Pyramid style-attentional network for arbitrary style transfer
Multimedia Tools and Applications(2024)
摘要
present, the self-attention mechanism represented by the non-local network has been applied in style transfer widely. Models can achieve good style transfer effects by considering long-range dependencies between content images and style images while well maintaining semantic content information. However, the self-attention mechanism has to calculate the relationship between all positions between the content feature maps and style feature maps. The associated computational complexity of the mechanism is rather high, which will consume a lot of computing resources and adversely impact the efficiency of style transfer of high-resolution images. To solve this problem, we propose a novel Pyramid Style-attentional Network (PSANet) to reduce the computational complexity of the self-attention network by using pyramid pooling on feature maps. We compare our method with the vanilla style-attentional network in terms of speed and quality. The experimental results show that our model can significantly reduce the computational complexity and achieve good transfer effects. Especially for handling high-resolution images, the execution time of our method can reduce by 34.7% .
更多查看译文
关键词
Style transfer,Pyramid pooling,Self-attention,Image processing
AI 理解论文
溯源树
样例
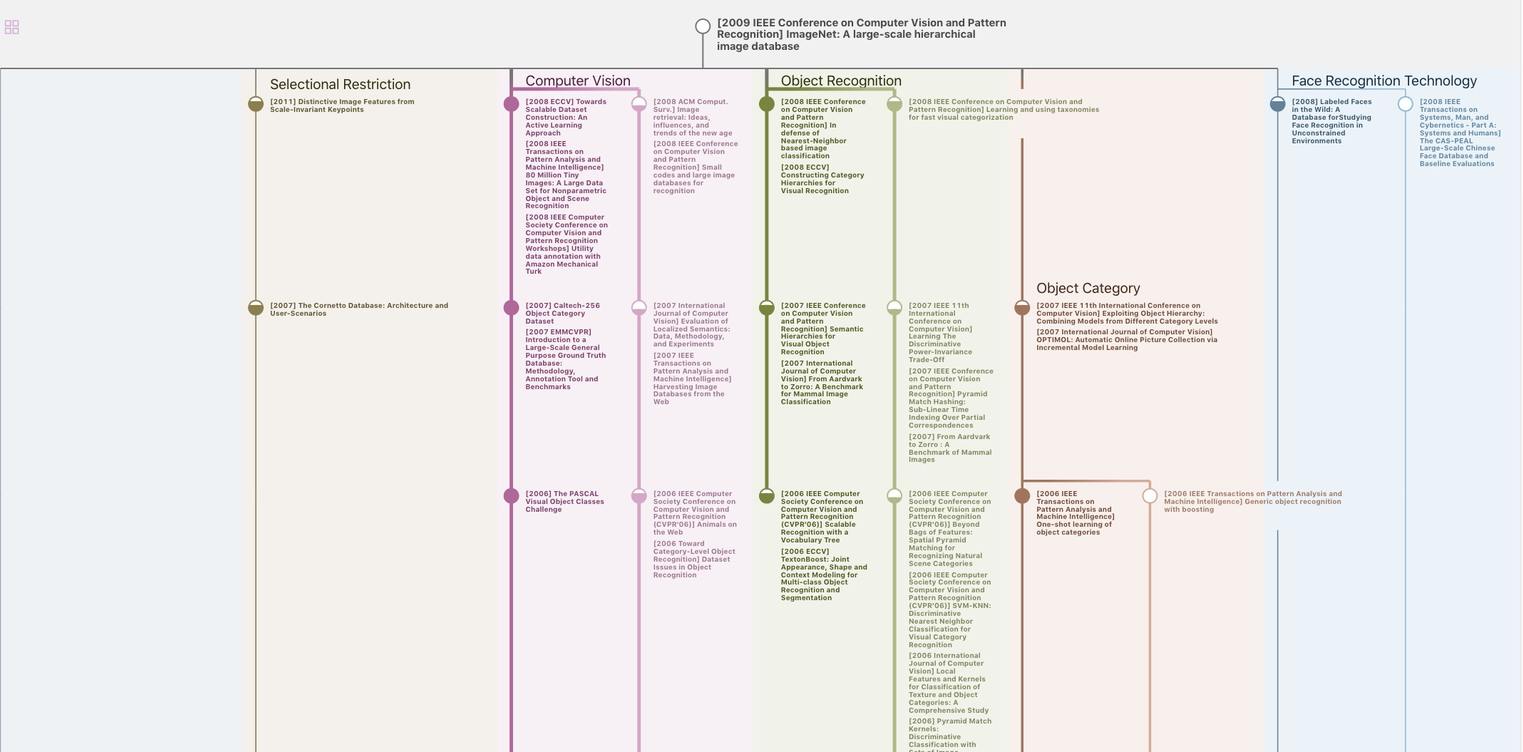
生成溯源树,研究论文发展脉络
Chat Paper
正在生成论文摘要