PARL: A Unified Framework for Policy Alignment in Reinforcement Learning from Human Feedback
arxiv(2023)
摘要
We present a novel unified bilevel optimization-based framework,
, formulated to address the recently highlighted critical issue of
policy alignment in reinforcement learning using utility or preference-based
feedback. We identify a major gap within current algorithmic designs for
solving policy alignment due to a lack of precise characterization of the
dependence of the alignment objective on the data generated by policy
trajectories. This shortfall contributes to the sub-optimal performance
observed in contemporary algorithms. Our framework addressed these concerns by
explicitly parameterizing the distribution of the upper alignment objective
(reward design) by the lower optimal variable (optimal policy for the designed
reward). Interestingly, from an optimization perspective, our formulation leads
to a new class of stochastic bilevel problems where the stochasticity at the
upper objective depends upon the lower-level variable. True to our best
knowledge, this work presents the first formulation of the RLHF as a bilevel
optimization problem which generalizes the existing RLHF formulations and
addresses the existing distribution shift issues in RLHF formulations. To
demonstrate the efficacy of our formulation in resolving alignment issues in
RL, we devised an algorithm named to solve PARL problem,
establishing sample complexity bounds of order 𝒪(1/T). Our
empirical results substantiate that the proposed can address the
alignment concerns in RL by showing significant improvements (up to 63% in
terms of required samples) for policy alignment in large-scale environments of
the Deepmind control suite and Meta world tasks.
更多查看译文
AI 理解论文
溯源树
样例
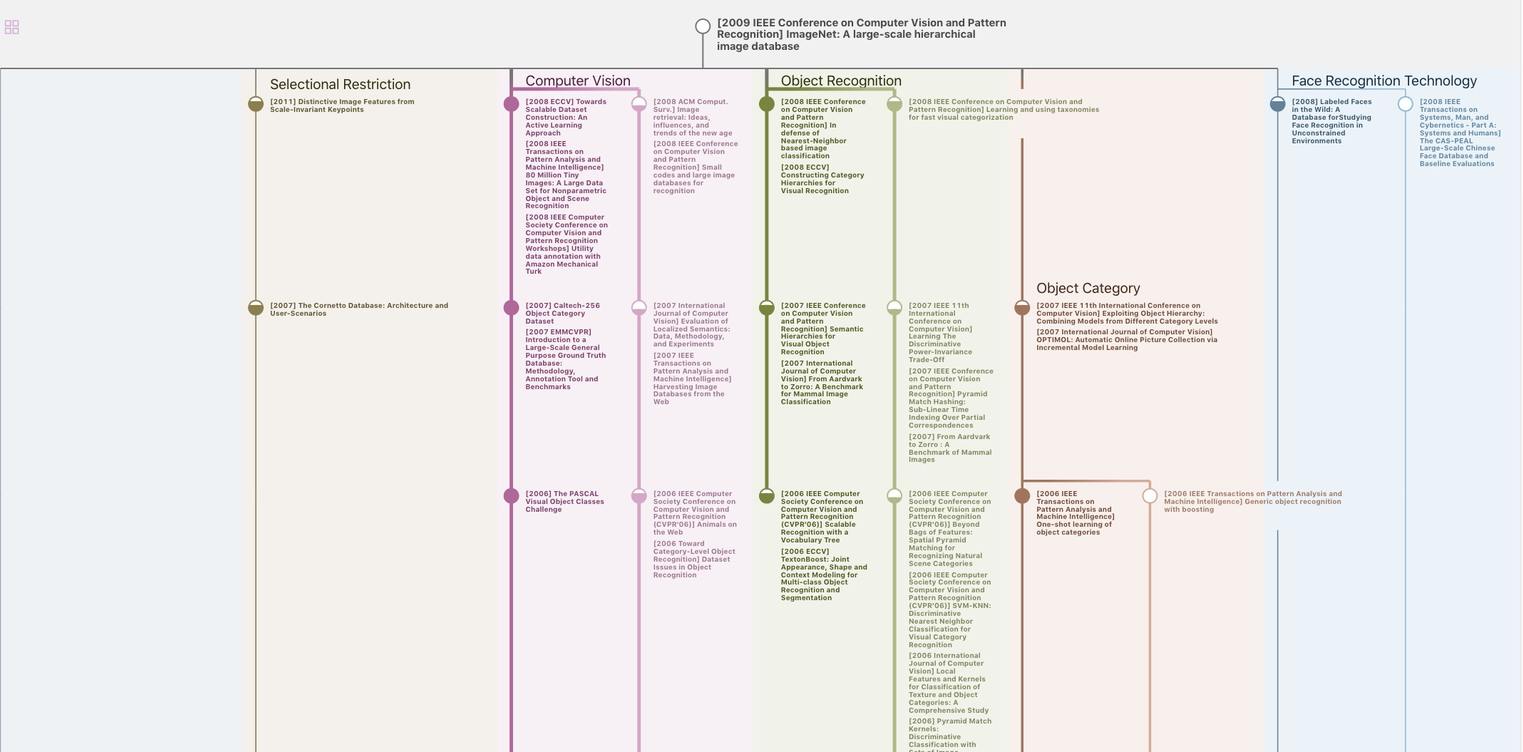
生成溯源树,研究论文发展脉络
Chat Paper
正在生成论文摘要