Resnet-based deep learning multilayer fault detection model-based fault diagnosis
Multimedia Tools and Applications(2024)
摘要
Fault detection has taken on critical relevance in today’s automated manufacturing processes. Defect tolerance, dependability, and safety are some of the fundamental design attributes of complex engineering systems provided by this method. Fault Diagnosis is made more difficult by a lack of performance; data-driven design and the capacity to transfer learning are also essential considerations. This paper proposes the ResNet-based deep learning multilayer fault detection model (ResNet-DLMFDM) to enrich high performance, design, and transmission-learning skills. Wavelet pyramid packet decomposition and each sub drive coefficient utilize the input of each deep research network channel for multi-kernel domain analysis. Pseudo-label networks have been developed conceptually to investigate different interval lengths of sequential functionality and to gather local database flow sequence functions to improve existing error detection processes. Experiment findings reveal that the proposed approach outperforms current algorithms regarding data correctness, storage space utilization, computational complexity, noiselessness, and transfer performance. The results are obtained by analyzing the multi-kernel and showing the domain ratio of 87.6%, increased storage space ratio of 88.6%, wavelet decomposition performance ratio of 84.5%, and the high accuracy of the data transmission ratio of 83.5%, and the noiseless diagnosis ratio of 93.8%.
更多查看译文
关键词
ResNet, Fault diagnosis, Automation,Multilayer,Deep learning,Fault detection model,SVM
AI 理解论文
溯源树
样例
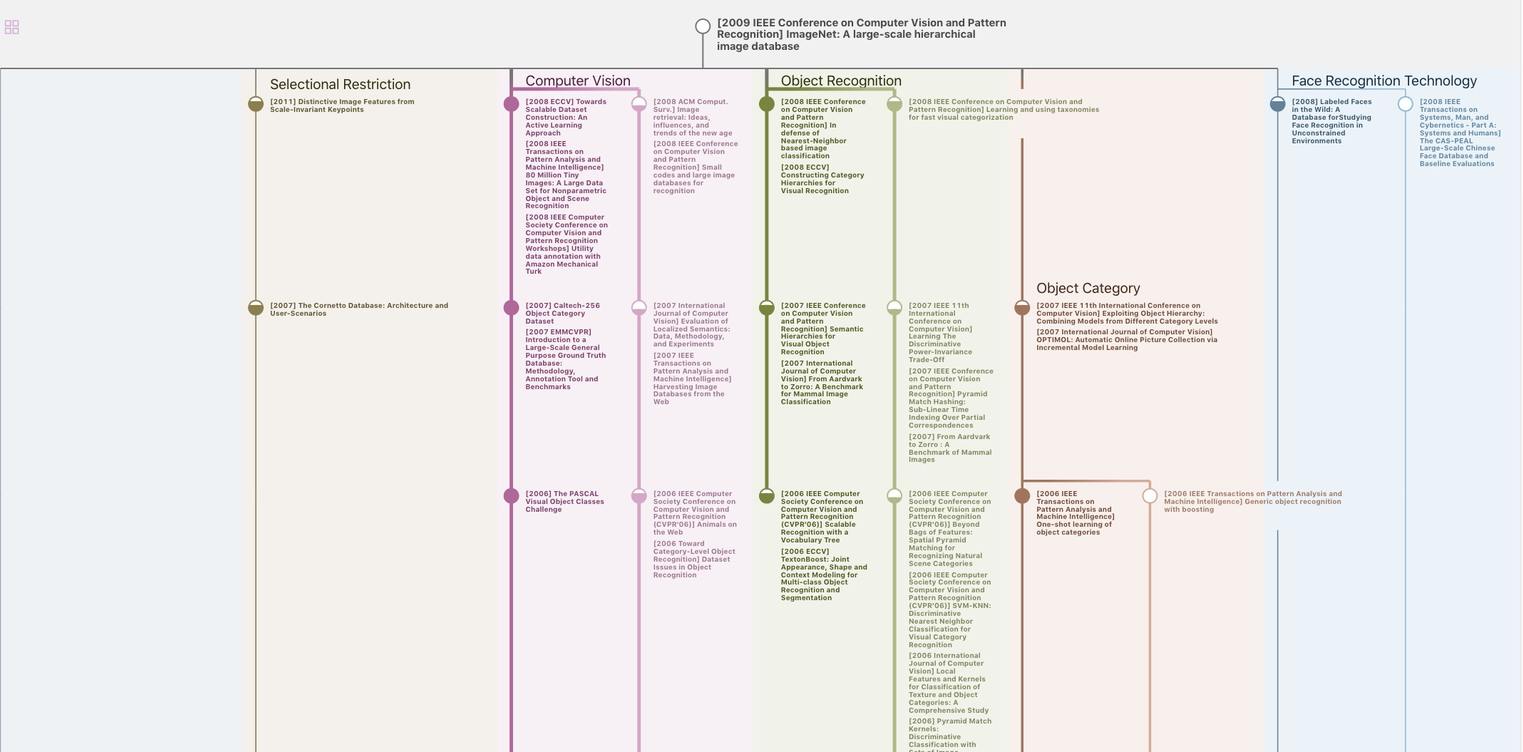
生成溯源树,研究论文发展脉络
Chat Paper
正在生成论文摘要