable selection step based on the marginal likelihood from the predictors ranks. Our method appropriately combines the estimated regression coefficients to produce a graph estimate and a matrix of partial correlation estimates for inference. Performance of various methods are assessed using measures like FDR and TPR. Competitive performance across a variety of cases is demonstrated through extensive simulations. Lastly, we apply these methods to investigate the dependence structure across genetic expressions for women with triple negative breast cancer.
MoreTranslated text
AI Read Science
Must-Reading Tree
Example
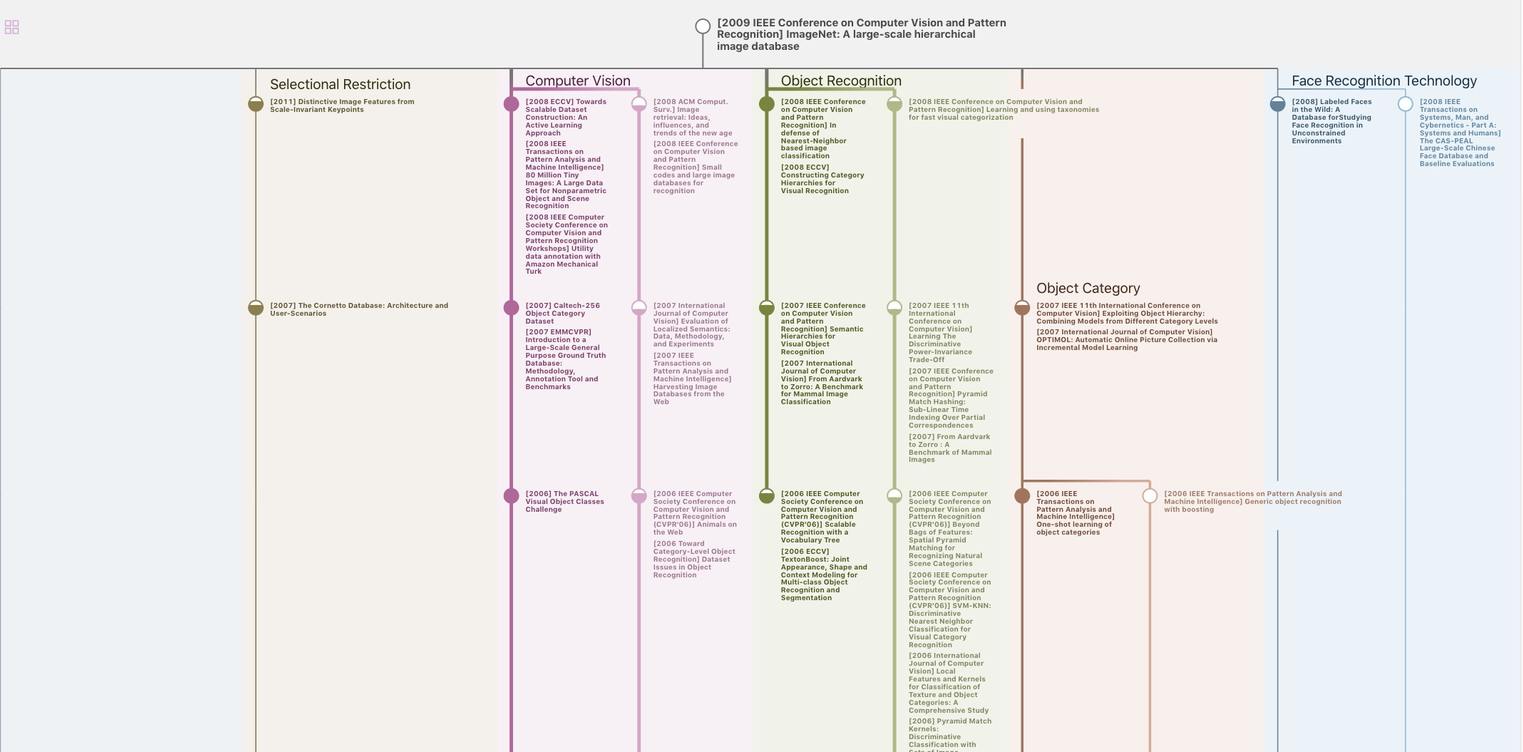
Generate MRT to find the research sequence of this paper
Chat Paper
Summary is being generated by the instructions you defined