SMARLA: A Safety Monitoring Approach for Deep Reinforcement Learning Agents
CoRR(2023)
摘要
Deep reinforcement learning algorithms (DRL) are increasingly being used in
safety-critical systems. Ensuring the safety of DRL agents is a critical
concern in such contexts. However, relying solely on testing is not sufficient
to ensure safety as it does not offer guarantees. Building safety monitors is
one solution to alleviate this challenge. This paper proposes SMARLA, a machine
learning-based safety monitoring approach designed for DRL agents. For
practical reasons, SMARLA is designed to be black-box (as it does not require
access to the internals or training data of the agent) and leverages state
abstraction to reduce the state space and thus facilitate the learning of
safety violation prediction models from agent's states. We validated SMARLA on
two well-known RL case studies. Empirical analysis reveals that SMARLA achieves
accurate violation prediction with a low false positive rate, and can predict
safety violations at an early stage, approximately halfway through the agent's
execution before violations occur.
更多查看译文
关键词
deep reinforcement learning
AI 理解论文
溯源树
样例
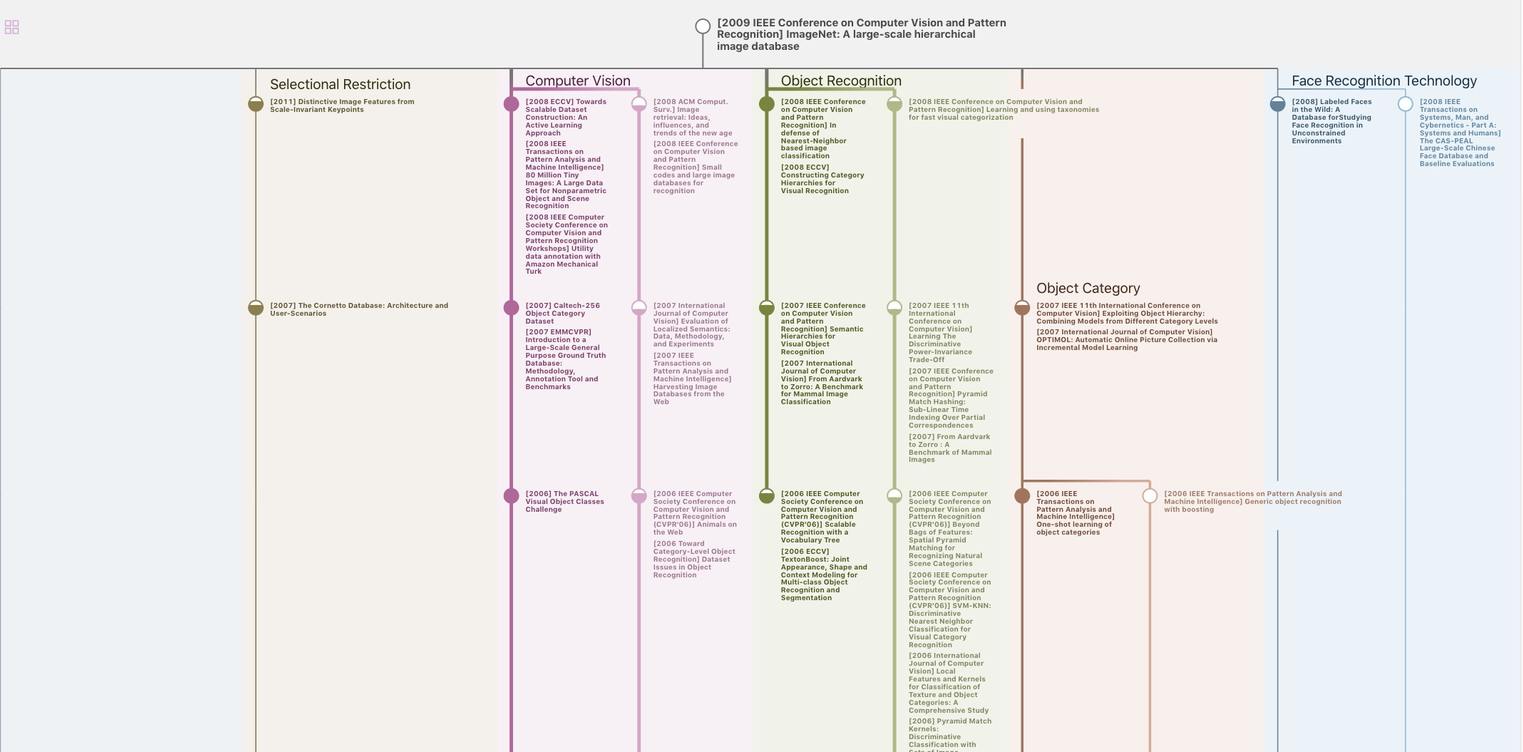
生成溯源树,研究论文发展脉络
Chat Paper
正在生成论文摘要