Suicide risk assessment using word-level model with dictionary-based risky posts selection
Multimedia Tools and Applications(2024)
摘要
Suicide is a serious issue around the world and is a leading cause of death in US. In the past 20 years, the suicide rate has seen a significant increase of 35%. With the rapid development of information technology, more and more people begin to use social media to share their inner feelings. It enables social media data to be widely used for research on suicide risk assessment. However, not all social media posts are suicide related. Previous research addressed this problem with post-level attention mechanism. However, post-level attention mechanism may not find relevant suicide posts. This problem becomes more serious in the feature-based post embeddings since each post is converted into a single vector to serve as the input of the model, resulting in the loss of word-level information during training. In this paper, we addressed this problem by introducing a novel word-level model including a post-selectin layer as a solution. Firstly, we utilize a suicide keyword dictionary to identify risky posts that may be missed by the post-level attention mechanism. We then convert the words in the risky posts into word embeddings and use self-attention to generate the post embeddings for the risky posts. Finally, we pass the post embeddings to a multilayer perceptron to classify the suicide risk. We also demonstrate that the FScore used in previous studies can be reduced to a function of accuracy, which does not reflect the model performance in predicting imbalanced datasets. Therefore, we additionally adopt macro F1 score as the evaluation function. Experiment results show that our model not only outperforms previous studies in FScore performance, but also achieves macro F1 Score a nearly 4% improvement compared to previous studies.
更多查看译文
关键词
Social media,Deep learning,Natural language processing,Suicide ideation,Reddit
AI 理解论文
溯源树
样例
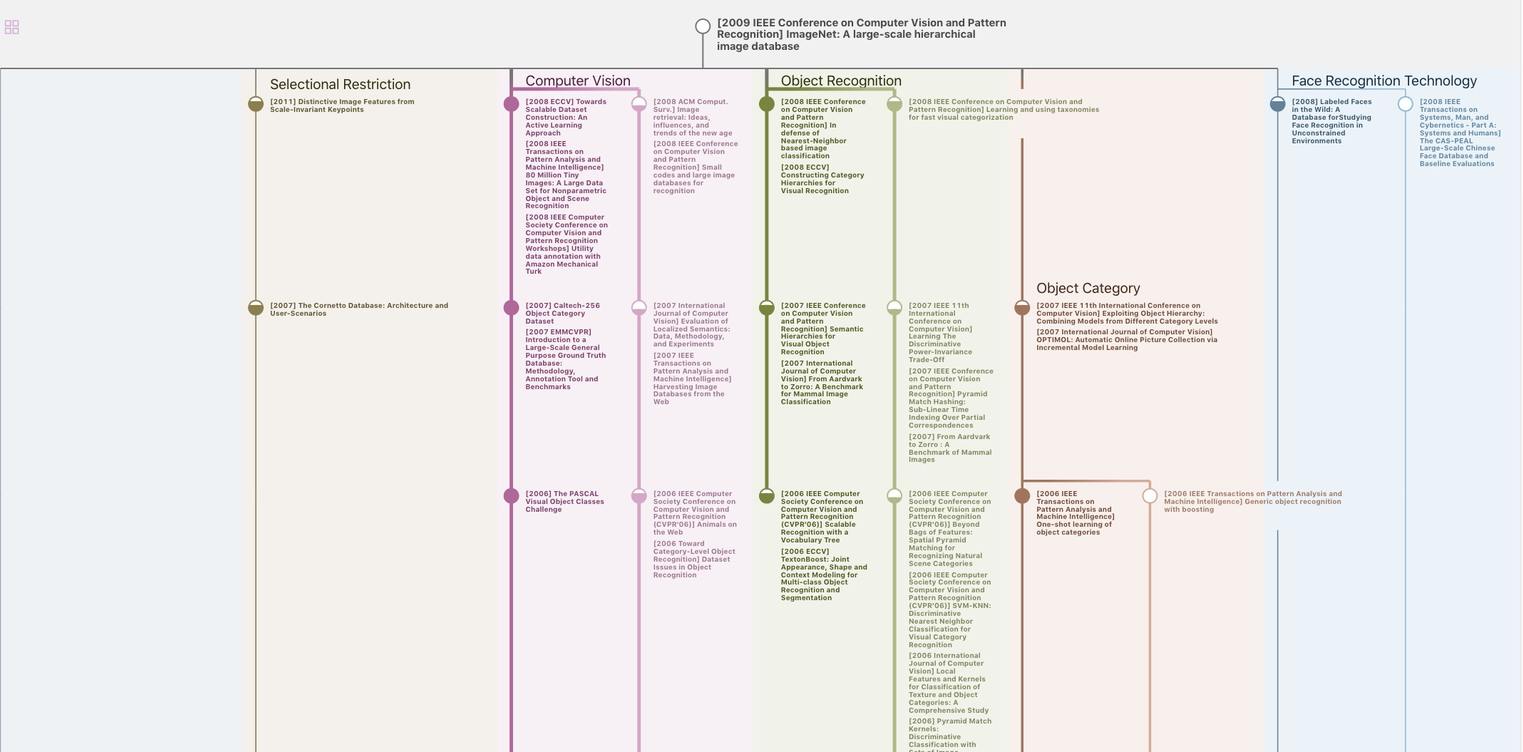
生成溯源树,研究论文发展脉络
Chat Paper
正在生成论文摘要