Central limit theorem for the stratified resampling mechanism
arXiv (Cornell University)(2023)
摘要
The stratified resampling mechanism is one of the resampling schemes commonly used in the resampling steps of particle filters. In the present paper, we prove a central limit theorem for this mechanism under the assumption that the initial positions are independent and identically distributed and the weights proportional to a positive function of the positions such that the image of their common distribution by this function has a non zero component absolutely continuous with respect to the Lebesgue measure. This result relies on the convergence in distribution of the fractional part of partial sums of the normalized weights to some random variable uniformly distributed on $[0,1]$, which is established in the companion paper \cite{CLTfract} by overcoming the difficulty raised by the coupling through the normalization. Under the conjecture that a similar convergence in distribution remains valid at the next steps of a particle filter which alternates selections according to the stratified resampling mechanism and mutations according to Markov kernels, we provide an inductive formula for the asymptotic variance of the resampled population after $n$ steps. We perform numerical experiments which support the validity of this formula.
更多查看译文
关键词
stratified resampling mechanism,central limit theorem
AI 理解论文
溯源树
样例
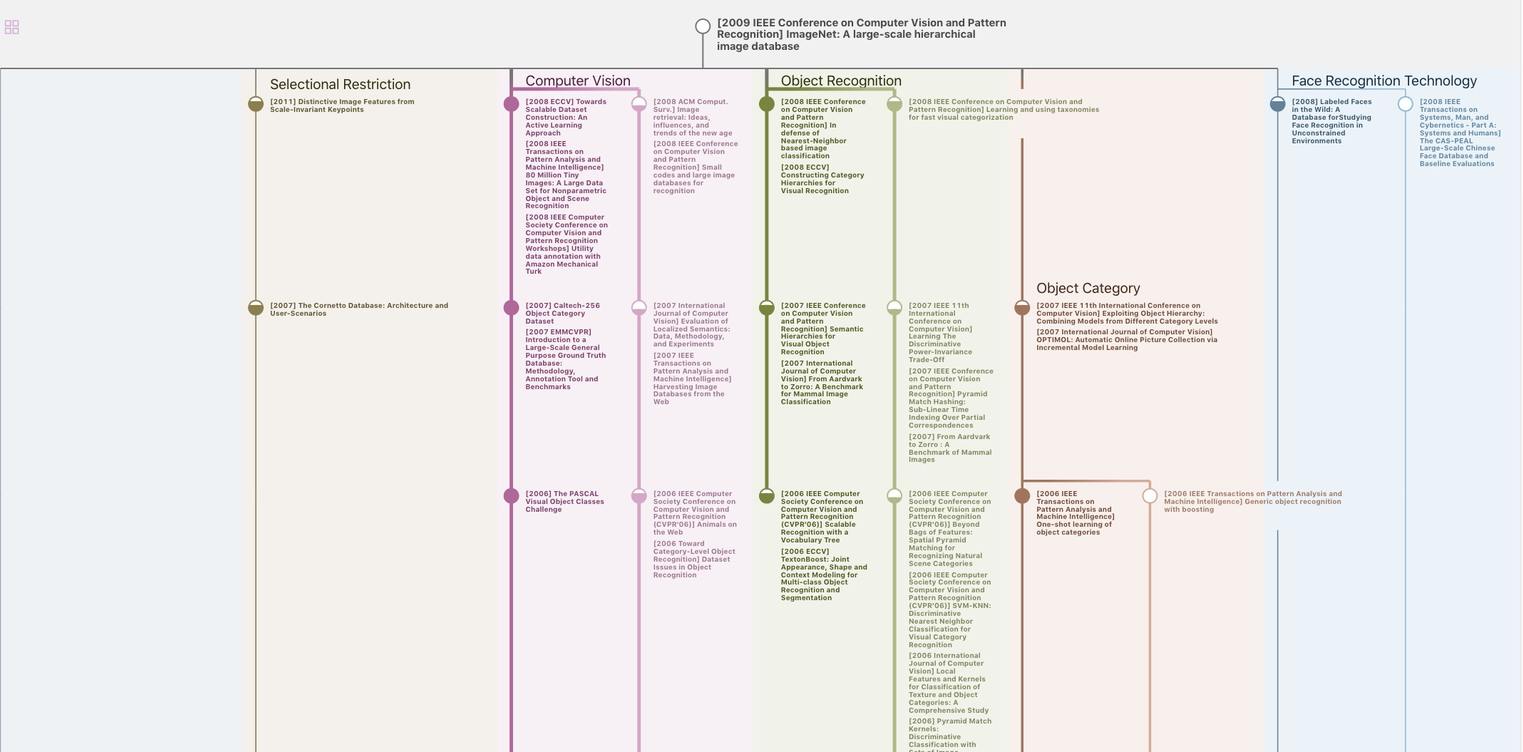
生成溯源树,研究论文发展脉络
Chat Paper
正在生成论文摘要